Fully Automatic Segmentation Of Right And Left Ventricle On Short-Axis Cardiac Mri Images
COMPUTERIZED MEDICAL IMAGING AND GRAPHICS(2020)
摘要
Cardiac magnetic resonance imaging (CMR) is a widely used non-invasive imaging modality for evaluating cardiovascular diseases. CMR is the gold standard method for left and right ventricular functional assessment due to its ability to characterize myocardial structure and function and low intra- and inter-observer variability. However the post-processing segmentation during the functional evaluation is time-consuming and challenging. A fully automated segmentation method can assist the experts; therefore, they can do more efficient work. In this paper, a regression-based fully automated method is presented for the right- and left ventricle segmentation. For training and evaluation, our dataset contained MRI short-axis scans of 5570 patients, who underwent CMR examinations at Heart and Vascular Center, Semmelweis University Budapest. Our approach is novel and after training the state-of-the-art algorithm on our dataset, our algorithm proved to be superior on both of the ventricles. The evaluation metrics were the Dice index, Hausdorff distance and volume related parameters. We have achieved average Dice index for the left endocardium: 0.927, left epicardium: 0.940 and right endocardium: 0.873 on our dataset. We have also compared the performance of the algorithm to the human-level segmentation on both ventricles and it is similar to experienced readers for the left, and comparable for the right ventricle. We also evaluated the proposed algorithm on the ACDC dataset, which is publicly available, with and without transfer learning. The results on ACDC were also satisfying and similar to human observers. Our method is lightweight, fast to train and does not require more than 2 GB GPU memory for execution and training.
更多查看译文
关键词
Deep learning, Cmr, Segmentation, Short-axis image, Image processing
AI 理解论文
溯源树
样例
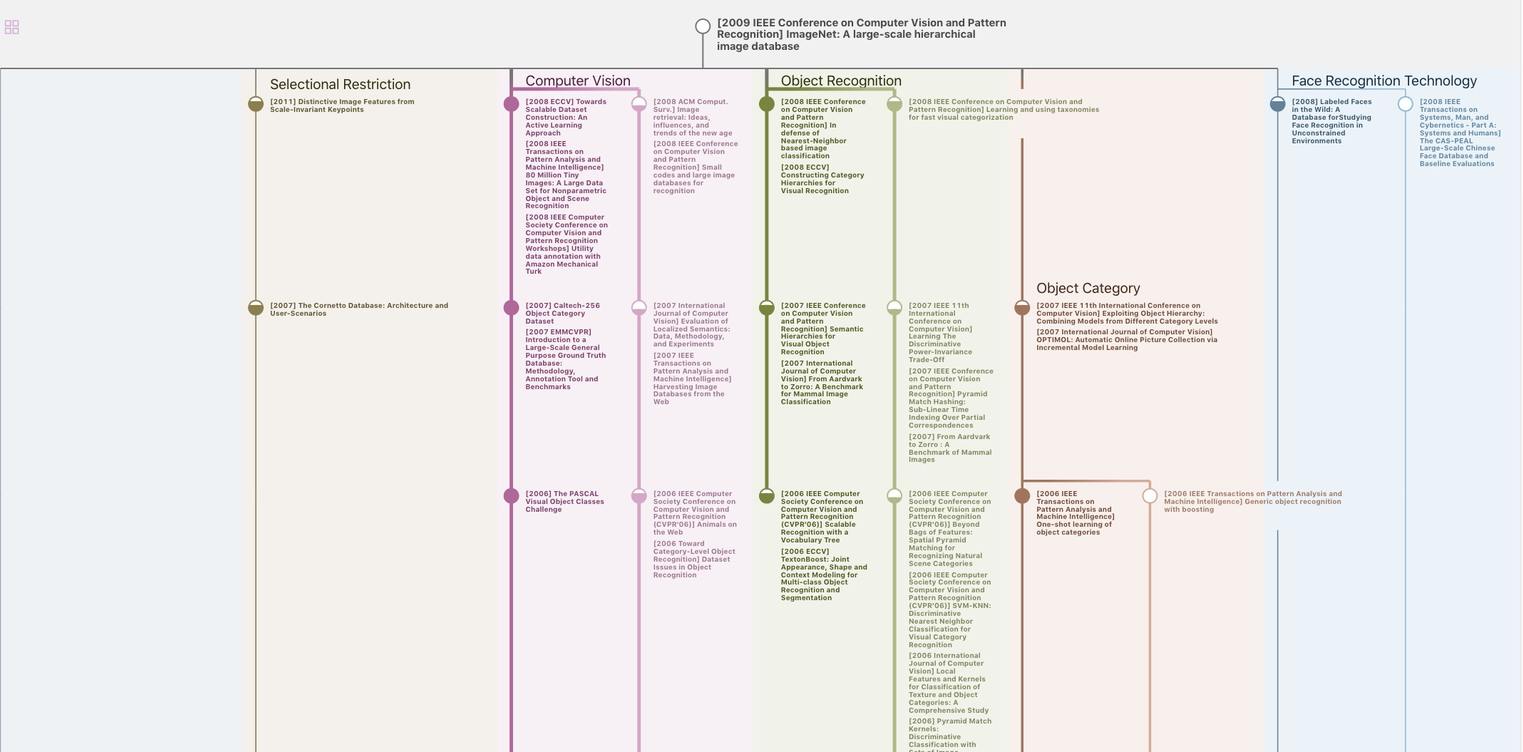
生成溯源树,研究论文发展脉络
Chat Paper
正在生成论文摘要