Computational Learning Theory
user-5ebe28444c775eda72abcdcf(2015)
摘要
Page 1. Computational Learning Theory Mark Craven Computer Sciences 760 Fall 2015 www.biostat.wisc.edu/~craven/cs760/ Some of the slides in these lectures have been adapted/borrowed from materials developed by Tom Dietterich, Pedro Domingos, Tom Mitchell, David Page, and Jude Shavlik Goals for the lecture you should understand the following concepts • PAC learnability • consistent learners and version spaces • sample complexity • PAC learnability in the agnostic setting • the VC dimension • sample complexity using the VC dimension • the on-line learning setting • the mistake bound model of learnability • the Halving algorithm • the Weighted Majority algorithm Page 2. Learning setting #1 instance space X! + + + - set of instances X! • set of hypotheses (models) H • set of possible target concepts C • unknown probability distribution D over instances c ∈C Page 3. Learning setting #1 …
更多查看译文
AI 理解论文
溯源树
样例
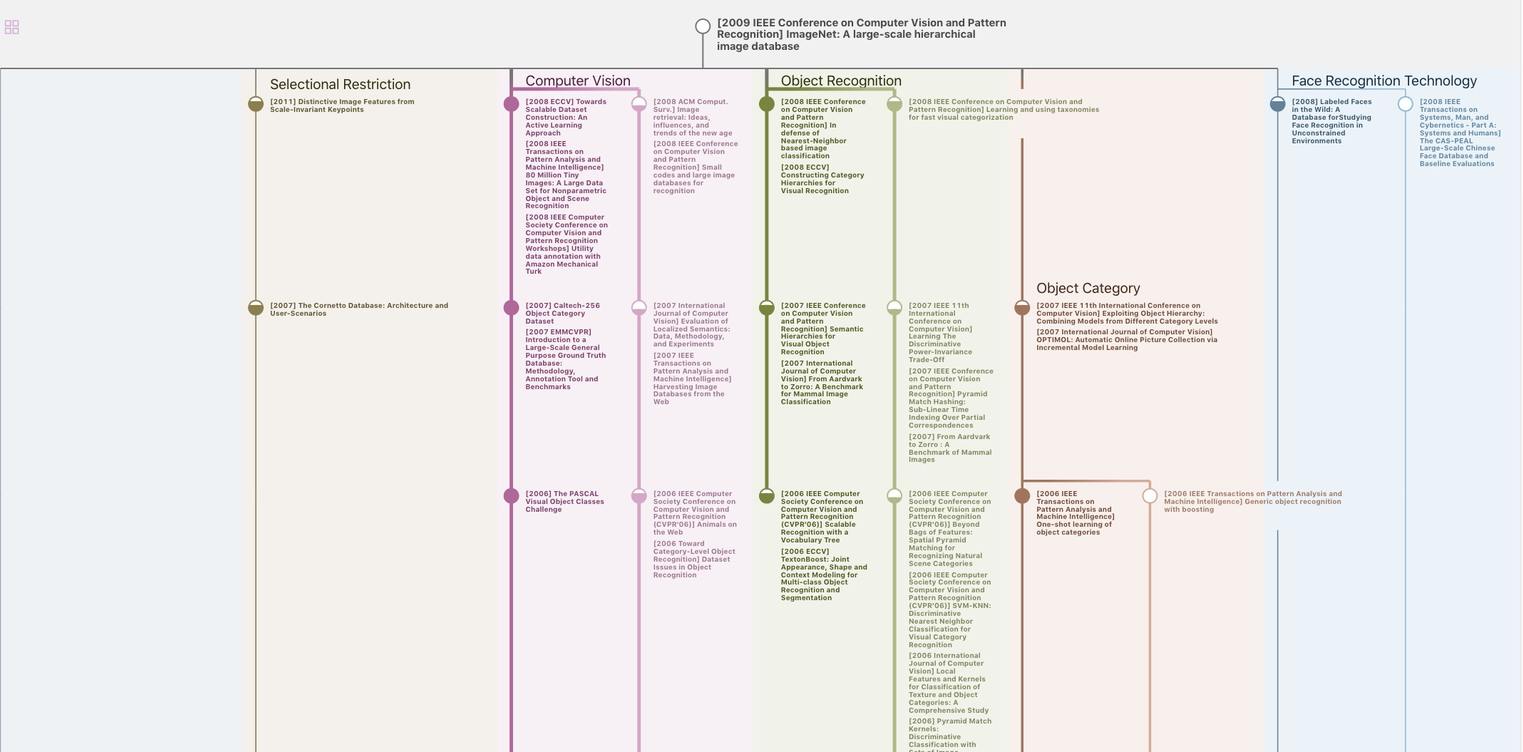
生成溯源树,研究论文发展脉络
Chat Paper
正在生成论文摘要