Tail-Robust Quantile Normalization
PROTEOMICS(2020)
摘要
High-throughput biological data-such as mass spectrometry (MS)-based proteomics data-suffer from systematic non-biological variance due to systematic errors. This hinders the estimation of "real" biological signals and, in turn, decreases the power of statistical tests and biases the identification of differentially expressed proteins. To remove such unintended variation, while retaining the biological signal of interest, analysis workflows for quantitative MS data typically comprise normalization prior to their statistical analysis. Several normalization methods, such as quantile normalization (QN), have originally been developed for microarray data. In contrast to microarray data proteomics data may contain features, in the form of protein intensities that are consistently high across experimental conditions and, hence, are encountered in the tails of the protein intensity distribution. If QN is applied in the presence of such proteins statistical inferences of the features' intensity profiles are impeded due to the biased estimation of their variance. A freely available, novel approach is introduced which serves as an improvement of the classical QN by preserving the biological signals of features in the tails of the intensity distribution and by accounting for sample-dependent missing values (MVs): The "tail-robust quantile normalization" (TRQN).
更多查看译文
关键词
missing values, normalization, PRIDE, proteomics, rank invariance
AI 理解论文
溯源树
样例
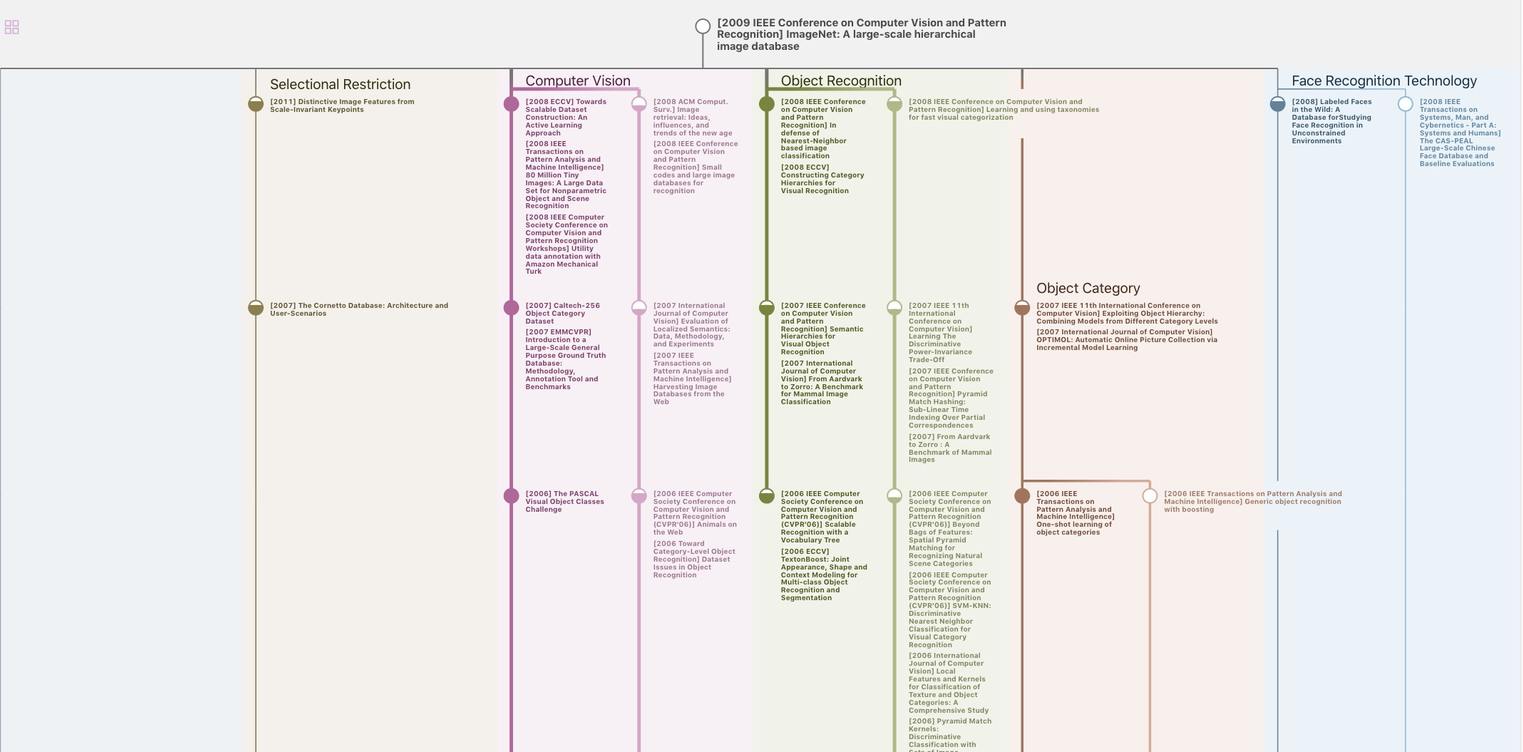
生成溯源树,研究论文发展脉络
Chat Paper
正在生成论文摘要