VoiceGrad: Non-Parallel Any-to-Many Voice Conversion With Annealed Langevin Dynamics
IEEE-ACM TRANSACTIONS ON AUDIO SPEECH AND LANGUAGE PROCESSING(2024)
摘要
In this paper, we propose a non-parallel any-to-many voice conversion (VC) method termed VoiceGrad. Inspired by WaveGrad, a recently introduced novel waveform generation method, VoiceGrad is based upon the concepts of score matching, Langevin dynamics, and diffusion models. The idea involves training a score approximator, a fully convolutional network with a U-Net structure, to predict the gradient of the log density of the speech feature sequences of multiple speakers. The trained score approximator can be used to perform VC by using annealed Langevin dynamics or reverse diffusion process to iteratively update an input feature sequence towards the nearest stationary point of the target distribution. Thanks to the nature of this concept, VoiceGrad enables any-to-many VC, a VC scenario in which the speaker of input speech can be arbitrary, and allows for non-parallel training, which requires no parallel utterances.
更多查看译文
关键词
Decoding,Training,Data models,Generators,Diffusion processes,Jacobian matrices,Generative adversarial networks,Voice conversion (VC),non-parallel VC,any-to-many VC,score matching,Langevin dynamics,diffusion models
AI 理解论文
溯源树
样例
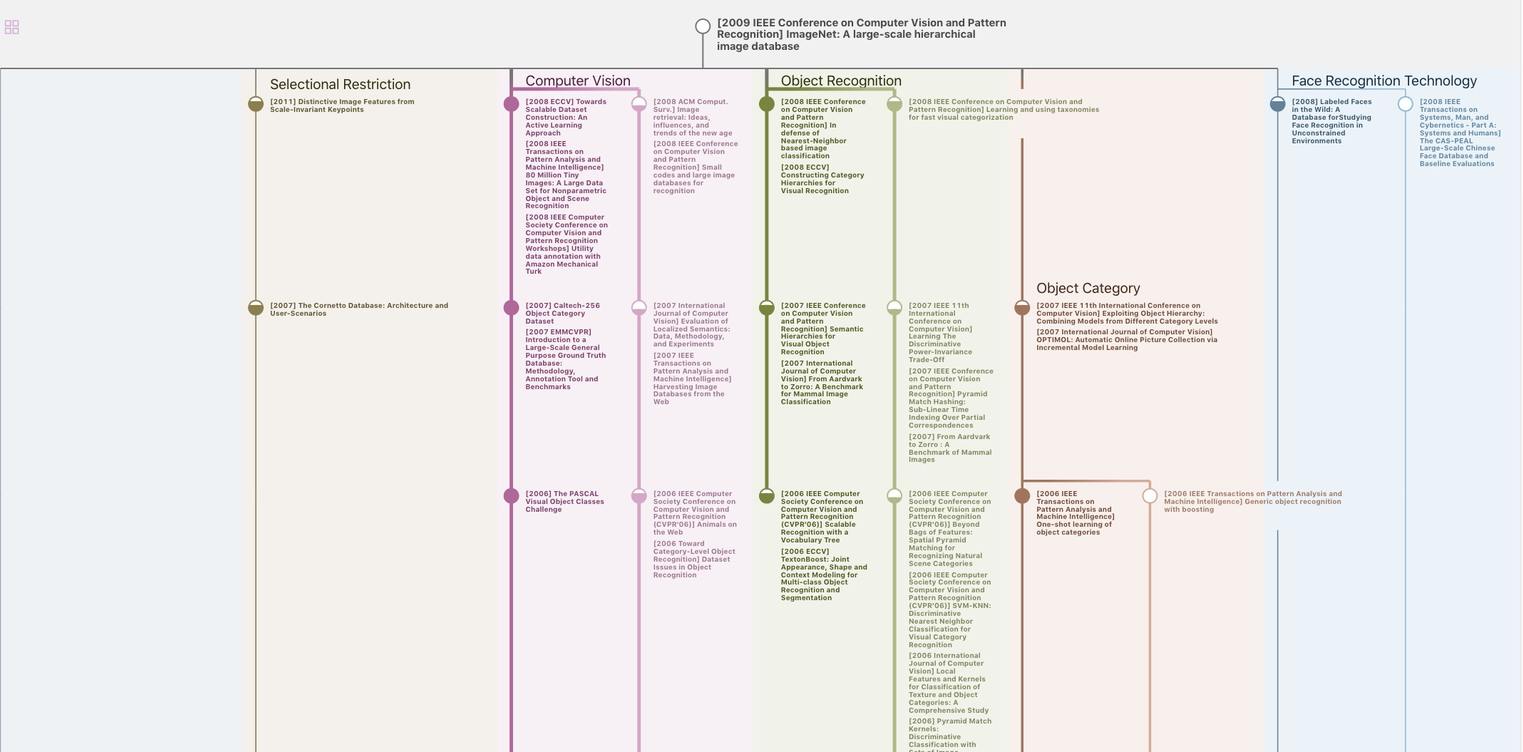
生成溯源树,研究论文发展脉络
Chat Paper
正在生成论文摘要