Learn to Talk via Proactive Knowledge Transfer
arxiv(2020)
摘要
Knowledge Transfer has been applied in solving a wide variety of problems. For example, knowledge can be transferred between tasks (e.g., learning to handle novel situations by leveraging prior knowledge) or between agents (e.g., learning from others without direct experience). Without loss of generality, we relate knowledge transfer to KL-divergence minimization, i.e., matching the (belief) distributions of learners and teachers. The equivalence gives us a new perspective in understanding variants of the KL-divergence by looking at how learners structure their interaction with teachers in order to acquire knowledge. In this paper, we provide an in-depth analysis of KL-divergence minimization in Forward and Backward orders, which shows that learners are reinforced via on-policy learning in Backward. In contrast, learners are supervised in Forward. Moreover, our analysis is gradient-based, so it can be generalized to arbitrary tasks and help to decide which order to minimize given the property of the task. By replacing Forward with Backward in Knowledge Distillation, we observed +0.7-1.1 BLEU gains on the WMT'17 De-En and IWSLT'15 Th-En machine translation tasks.
更多查看译文
关键词
knowledge,transfer
AI 理解论文
溯源树
样例
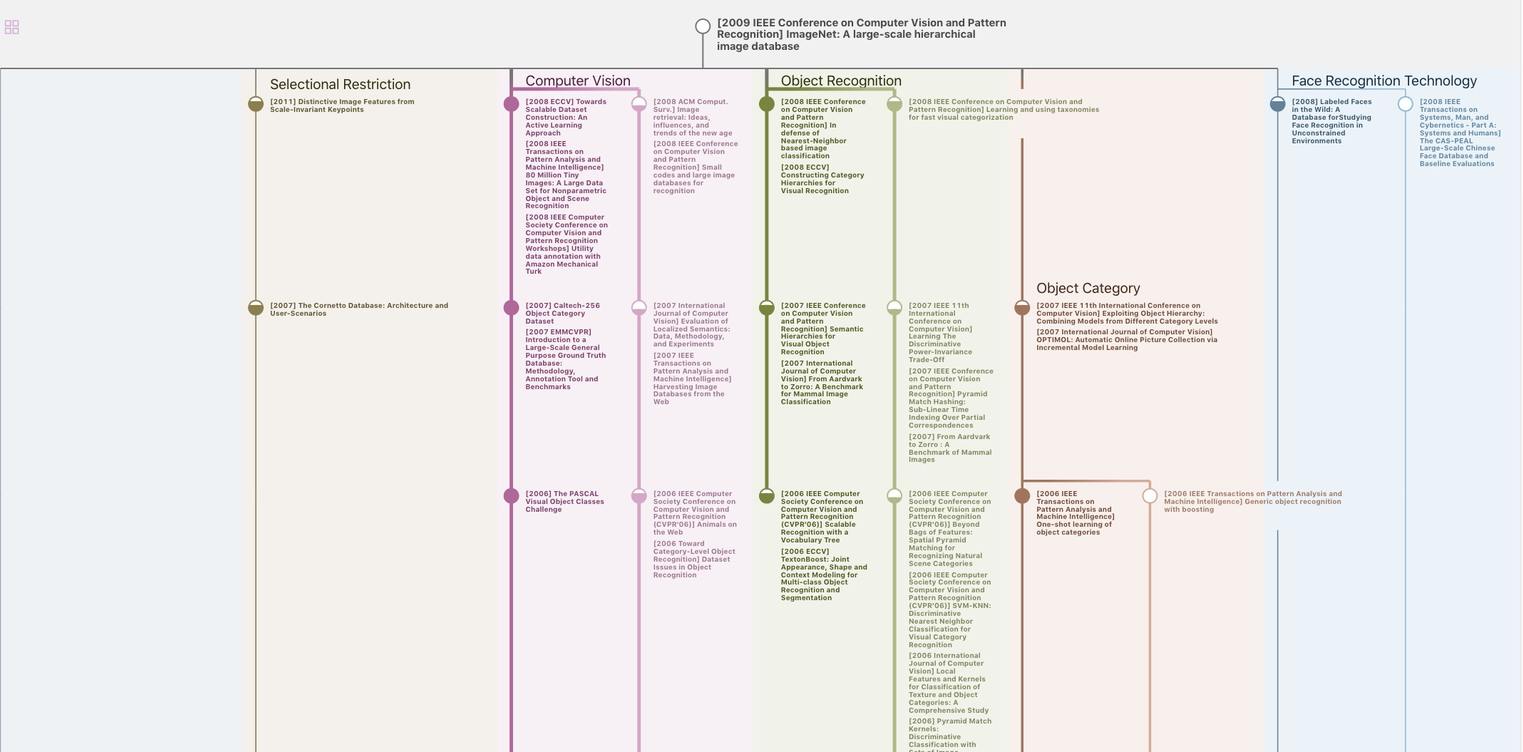
生成溯源树,研究论文发展脉络
Chat Paper
正在生成论文摘要