Deep Learning-Based Signal Strength Prediction Using Geographical Images And Expert Knowledge
2020 IEEE GLOBAL COMMUNICATIONS CONFERENCE (GLOBECOM)(2020)
摘要
Methods for accurate prediction of radio signal quality parameters are crucial for optimization of mobile networks, and a necessity for future autonomous driving solutions. The power-distance relation of current empirical models struggles with describing the specific local geo-statistics that influence signal quality parameters. The use of empirical models commonly results in an over- or under-estimation of the signal quality parameters and require additional calibration studies.In this paper, we present a novel model-aided deep learning approach for path loss prediction, which implicitly extracts radio propagation characteristics from lop-view geographical images of the receiver location. In a comprehensive evaluation campaign, we apply the proposed method on an extensive real-world data set consisting of five different scenarios and more than 125.000 individual measurements.It is found that 1) the novel approach reduces the average prediction error by up to 53 % in comparison to ray-tracing techniques, 2) A distance of 250 - 300 meters spanned by the images offer the necessary level of detail, 3) Predictions with a root-mean-squared error of approximate to 6 dB is achieved across inherently different data sources.
更多查看译文
关键词
signal strength prediction,expert knowledge,geographical images,learning-based
AI 理解论文
溯源树
样例
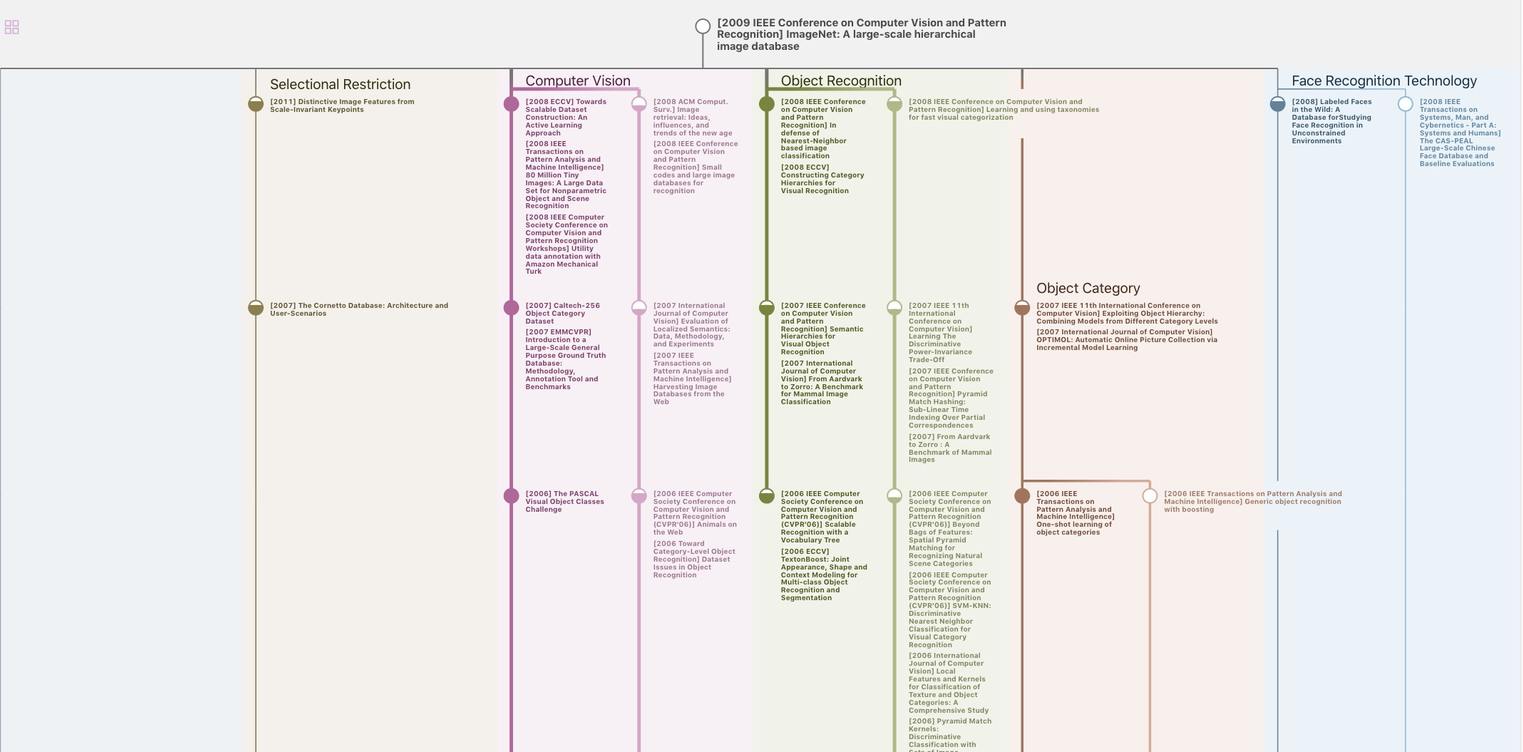
生成溯源树,研究论文发展脉络
Chat Paper
正在生成论文摘要