Knowledge-based classification of fine-grained immune cell types in single-cell RNA-Seq data
biorxiv(2021)
摘要
Single-cell RNA sequencing (scRNA-Seq) is an emerging strategy for characterizing immune cell populations. Compared to flow or mass cytometry, scRNA-Seq could potentially identify cell types and activation states that lack precise cell surface markers. However, scRNA-Seq is currently limited due to the need to manually classify each immune cell from its transcriptional profile. While recently developed algorithms accurately annotate coarse cell types (e.g. T cells versus macrophages), making fine distinctions (e.g. CD8(+) effector memory T cells) remains a difficult challenge. To address this, we developed a machine learning classifier called ImmClassifier that leverages a hierarchical ontology of cell type. We demonstrate that its predictions are highly concordant with flow-based markers from CITE-seq and outperforms other tools (+15% recall, +14% precision) in distinguishing fine-grained cell types with comparable performance on coarse ones. Thus, ImmClassifier can be used to explore more deeply the heterogeneity of the immune system in scRNA-Seq experiments.
更多查看译文
关键词
deep learning, immune cell classification, machine learning, single-cell RNA-Seq
AI 理解论文
溯源树
样例
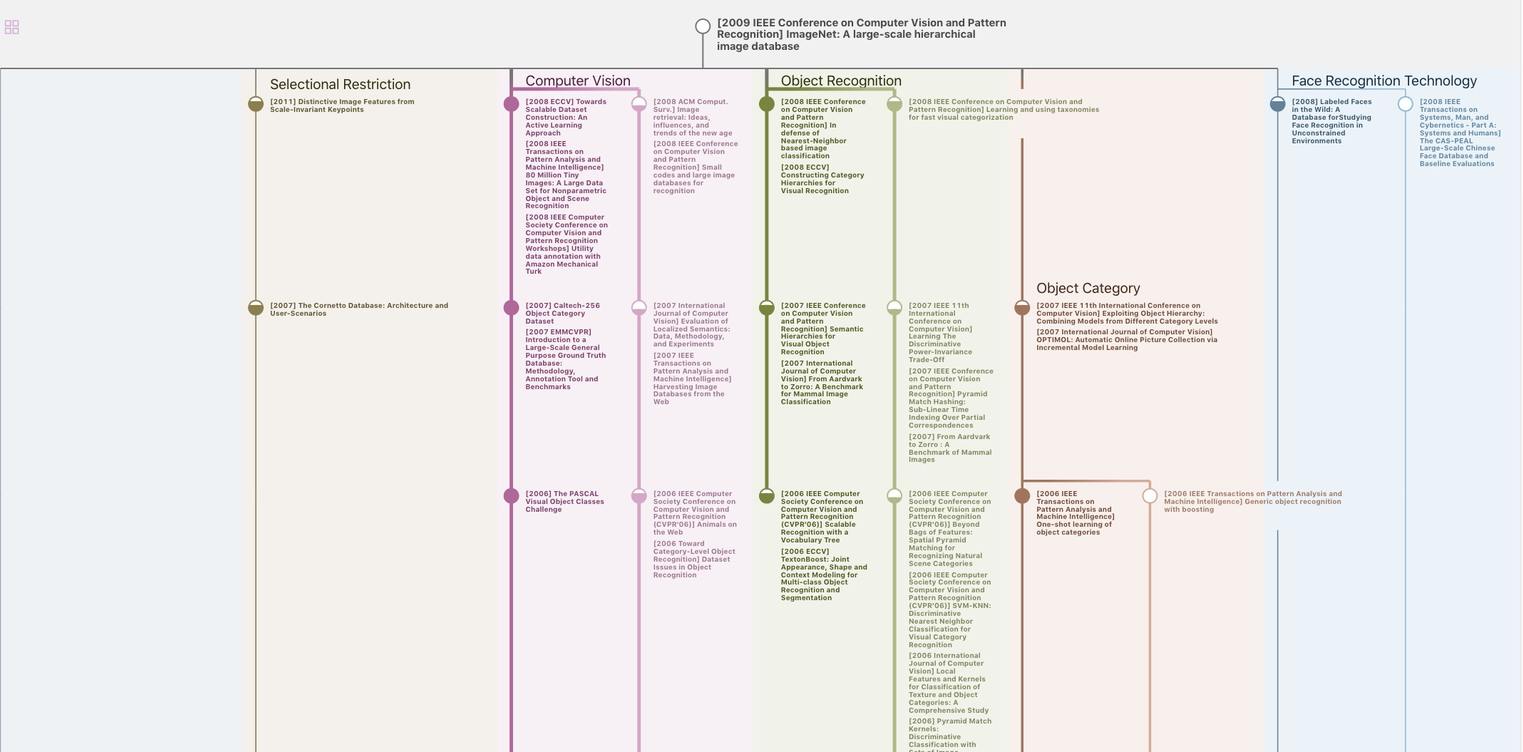
生成溯源树,研究论文发展脉络
Chat Paper
正在生成论文摘要