Redbot: Natural Language Process Methods For Clinical Copy Number Variation Reporting In Prenatal And Products Of Conception Diagnosis
MOLECULAR GENETICS & GENOMIC MEDICINE(2020)
摘要
Background: Current copy number variation (CNV) identification methods have rapidly become mature. However, the postdetection processes such as variant interpretation or reporting are inefficient. To overcome this situation, we developed REDBot as an automated software package for accurate and direct generation of clinical diagnostic reports for prenatal and products of conception (POC) samples.Methods: We applied natural language process (NLP) methods for analyzing 30,235 in-house historical clinical reports through active learning, and then, developed clinical knowledge bases, evidence-based interpretation methods and reporting criteria to support the whole postdetection pipeline.Results: Of the 30,235 reports, we obtained 37,175 CNV-paragraph pairs. For these pairs, the active learning approaches achieved a 0.9466 average F1-score in sentence classification. The overall accuracy for variant classification was 95.7%, 95.2%, and 100.0% in retrospective, prospective, and clinical utility experiments, respectively.Conclusion: By integrating NLP methods in CNVs postdetection pipeline, REDBot is a robust and rapid tool with clinical utility for prenatal and POC diagnosis.
更多查看译文
关键词
active learning, copy number variation, natural language process, prenatal diagnosis, variant classification
AI 理解论文
溯源树
样例
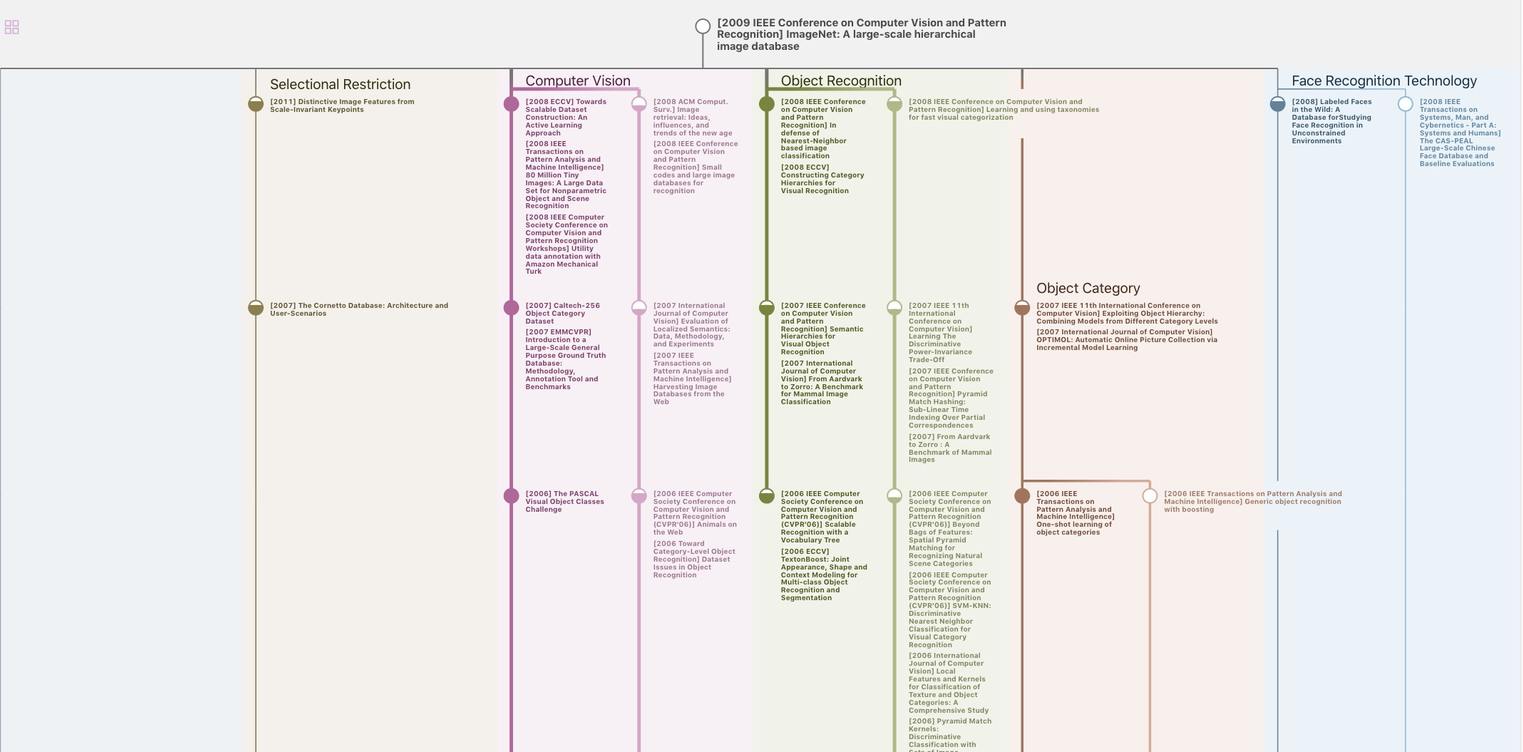
生成溯源树,研究论文发展脉络
Chat Paper
正在生成论文摘要