Lifelong Language Knowledge Distillation
Conference on Empirical Methods in Natural Language Processing(2020)
摘要
It is challenging to perform lifelong language learning (LLL) on a stream of different tasks without any performance degradation comparing to the multi-task counterparts. To address this issue, we present Lifelong Language Knowledge Distillation (L2KD), a simple but efficient method that can be easily applied to existing LLL architectures in order to mitigate the degradation. Specifically, when the LLL model is trained on a new task, we assign a teacher model to first learn the new task, and pass the knowledge to the LLL model via knowledge distillation. Therefore, the LLL model can better adapt to the new task while keeping the previously learned knowledge. Experiments show that the proposed L2KD consistently improves previous state-of-the-art models, and the degradation comparing to multi-task models in LLL tasks is well mitigated for both sequence generation and text classification tasks.
更多查看译文
关键词
knowledge,language
AI 理解论文
溯源树
样例
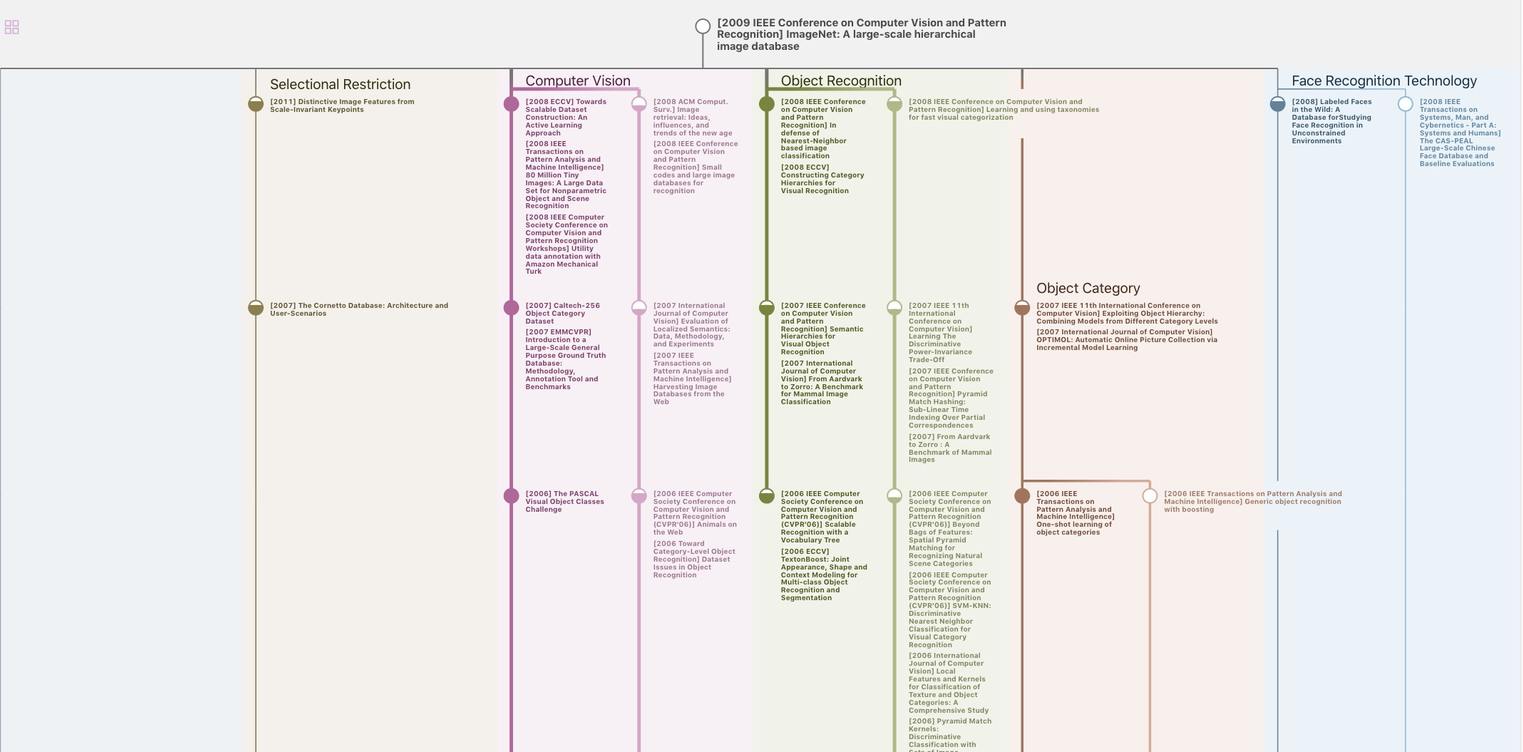
生成溯源树,研究论文发展脉络
Chat Paper
正在生成论文摘要