Adaptive Actuator Failure Compensation Control Schemes for Uncertain Noncanonical Neural-Network Systems
IEEE Transactions on Cybernetics(2022)
摘要
In this article, direct adaptive actuator failure compensation control is investigated for a class of noncanonical neural-network nonlinear systems whose relative degrees are implicit and parameters are unknown. Both the state tracking and output tracking control problems are considered, and their adaptive solutions are developed which have specific mechanisms to accommodate both actuator failures and parameter uncertainties to ensure the closed-loop system stability and asymptotic state or output tracking. The adaptive actuator failure compensation control schemes are derived for noncanonical nonlinear systems with neural-network approximation, and are also applicable to general parametrizable noncanonical nonlinear systems with both unknown actuator failures and unknown parameters, solving some key technical issues, in particular, dealing with the system zero dynamics under uncertain actuator failures. The effectiveness of the developed adaptive control schemes is confirmed by simulation results from an application example of speed control of dc motors.
更多查看译文
关键词
Computer Simulation,Feedback,Neural Networks, Computer,Nonlinear Dynamics
AI 理解论文
溯源树
样例
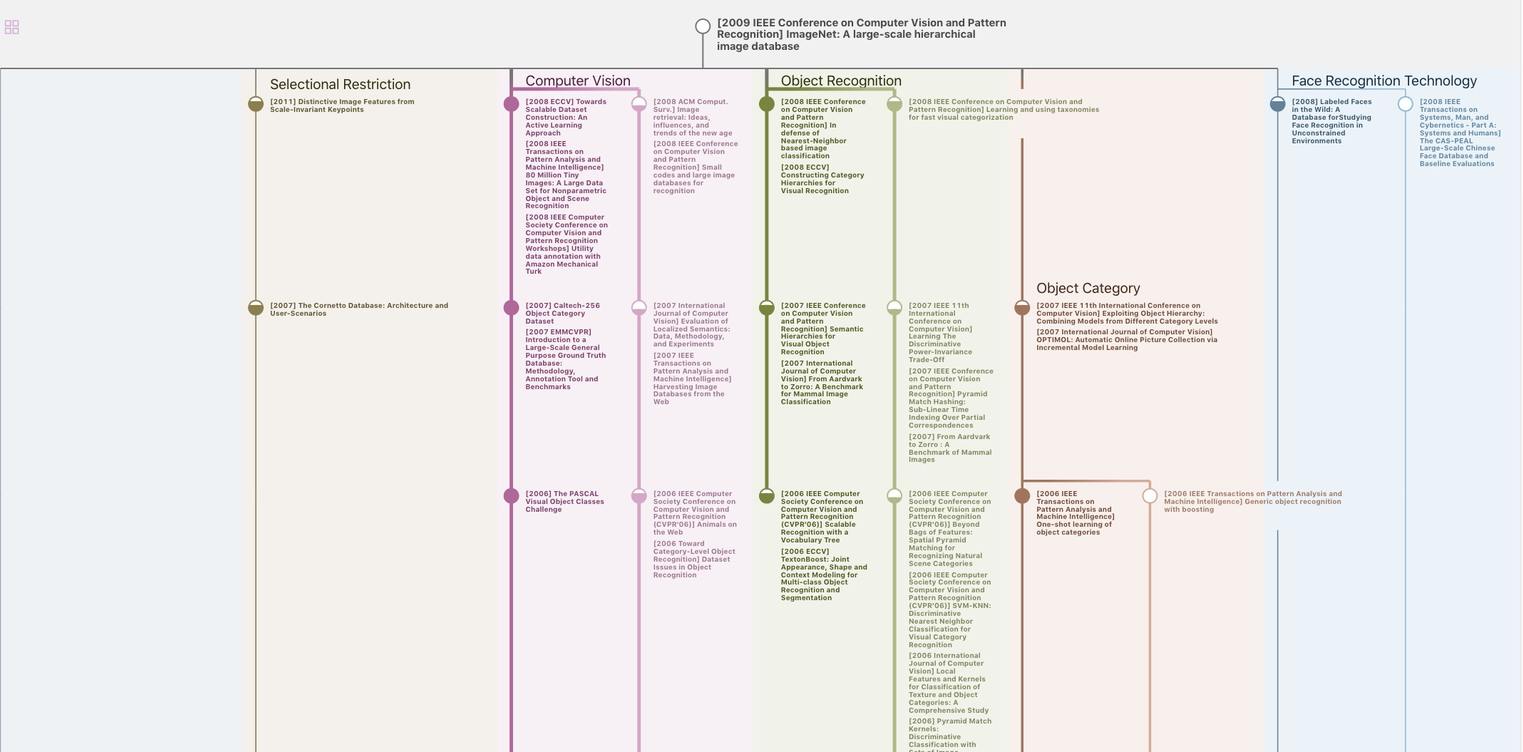
生成溯源树,研究论文发展脉络
Chat Paper
正在生成论文摘要