CLEP: A Hybrid Data- and Knowledge-Driven Framework for Generating Patient Representations
biorxiv(2020)
摘要
As machine learning and artificial intelligence become more useful in the interpretation of biomedical data, their utility depends on the data used to train them. Due to the complexity and high dimensionality of biomedical data, there is a need for approaches that combine prior knowledge around known biological interactions with patient data. Here, we present CLEP, a novel approach that generates new patient representations by leveraging both prior knowledge and patient-level data. First, given a patient-level dataset and a knowledge graph containing relations across features that can be mapped to the dataset, CLEP incorporates patients into the knowledge graph as new nodes connected to their most characteristic features. Next, CLEP employs knowledge graph embedding models to generate new patient representations that can ultimately be used for a variety of downstream tasks, ranging from clustering to classification. We demonstrate how using new patient representations generated by CLEP significantly improves performance in classifying between cognitively impaired patients and healthy controls for a variety of machine learning models, as compared to the use of the original transcriptomics data. Furthermore, we also show how incorporating patients into a knowledge graph can foster the interpretation and identification of biological features characteristic of a specific disease or patient subgroup. Finally, we released CLEP as an open source Python package together with examples and documentation.
更多查看译文
关键词
generating patient representations,clep,knowledge-driven
AI 理解论文
溯源树
样例
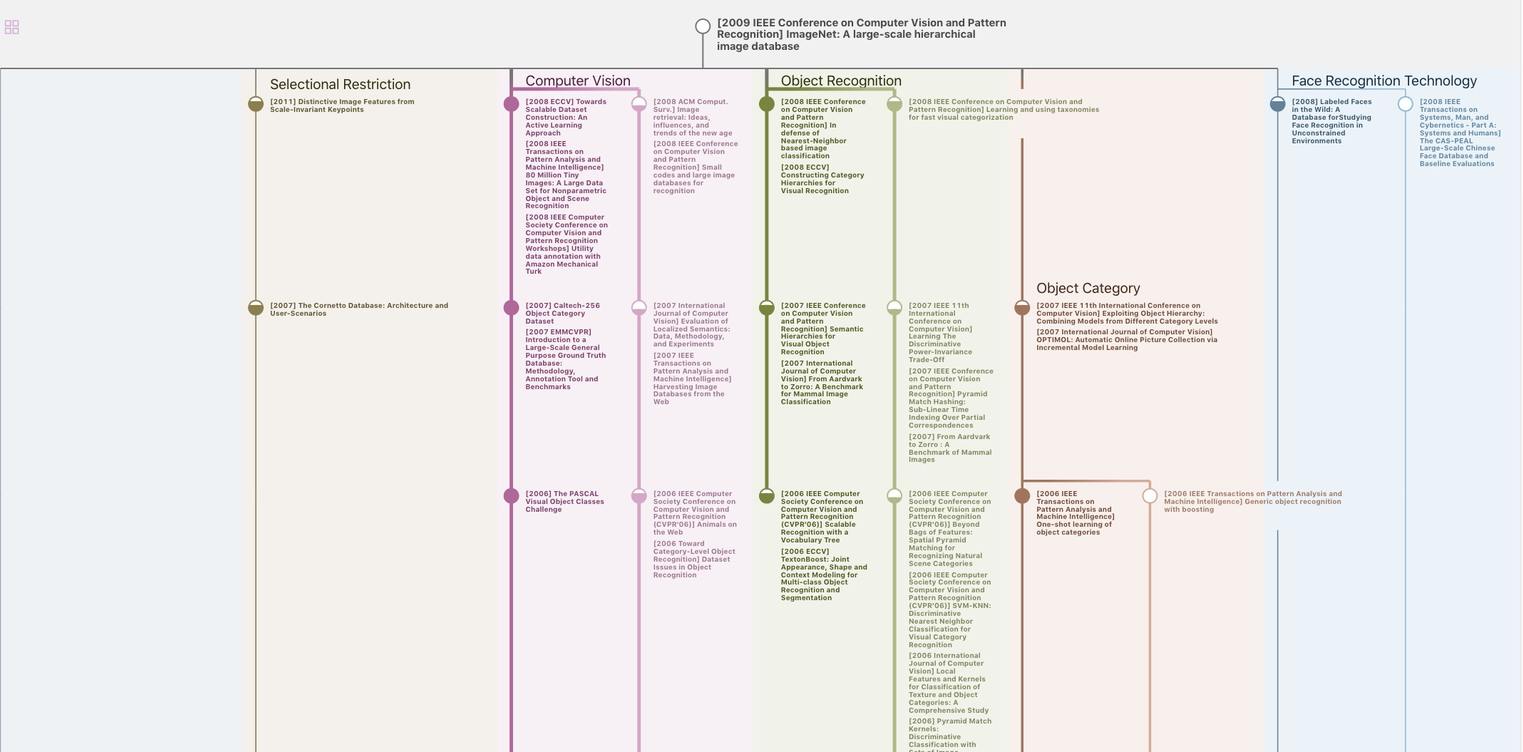
生成溯源树,研究论文发展脉络
Chat Paper
正在生成论文摘要