Pruning Neural Networks at Initialization: Why are We Missing the Mark?
ICLR(2021)
摘要
Recent work has explored the possibility of pruning neural networks at initialization. We assess proposals for doing so: SNIP (Lee et al., 2019), GraSP (Wang et al., 2020), SynFlow (Tanaka et al., 2020), and magnitude pruning. Although these methods surpass the trivial baseline of random pruning, they remain below the accuracy of magnitude pruning after training, and we endeavor to understand why. We show that, unlike pruning after training, accuracy is the same or higher when randomly shuffling which weights these methods prune within each layer or sampling new initial values. As such, the per-weight pruning decisions made by these methods can be replaced by a per-layer choice of the fraction of weights to prune. This property undermines the claimed justifications for these methods and suggests broader challenges with the underlying pruning heuristics, the desire to prune at initialization, or both.
更多查看译文
AI 理解论文
溯源树
样例
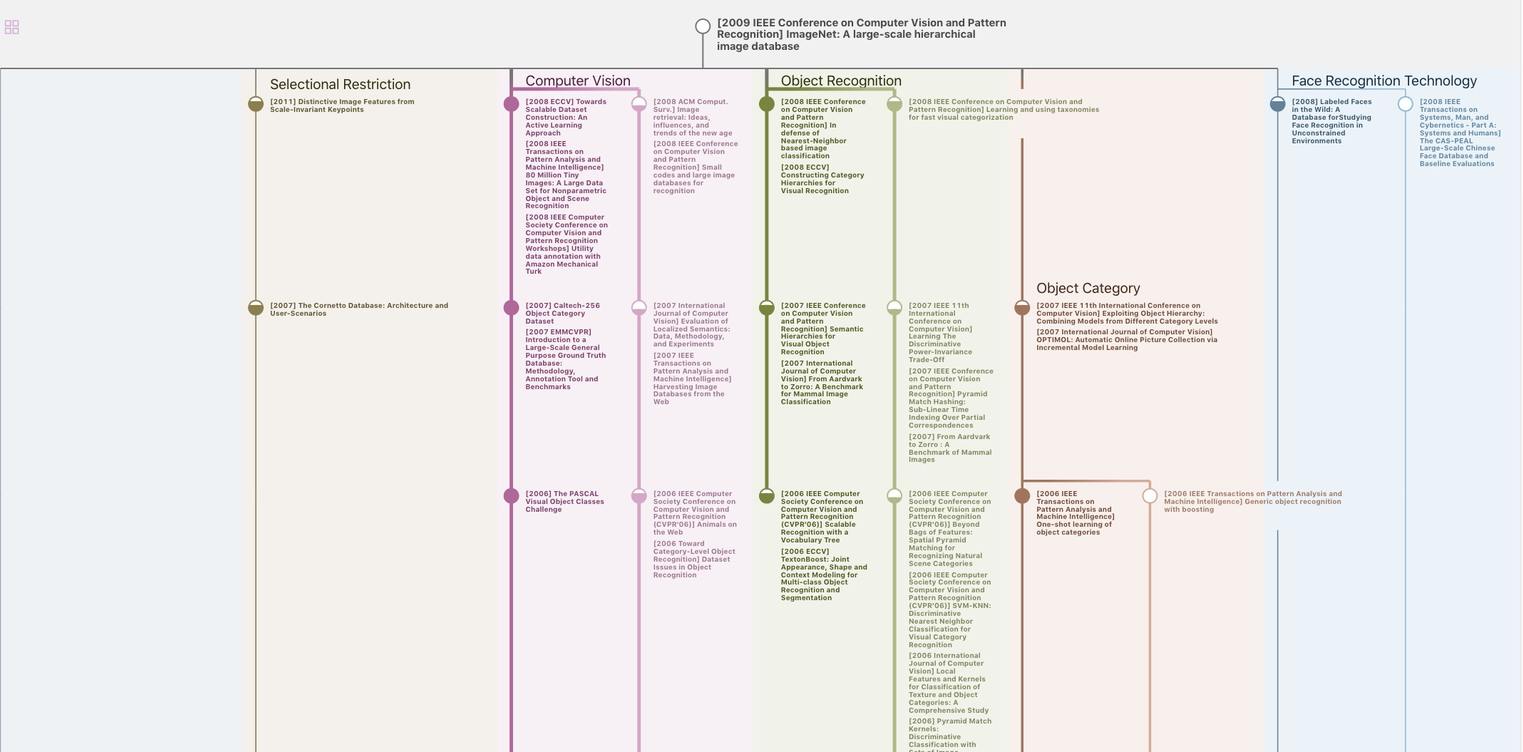
生成溯源树,研究论文发展脉络
Chat Paper
正在生成论文摘要