Sparse Network Inversion For Key Instance Detection In Multiple Instance Learning
2020 25TH INTERNATIONAL CONFERENCE ON PATTERN RECOGNITION (ICPR)(2020)
摘要
Multiple Instance Learning (MIL) involves predicting a single label for a bag of instances, given positive or negative labels at bag-level, without accessing to label for each instance in the training phase. Since a positive bag contains both positive and negative instances, it is often required to detect positive instances (key instances) when a set of instances is categorized as a positive bag. The attention-based deep MIL model is a recent advance in both bag-level classification and key instance detection (KID). However, if the positive and negative instances in a positive bag are not clearly distinguishable, the attention-based deep MIL model has limited KID performance as the attention scores are skewed to few positive instances. In this paper, we present a method to improve the attention-based deep MIL model in the task of KID. The main idea is to use the neural network inversion to find which instances made contribution to the bag-level prediction produced by the trained MIL model. Moreover, we incorporate a sparseness constraint into the neural network inversion, leading to the sparse network inversion which is solved by the proximal gradient method. Numerical experiments on an MNIST-based image MIL dataset and two real-world histopathology datasets verify the validity of our method, demonstrating the KID performance is significantly improved while the performance of bag-level prediction is maintained.
更多查看译文
关键词
sparse network inversion,key instance detection,instance learning,positive labels,negative labels,positive bag,positive instances,negative instances,attention-based deep MIL model,bag-level classification,KID performance,neural network inversion,bag-level prediction,trained MIL model,MNIST-based image MIL dataset,proximal gradient method
AI 理解论文
溯源树
样例
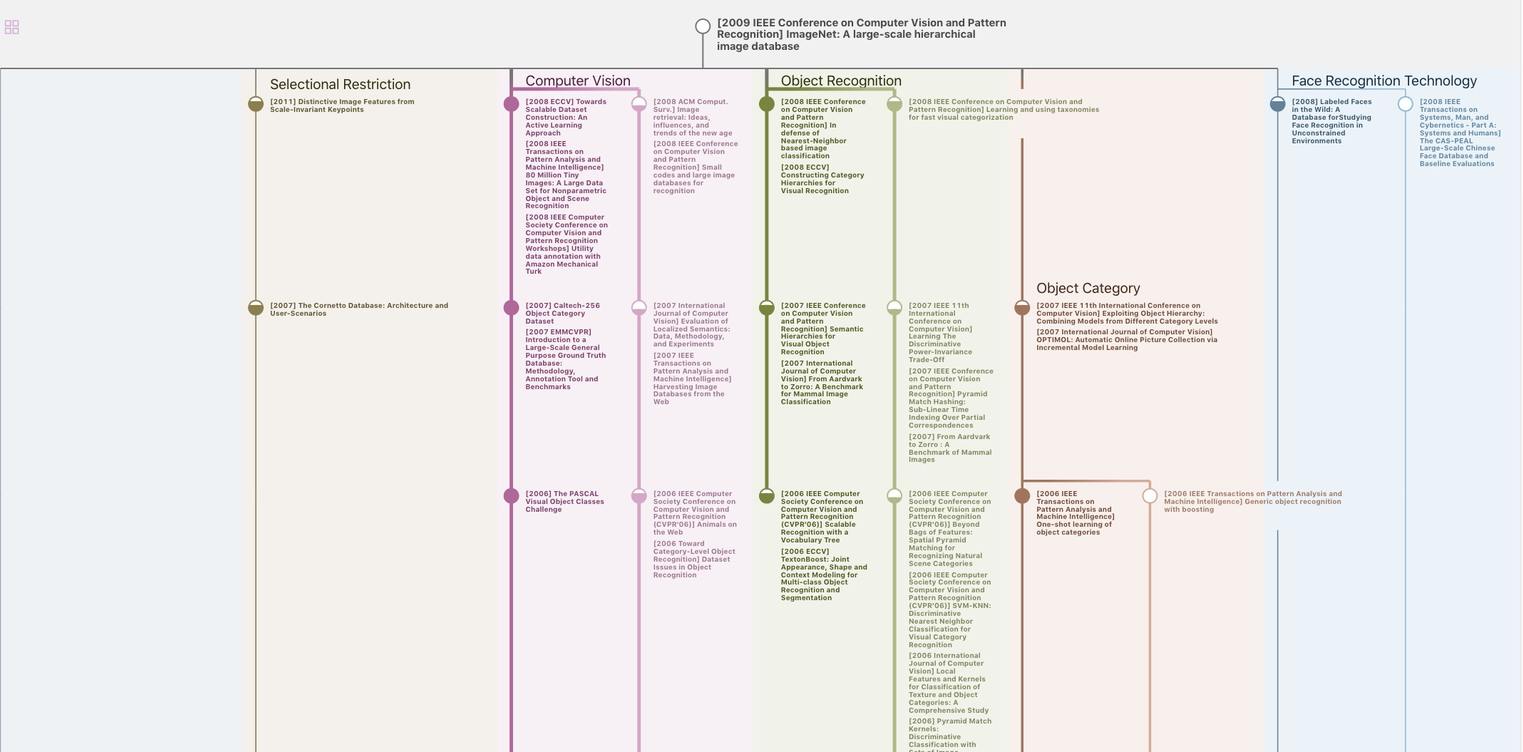
生成溯源树,研究论文发展脉络
Chat Paper
正在生成论文摘要