Faster Motif Counting via Succinct Color Coding and Adaptive Sampling
ACM Transactions on Knowledge Discovery from Data(2021)
摘要
AbstractWe address the problem of computing the distribution of induced connected subgraphs, aka graphlets or motifs, in large graphs. The current state-of-the-art algorithms estimate the motif counts via uniform sampling by leveraging the color coding technique by Alon, Yuster, and Zwick. In this work, we extend the applicability of this approach by introducing a set of algorithmic optimizations and techniques that reduce the running time and space usage of color coding and improve the accuracy of the counts. To this end, we first show how to optimize color coding to efficiently build a compact table of a representative subsample of all graphlets in the input graph. For 8-node motifs, we can build such a table in one hour for a graph with 65M nodes and 1.8B edges, which is \(\) times larger than the state of the art. We then introduce a novel adaptive sampling scheme that breaks the “additive error barrier” of uniform sampling, guaranteeing multiplicative approximations instead of just additive ones. This allows us to count not only the most frequent motifs, but also extremely rare ones. For instance, on one graph we accurately count nearly 10.000 distinct 8-node motifs whose relative frequency is so small that uniform sampling would literally take centuries to find them. Our results show that color coding is still the most promising approach to scalable motif counting.
更多查看译文
关键词
Graphlets, motifs, color coding, subgraph counting, graph mining
AI 理解论文
溯源树
样例
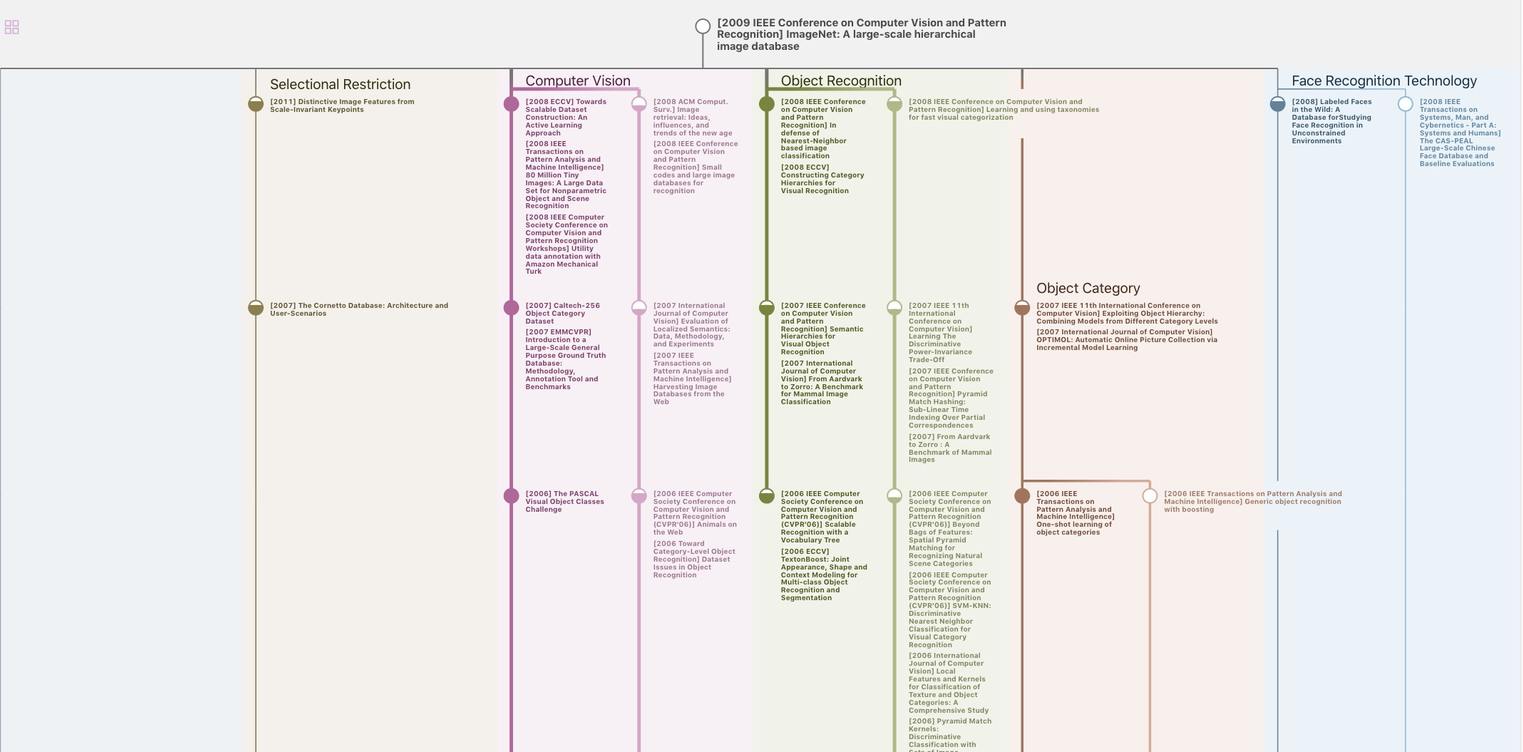
生成溯源树,研究论文发展脉络
Chat Paper
正在生成论文摘要