Visual Neural Decomposition To Explain Multivariate Data Sets
IEEE TRANSACTIONS ON VISUALIZATION AND COMPUTER GRAPHICS(2021)
摘要
Investigating relationships between variables in multi-dimensional data sets is a common task for data analysts and engineers. More specifically, it is often valuable to understand which ranges of which input variables lead to particular values of a given target variable. Unfortunately, with an increasing number of independent variables, this process may become cumbersome and time-consuming due to the many possible combinations that have to be explored. In this paper, we propose a novel approach to visualize correlations between input variables and a target output variable that scales to hundreds of variables. We developed a visual model based on neural networks that can be explored in a guided way to help analysts find and understand such correlations. First, we train a neural network to predict the target from the input variables. Then, we visualize the inner workings of the resulting model to help understand relations within the data set. We further introduce a new regularization term for the backpropagation algorithm that encourages the neural network to learn representations that are easier to interpret visually. We apply our method to artificial and real-world data sets to show its utility.
更多查看译文
关键词
Data visualization, Visualization, Correlation, Analytical models, Input variables, Neural networks, Semiconductor device measurement, Visual Analytics, Multivariate Data Analysis, Machine Learning
AI 理解论文
溯源树
样例
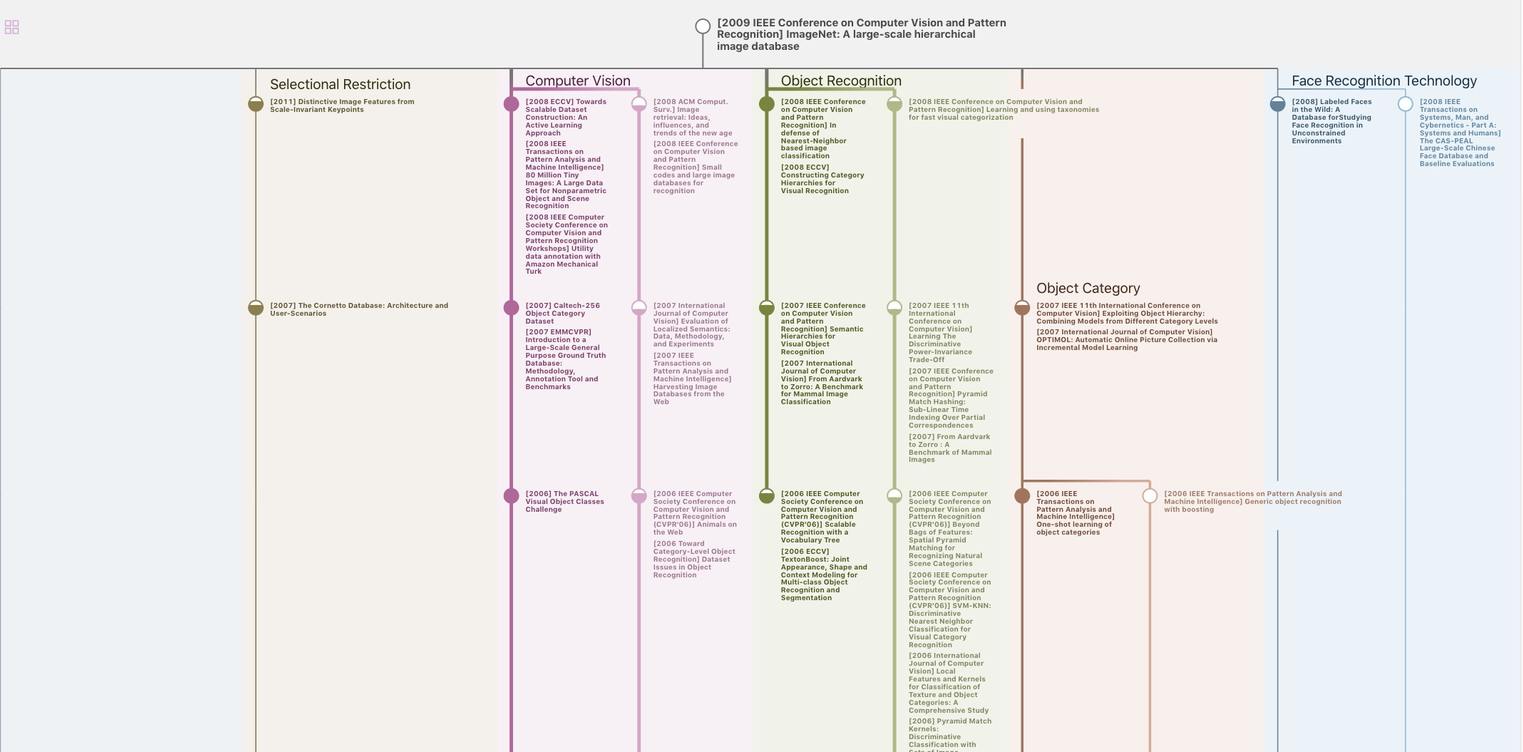
生成溯源树,研究论文发展脉络
Chat Paper
正在生成论文摘要