My Health Sensor, My Classifier – Adapting a Trained Classifier to Unlabeled End-User Data
ACM Transactions on Computing for Healthcare(2022)
摘要
AbstractSleep apnea is a common yet severely under-diagnosed sleep related disorder. Unattended sleep monitoring at home with low-cost sensors can be leveraged for condition detection, and Machine Learning offers a generalized solution for this task. However, patient characteristics, lack of sufficient training data, and other factors can imply a domain shift between training and end-user data and reduced task performance. In this work, we address this issue with the aim to achieve personalization based on the patient’s needs. We present an unsupervised domain adaptation (UDA) solution with the constraint that labeled source data are not directly available. Instead, a classifier trained on the source data is provided. Our solution iteratively labels target data sub-regions based on classifier beliefs, and trains new classifiers from the expanding dataset. Experiments with sleep monitoring datasets and various sensors show that our solution outperforms the classifier trained on the source domain, with a kappa coefficient improvement from 0.012 to 0.242. Additionally, we apply our solution to digit classification DA between three well-established datasets, to investigate its generalizability, and allow for related work comparisons. Even without direct access to the source data, it outperforms several well-established UDA methods in these datasets.
更多查看译文
关键词
health sensor,classifier,data,end-user
AI 理解论文
溯源树
样例
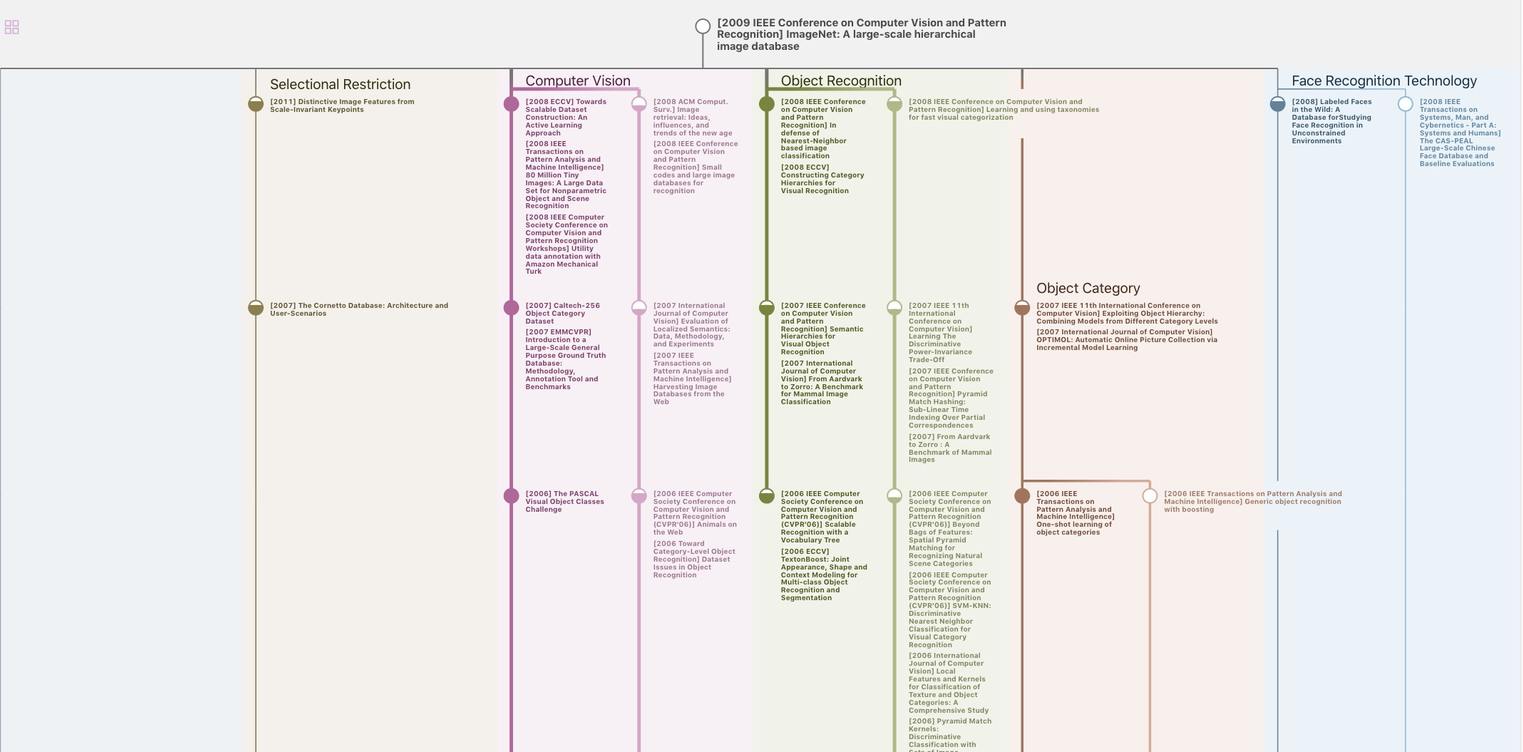
生成溯源树,研究论文发展脉络
Chat Paper
正在生成论文摘要