$\mathbb {H}$ H -Patches: A Benchmark and Evaluation of Handcrafted and Learned Local Descriptors
IEEE Transactions on Pattern Analysis and Machine Intelligence(2020)
摘要
In this paper, a novel benchmark is introduced for evaluating local image descriptors. We demonstrate limitations of the commonly used datasets and evaluation protocols, that lead to ambiguities and contradictory results in the literature. Furthermore, these benchmarks are nearly saturated due to the recent improvements in local descriptors obtained by learning from large annotated datasets. To address these issues, we introduce a new large dataset suitable for training and testing modern descriptors, together with strictly defined evaluation protocols in several tasks such as matching, retrieval and verification. This allows for more realistic, thus more reliable comparisons in different application scenarios. We evaluate the performance of several state-of-the-art descriptors and analyse their properties. We show that a simple normalisation of traditional hand-crafted descriptors is able to boost their performance to the level of deep learning based descriptors once realistic benchmarks are considered. Additionally we specify a protocol for learning and evaluating using cross validation. We show that when training state-of-the-art descriptors on this dataset, the traditional verification task is almost entirely saturated.
更多查看译文
关键词
Benchmark testing,Detectors,Protocols,Task analysis,Feature extraction,Training,Image matching
AI 理解论文
溯源树
样例
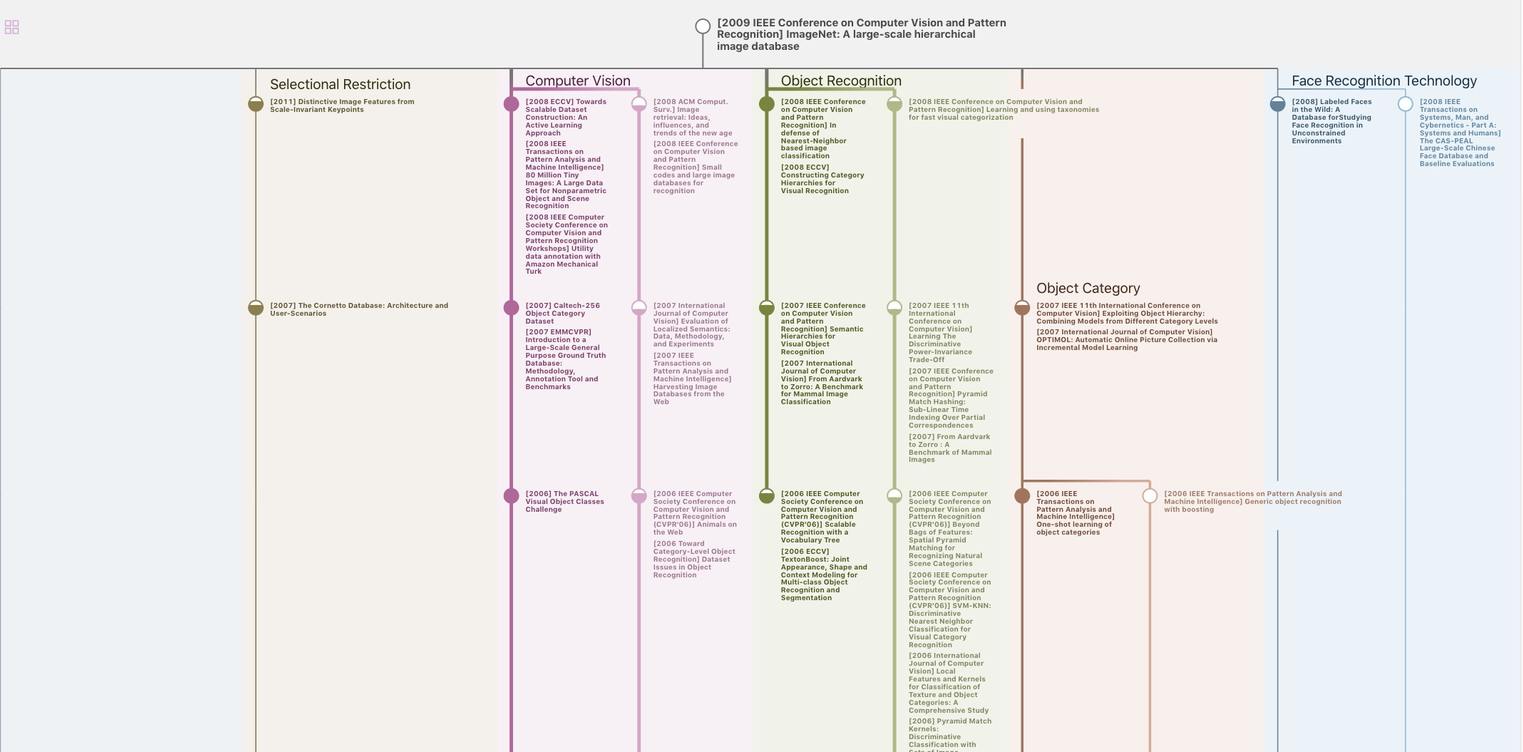
生成溯源树,研究论文发展脉络
Chat Paper
正在生成论文摘要