A Dependency Capturing Code for Robust Object Representation
biorxiv(2019)
摘要
The brain has a remarkable ability to recognize objects from noisy or corrupted sensory inputs. How this cognitive robustness is achieved computationally remains unknown. We present a coding paradigm, which encodes structural dependence among features of the input and transforms various forms of the same input into the same representation. The paradigm, through dimensionally expanded representation and sparsity constraint, allows redundant feature coding to enhance robustness and is efficient in representing objects. We demonstrate consistent representations of visual and olfactory objects under conditions of occlusion, high noise or with corrupted coding units. Robust face recognition is achievable without deep layers or large training sets. The paradigm produces both complex and simple receptive fields depending on learning experience, thereby offers a unifying framework of sensory processing.
更多查看译文
AI 理解论文
溯源树
样例
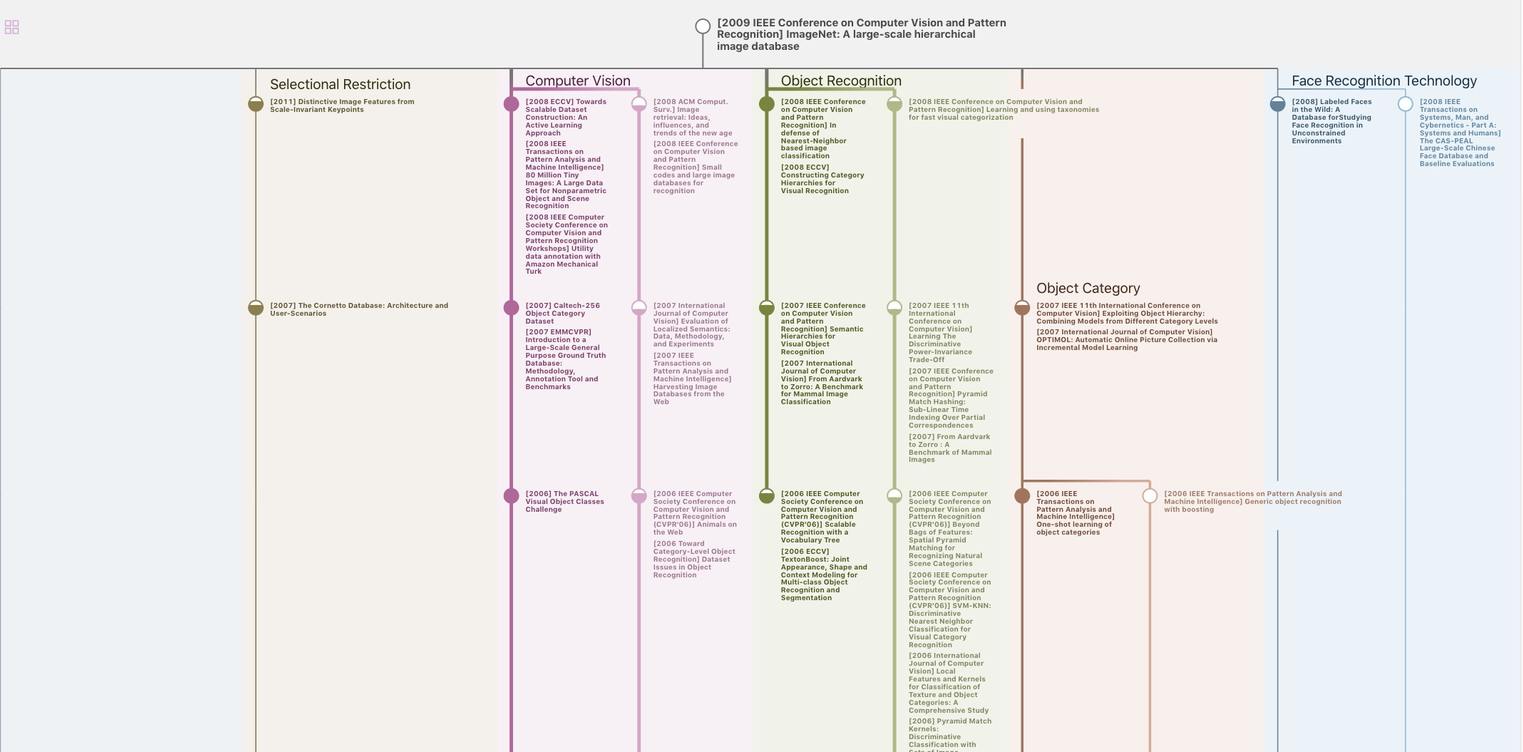
生成溯源树,研究论文发展脉络
Chat Paper
正在生成论文摘要