CAN: A Causal Adversarial Network for Learning Observational and Interventional Distributions
arxiv(2020)
摘要
We propose a generative Causal Adversarial Network (CAN) for learning and sampling from observational (conditional) and interventional distributions. In contrast to the existing CausalGAN which requires the causal graph for the labels to be given, our proposed framework learns the causal relations from the data and generates samples accordingly. In addition to the relationships between labels, our model also learns the label-pixel and pixel-pixel dependencies and incorporate them in sample generation. The proposed CAN comprises a two-fold process namely Label Generation Network (LGN) and Conditional Image Generation Network (CIGN). The LGN is a novel GAN architecture which learns and samples from the causal graph over multi-categorical labels. The sampled labels are then fed to CIGN, a new conditional GAN architecture, which learns the relationships amongst labels and pixels and pixels themselves and generates samples based on them. This framework additionally provides an intervention mechanism which enables the model to generate samples from interventional distributions. We quantitatively and qualitatively assess the performance of CAN and empirically show that our model is able to generate both interventional and observational samples without having access to the causal graph for the application of face generation on CelebA data.
更多查看译文
关键词
causal adversarial network,learning observational,interventional distributions
AI 理解论文
溯源树
样例
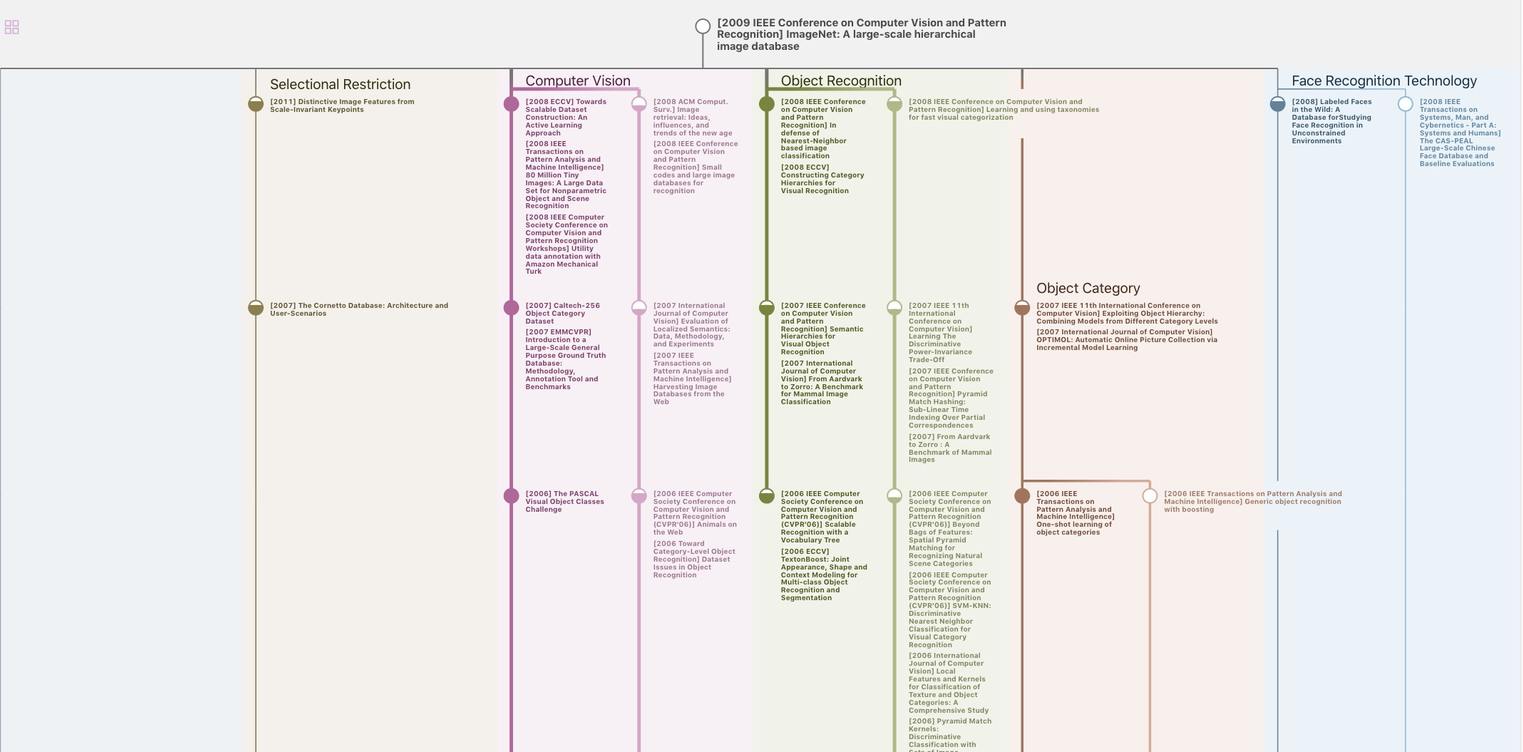
生成溯源树,研究论文发展脉络
Chat Paper
正在生成论文摘要