Unsupervised Text Style Transfer with Padded Masked Language Models
Conference on Empirical Methods in Natural Language Processing(2020)
摘要
We propose Masker, an unsupervised text-editing method for style transfer. To tackle cases when no parallel source–target pairs are available, we train masked language models (MLMs) for both the source and the target domain. Then we find the text spans where the two models disagree the most in terms of likelihood. This allows us to identify the source tokens to delete to transform the source text to match the style of the target domain. The deleted tokens are replaced with the target MLM, and by using a padded MLM variant, we avoid having to predetermine the number of inserted tokens. Our experiments on sentence fusion and sentiment transfer demonstrate that Masker performs competitively in a fully unsupervised setting. Moreover, in low-resource settings, it improves supervised methods’ accuracy by over 10 percentage points when pre-training them on silver training data generated by Masker.
更多查看译文
关键词
padded masked language models,text,transfer,style
AI 理解论文
溯源树
样例
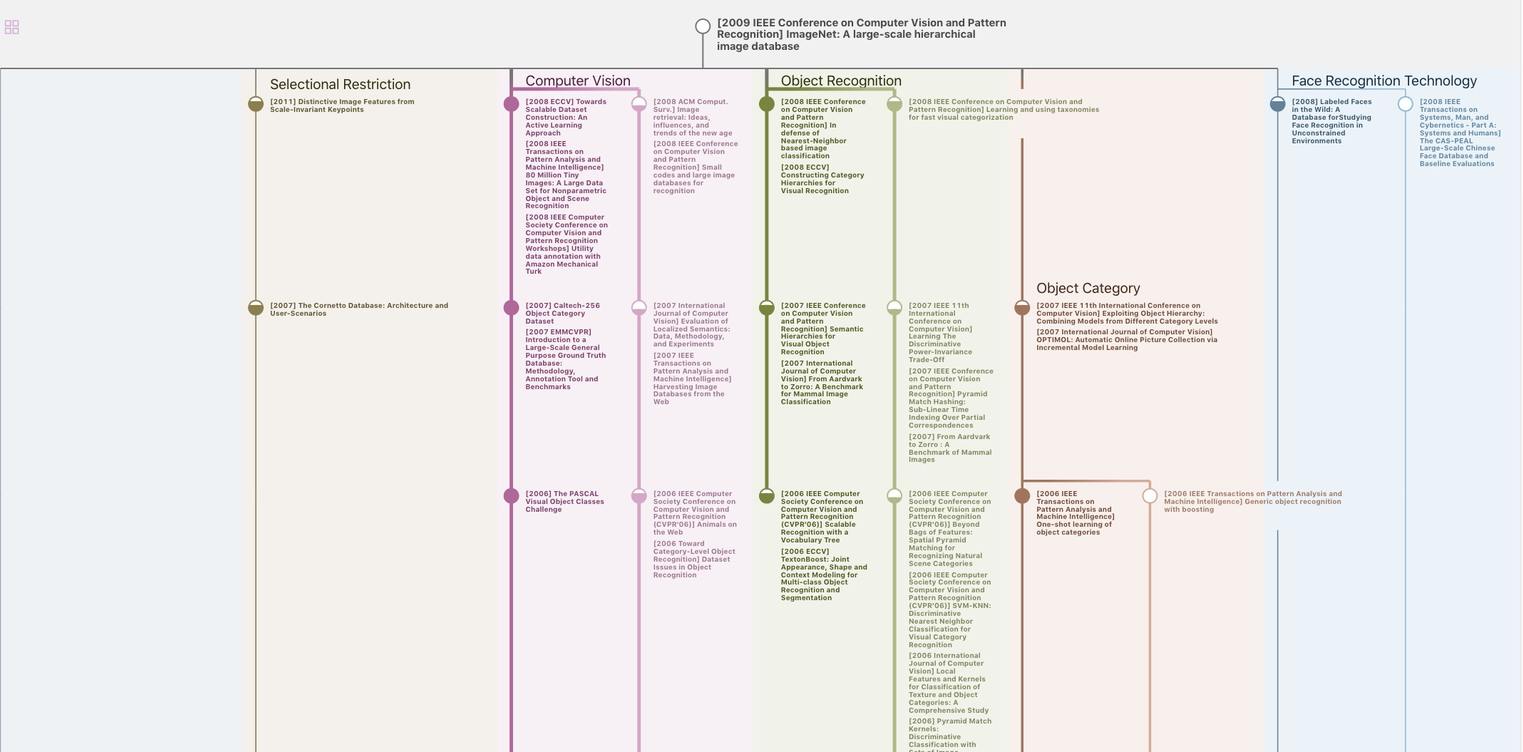
生成溯源树,研究论文发展脉络
Chat Paper
正在生成论文摘要