Adaptive randomization in network data
ELECTRONIC JOURNAL OF STATISTICS(2024)
摘要
Network data have become increasingly prevalent in recent research. For instance, network models have been proposed to improve the quality of estimation and hypothesis testing when comparing the effects of different types of treatment. In this paper, we focus on efficiently estimating the average treatment effect using an adaptive randomization procedure in networks. We are working on models of causal frameworks in which the outcome of a subject's treatment is influenced not only by their own characteristics but also by the characteristics of their neighbors. The experiment's designer does not observe these characteristics. We also consider situations where only the existing subjects' network is revealed when assigning treatments to current subjects. Balancing the connections in the network among different treatment groups can lessen the impact of unknown characteristics. In network data, it is often difficult to obtain theoretical properties because the numbers of nodes and connections increase simultaneously. Under mild assumptions, our proposed procedure is closely related to a time-varying inhomogeneous Markov chain. We utilize the technique of Lyapunov functions to control the moments of the imbalance measure sequence in our proposed approach. As a result, our theoretical analysis demonstrates that our procedure is capable of decreasing the variability of the observed outcome in the model. The advantages of the proposed procedures are also demonstrated by extensive simulations and experiments on real network data.
更多查看译文
关键词
Adaptive randomization design,Lamperti pro-cess,time-varying Markov process,Lyapunov function,network-correlated outcomes
AI 理解论文
溯源树
样例
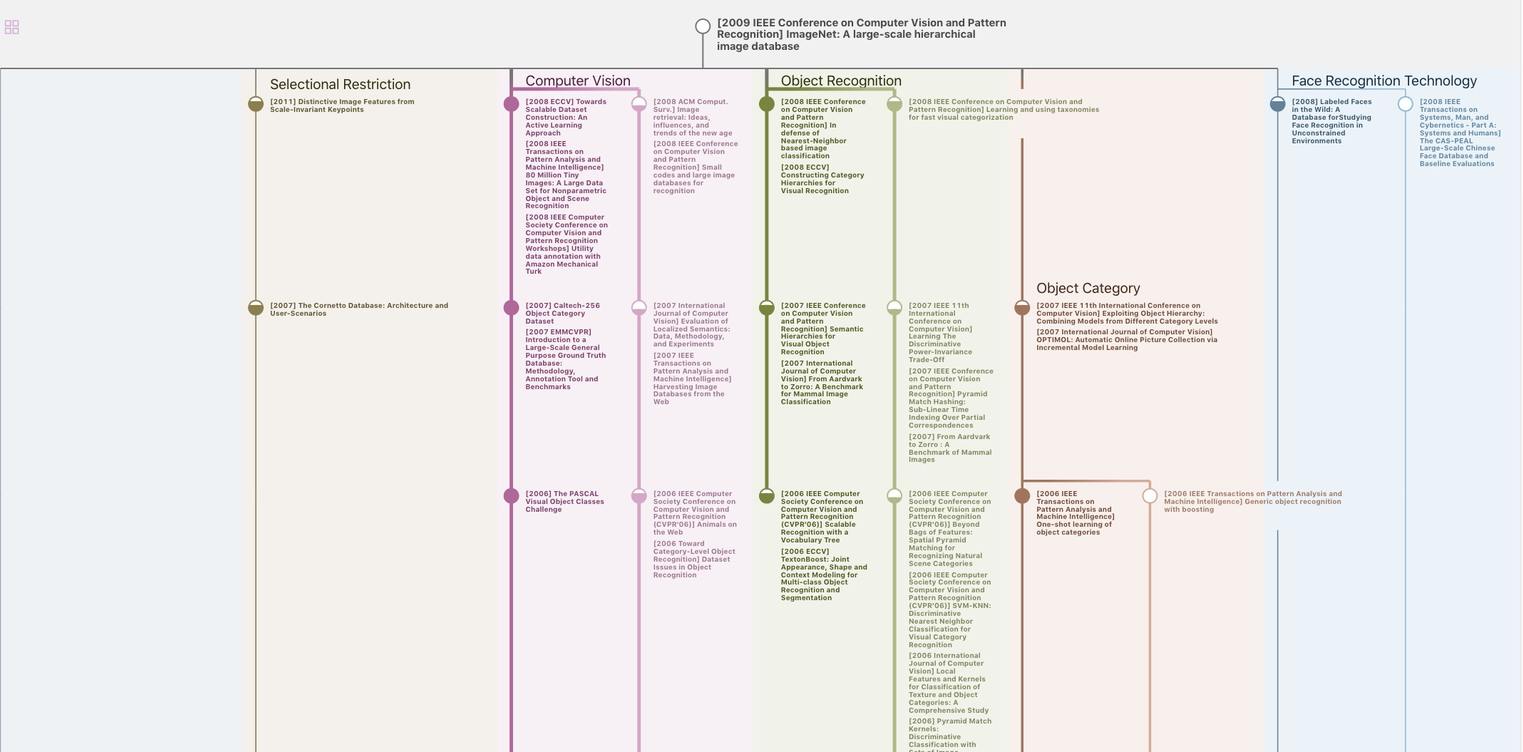
生成溯源树,研究论文发展脉络
Chat Paper
正在生成论文摘要