Roifusion: 3d Object Detection From Lidar And Vision
IEEE ACCESS(2021)
摘要
When localizing and detecting 3D objects for autonomous driving scenes, obtaining information from multiple sensors (e.g., camera, LIDAR) is capable of mutually offering useful complementary information to enhance the robustness of 3D detectors. In this paper, a deep neural network architecture, named RoIFusion, is proposed to efficiently fuse the multi-modality features for 3D object detection by leveraging the advantages of LIDAR and camera sensors. In order to achieve this task, instead of densely combining the point-wise feature of the point cloud with the related pixel features, our fusion method novelly aggregates a small set of 3D Region of Interests (RoIs) in the point clouds with the corresponding 2D RoIs in the images, which are beneficial for reducing the computation cost and avoiding the viewpoint misalignment during the feature aggregation from different sensors. Finally, Extensive experiments are performed on the KITTI 3D object detection challenging benchmark to show the effectiveness of our fusion method and demonstrate that our deep fusion approach achieves state-of-the-art performance.
更多查看译文
关键词
Three-dimensional displays, Feature extraction, Two dimensional displays, Object detection, Neural networks, Detectors, Sensor fusion, Sensors fusion, 3D object detection, Region of Interests, neural network, segmentation network, point cloud, image
AI 理解论文
溯源树
样例
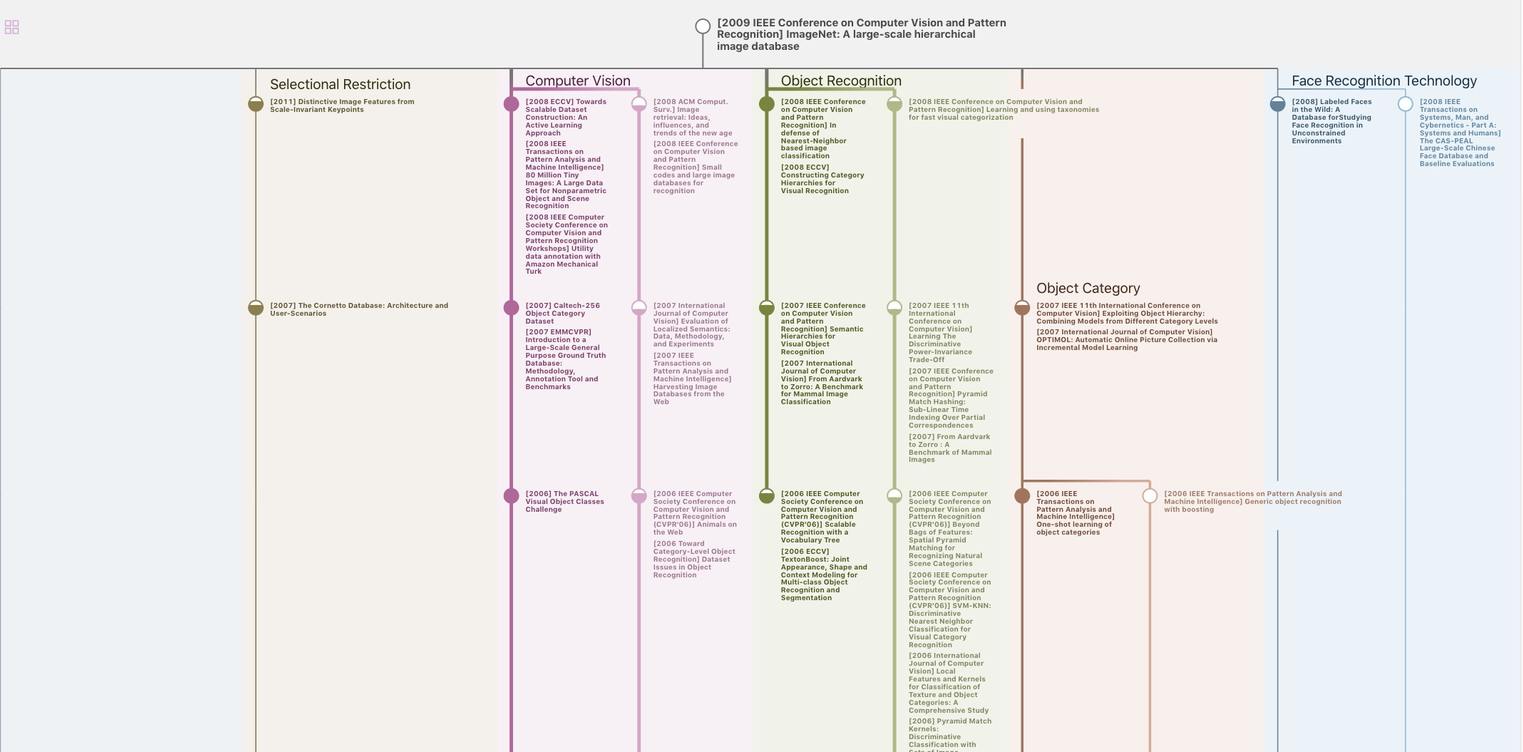
生成溯源树,研究论文发展脉络
Chat Paper
正在生成论文摘要