Holistic Filter Pruning For Efficient Deep Neural Networks
2021 IEEE WINTER CONFERENCE ON APPLICATIONS OF COMPUTER VISION WACV 2021(2021)
摘要
Deep neural networks (DNNs) are usually over-parameterized to increase the likelihood of getting adequate initial weights by random initialization. Consequently, trained DNNs have many redundancies which can be pruned from the model to reduce complexity and improve the ability to generalize. Structural sparsity, as achieved by filter pruning, directly reduces the tensor sizes of weights and activations and is thus particularly effective for reducing complexity. We propose Holistic Filter Pruning (HFP), a novel approach for common DNN training that is easy to implement and enables to specify accurate pruning rates for the number of both parameters and multiplications. After each forward pass, the current model size is calculated and compared to the desired target size. By gradient descent, a global solution can be found that allocates the pruning budget over the individual layers such that the desired target size is fulfilled. In various experiments, we give insights into the training and achieve state-of-the-art performance on CIFAR-10 and ImageNet.
更多查看译文
关键词
efficient deep neural networks,neural networks
AI 理解论文
溯源树
样例
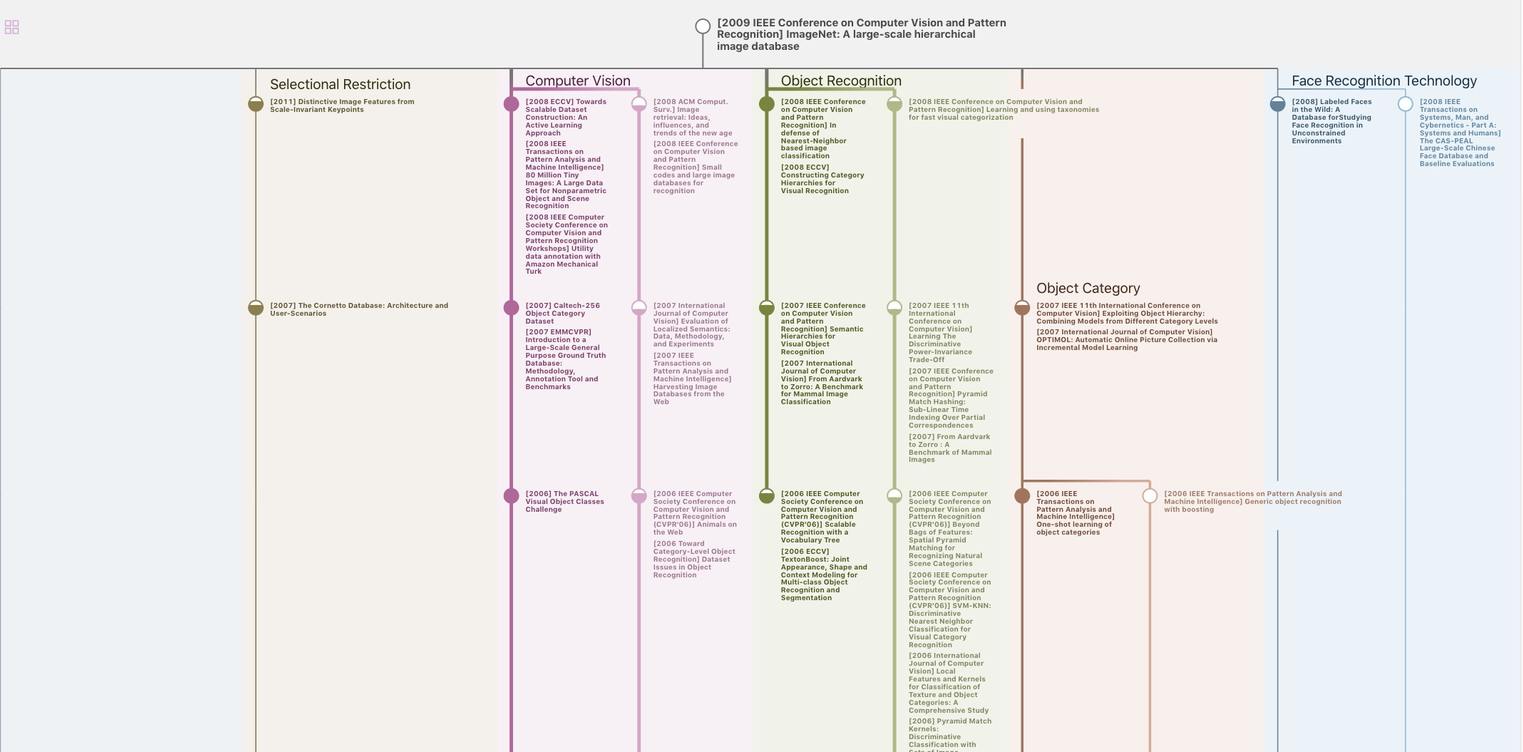
生成溯源树,研究论文发展脉络
Chat Paper
正在生成论文摘要