Systematic Generalization on gSCAN with Language Conditioned Embedding
AACL/IJCNLP(2020)
摘要
Systematic Generalization refers to a learning algorithm's ability to extrapolate learned behavior to unseen situations that are distinct but semantically similar to its training data. As shown in recent work, state-of-the-art deep learning models fail dramatically even on tasks for which they are designed when the test set is systematically different from the training data. We hypothesize that explicitly modeling the relations between objects in their contexts while learning their representations will help achieve systematic generalization. Therefore, we propose a novel method that learns objects' contextualized embeddings with dynamic message passing conditioned on the input natural language and end-to-end trainable with other downstream deep learning modules. To our knowledge, this model is the first one that significantly outperforms the provided baseline and reaches state-of-the-art performance on grounded-SCAN (gSCAN), a grounded natural language navigation dataset designed to require systematic generalization in its test splits.
更多查看译文
关键词
gscan,systematic generalization,language
AI 理解论文
溯源树
样例
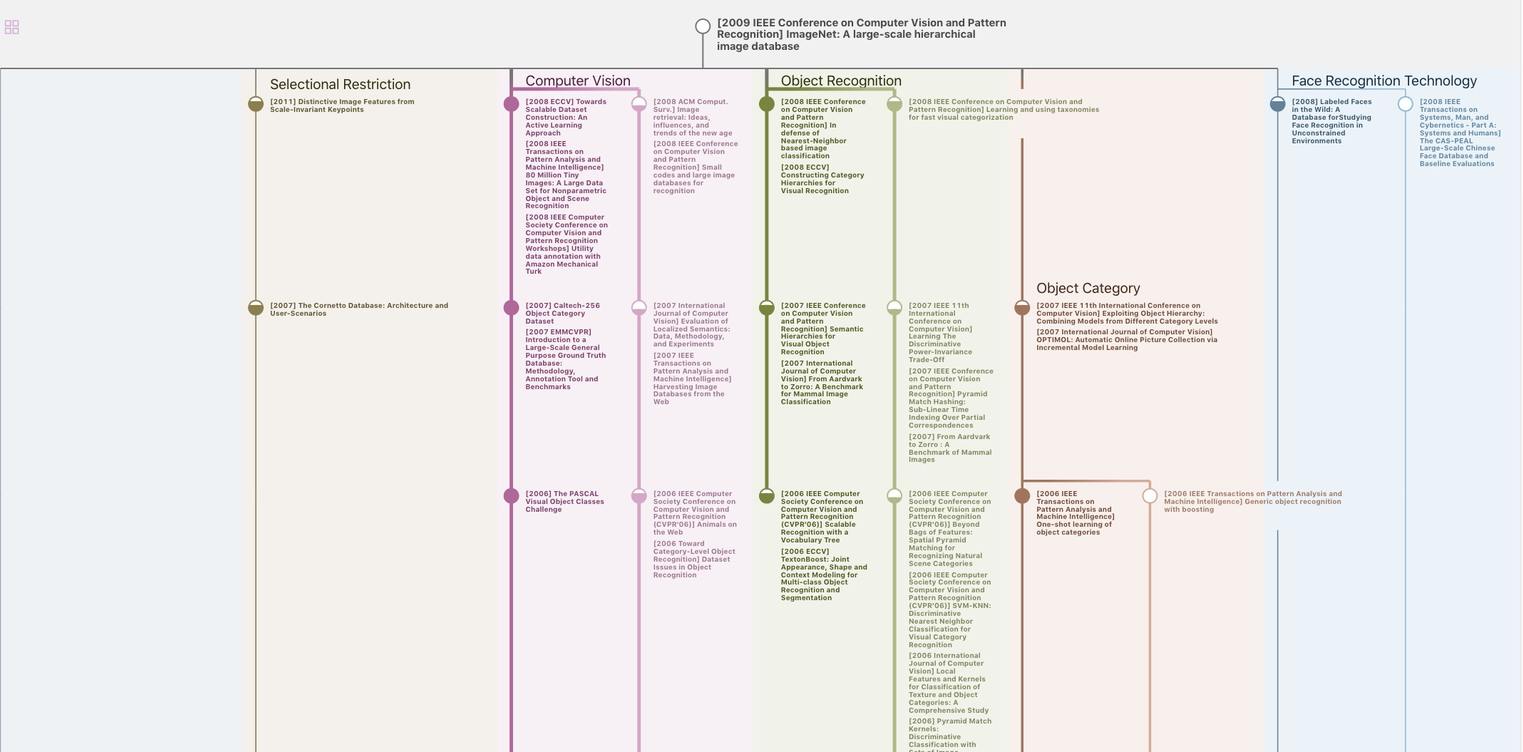
生成溯源树,研究论文发展脉络
Chat Paper
正在生成论文摘要