Uncertainty Reasoning for Probabilistic Petri Nets via Bayesian Networks
FSTTCS(2020)
摘要
This paper exploits extended Bayesian networks for uncertainty reasoning on Petri nets, where firing of transitions is probabilistic. In particular, Bayesian networks are used as symbolic representations of probability distributions, modelling the observer's knowledge about the tokens in the net. The observer can study the net by monitoring successful and failed steps. An update mechanism for Bayesian nets is enabled by relaxing some of their restrictions, leading to modular Bayesian nets that can conveniently be represented and modified. As for every symbolic representation, the question is how to derive information - in this case marginal probability distributions - from a modular Bayesian net. We show how to do this by generalizing the known method of variable elimination. The approach is illustrated by examples about the spreading of diseases (SIR model) and information diffusion in social networks. We have implemented our approach and provide runtime results.
更多查看译文
关键词
probabilistic petri nets,uncertainty,networks,reasoning
AI 理解论文
溯源树
样例
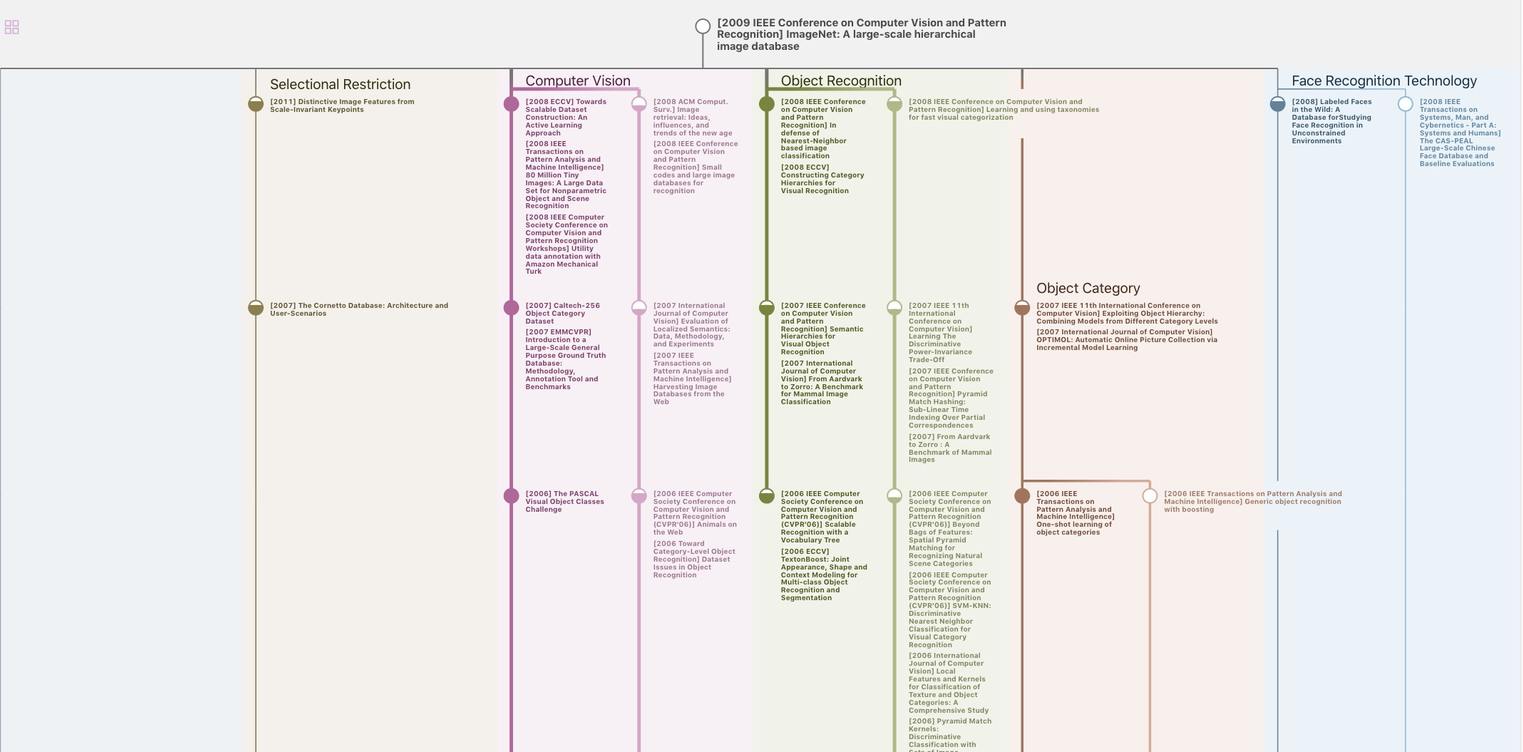
生成溯源树,研究论文发展脉络
Chat Paper
正在生成论文摘要