Evidence accumulation and associated error-related brain activity as computationally-informed prospective predictors of substance use in emerging adulthood
Psychopharmacology(2021)
摘要
Rationale Substance use peaks during the developmental period known as emerging adulthood (ages 18–25), but not every individual who uses substances during this period engages in frequent or problematic use. Although individual differences in neurocognition appear to predict use severity, mechanistic neurocognitive risk factors with clear links to both behavior and neural circuitry have yet to be identified. Here, we aim to do so with an approach rooted in computational psychiatry, an emerging field in which formal models are used to identify candidate biobehavioral dimensions that confer risk for psychopathology. Objectives We test whether lower efficiency of evidence accumulation (EEA), a computationally characterized individual difference variable that drives performance on the go/no-go and other neurocognitive tasks, is a risk factor for substance use in emerging adults. Methods and results In an fMRI substudy within a sociobehavioral longitudinal study ( n = 106), we find that lower EEA and reductions in a robust neural-level correlate of EEA (error-related activations in salience network structures) measured at ages 18–21 are both prospectively related to greater substance use during ages 22–26, even after adjusting for other well-known risk factors. Results from Bayesian model comparisons corroborated inferences from conventional hypothesis testing and provided evidence that both EEA and its neuroimaging correlates contain unique predictive information about substance use involvement. Conclusions These findings highlight EEA as a computationally characterized neurocognitive risk factor for substance use during a critical developmental period, with clear links to both neuroimaging measures and well-established formal theories of brain function.
更多查看译文
关键词
Computational psychiatry,Substance use,Diffusion model,Evidence accumulation,Drift rate,Salience network
AI 理解论文
溯源树
样例
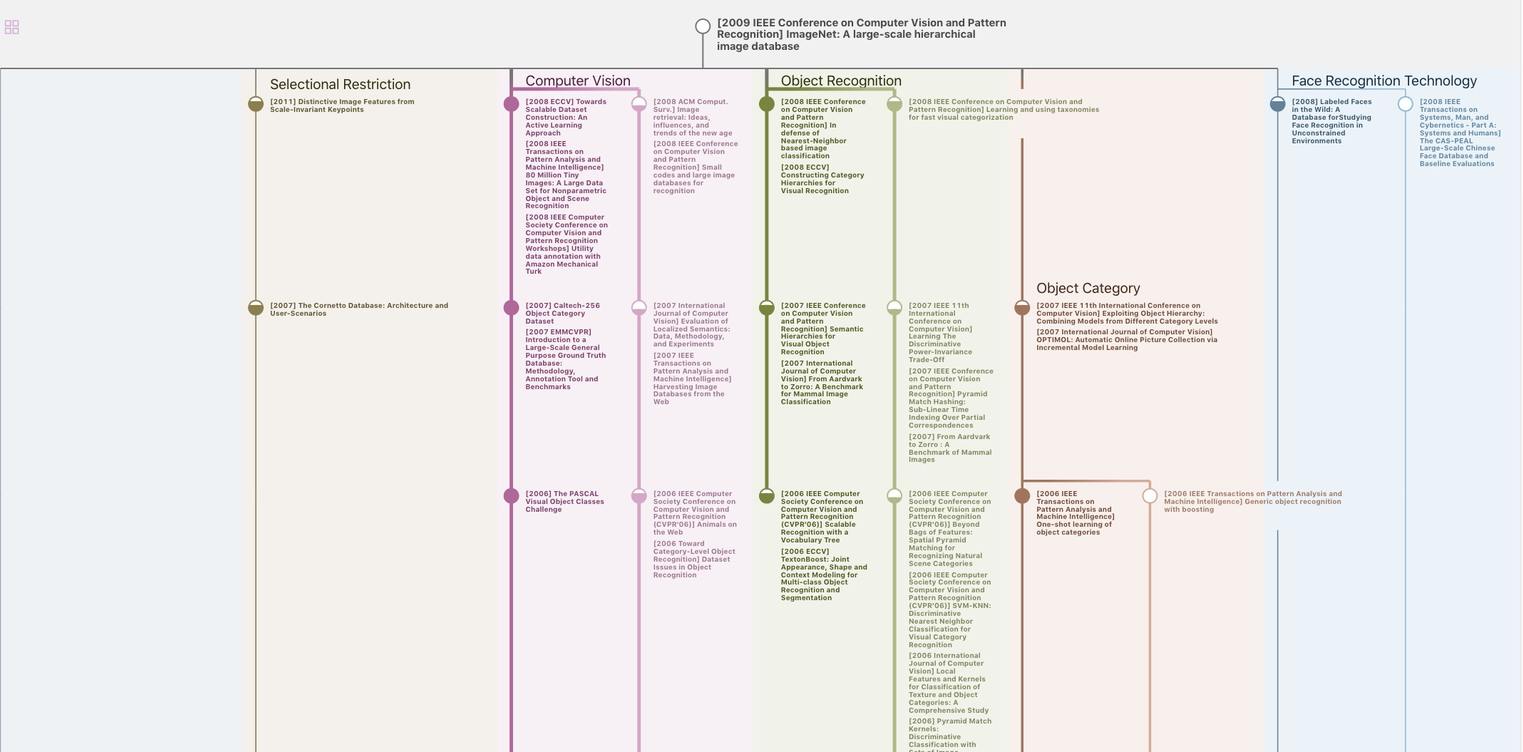
生成溯源树,研究论文发展脉络
Chat Paper
正在生成论文摘要