Property-Directed Verification and Robustness Certification of Recurrent Neural Networks
arxiv(2021)
摘要
This paper presents a property-directed approach to verifying recurrent neural networks (RNNs). To this end, we learn a deterministic finite automaton as a surrogate model from a given RNN using active automata learning. This model may then be analyzed using model checking as a verification technique. The term property-directed reflects the idea that our procedure is guided and controlled by the given property rather than performing the two steps separately. We show that this not only allows us to discover small counterexamples fast, but also to generalize them by pumping towards faulty flows hinting at the underlying error in the RNN. We also show that our method can be efficiently used for adversarial robustness certification of RNNs.
更多查看译文
关键词
recurrent neural networks,robustness certification,neural networks,verification,property-directed
AI 理解论文
溯源树
样例
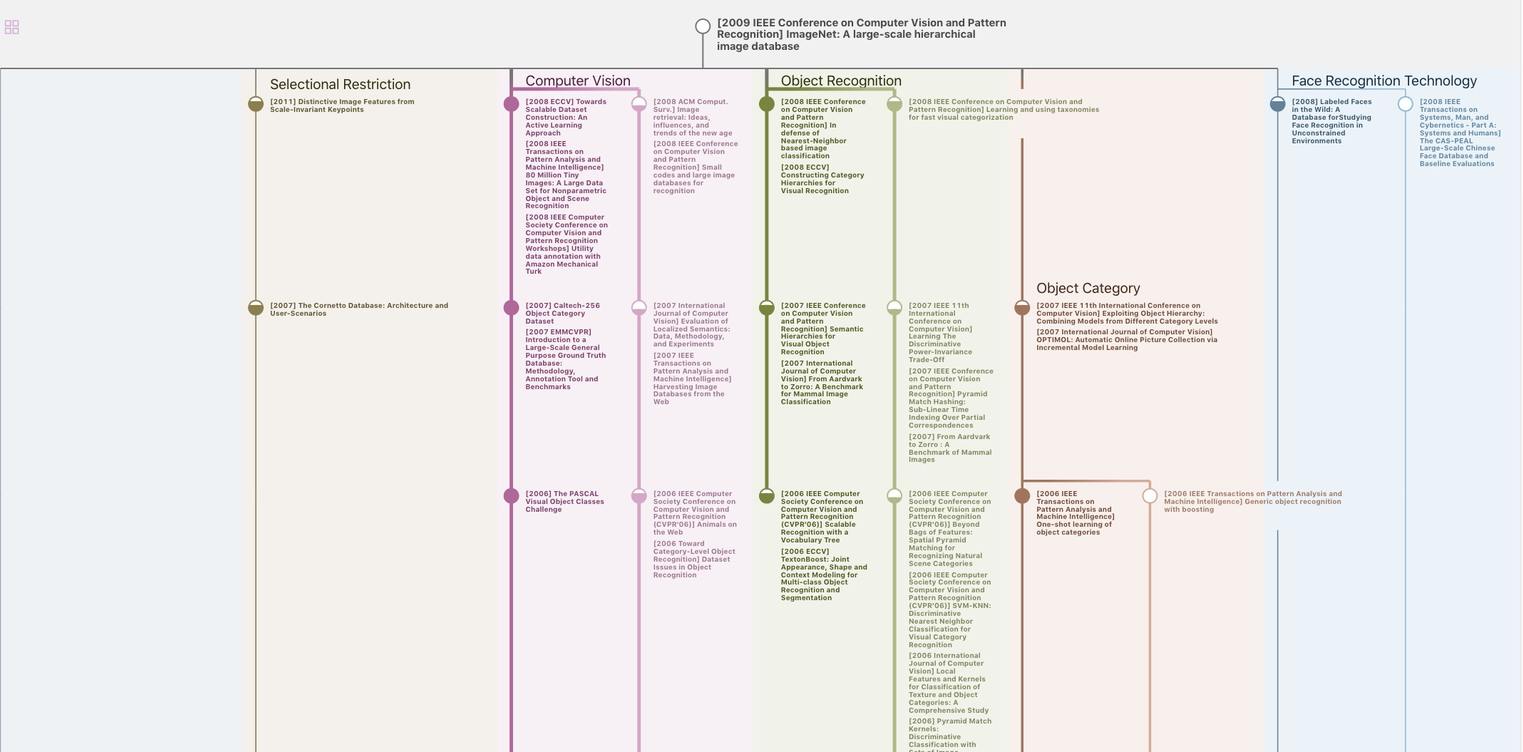
生成溯源树,研究论文发展脉络
Chat Paper
正在生成论文摘要