Predicting residues involved in anti-DNA autoantibodies with limited neural networks
biorxiv(2022)
摘要
Computer-aided rational vaccine design (RVD) and synthetic pharmacology are rapidly developing fields that leverage existing datasets for developing compounds of interest. Computational proteomics utilizes algorithms and models to probe proteins for functional prediction. A potentially strong target for computational approach is autoimmune antibodies, which are the result of broken tolerance in the immune system where it cannot distinguish “self” from “non-self” resulting in attack of its own structures (proteins and DNA, mainly). The information on structure, function, and pathogenicity of autoantibodies may assist in engineering RVD against autoimmune diseases. Current computational approaches exploit large datasets curated with extensive domain knowledge, most of which include the need for many resources and have been applied indirectly to problems of interest for DNA, RNA, and monomer protein binding. We present a novel method for discovering potential binding sites. We employed long short-term memory (LSTM) models trained on FASTA primary sequences to predict protein binding in DNA-binding hydrolytic antibodies (abzymes). We also employed CNN models applied to the same dataset for comparison with LSTM. While the CNN model outperformed the LSTM on the primary task of binding prediction, analysis of internal model representations of both models showed that the LSTM models recovered sub-sequences that were strongly correlated with sites known to be involved in binding. These results demonstrate that analysis of internal processes of LSTM models may serve as a powerful tool for primary sequence analysis. Graphical abstract
更多查看译文
关键词
Auto-immunity,Deep learning,DNA-binding,LSTM,Proteomics,Systemic lupus
AI 理解论文
溯源树
样例
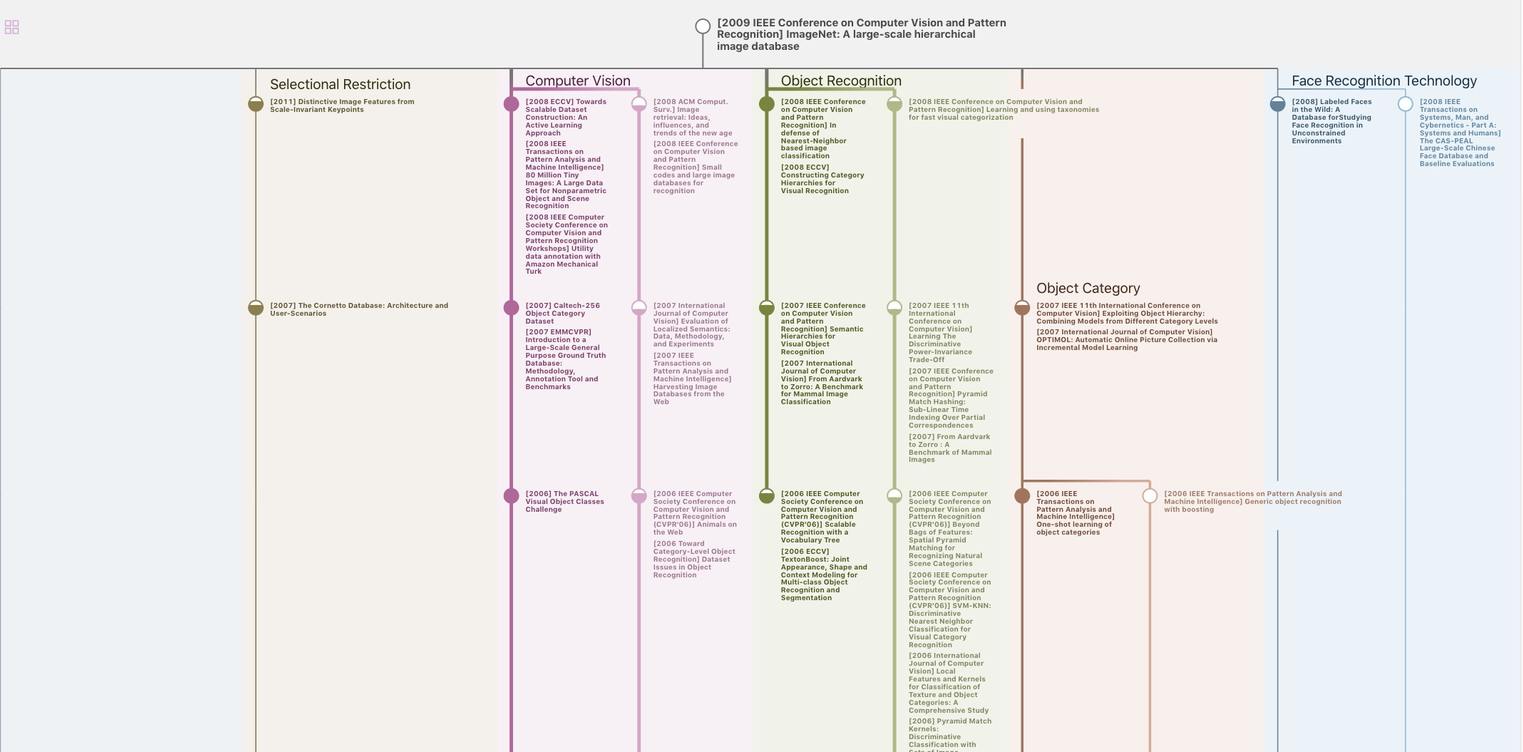
生成溯源树,研究论文发展脉络
Chat Paper
正在生成论文摘要