Re-evaluating Evaluation in Text Summarization
Conference on Empirical Methods in Natural Language Processing(2020)
摘要
Automated evaluation metrics as a stand-in for manual evaluation are an essential part of the development of text-generation tasks such as text summarization. However, while the field has progressed, our standard metrics have not – for nearly 20 years ROUGE has been the standard evaluation in most summarization papers. In this paper, we make an attempt to re-evaluate the evaluation method for text summarization: assessing the reliability of automatic metrics using top-scoring system outputs, both abstractive and extractive, on recently popular datasets for both system-level and summary-level evaluation settings. We find that conclusions about evaluation metrics on older datasets do not necessarily hold on modern datasets and systems. We release a dataset of human judgments that are collected from 25 top-scoring neural summarization systems (14 abstractive and 11 extractive).
更多查看译文
关键词
evaluation,text,re-evaluating
AI 理解论文
溯源树
样例
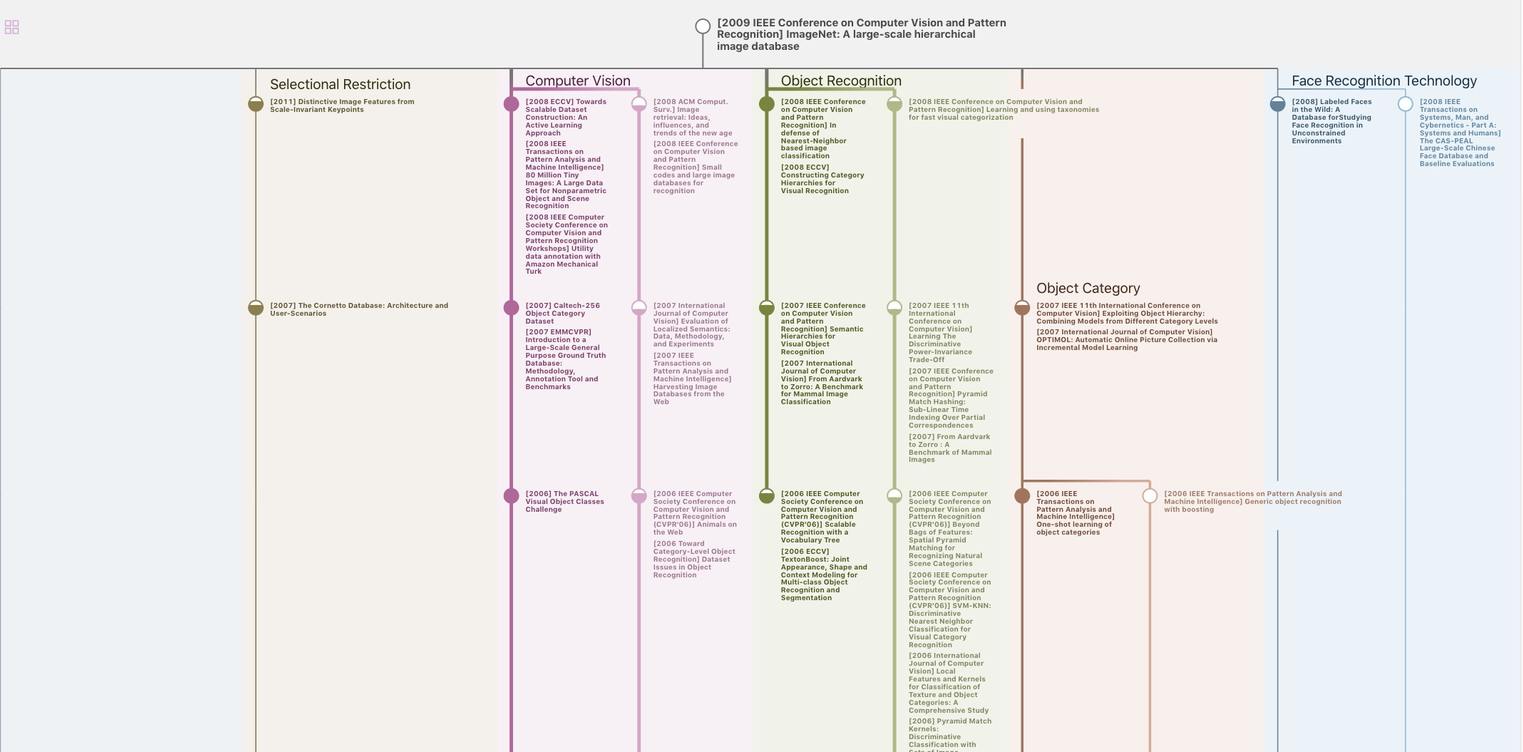
生成溯源树,研究论文发展脉络
Chat Paper
正在生成论文摘要