Iteration-complexity of a proximal augmented Lagrangian method for solving nonconvex composite optimization problems with nonlinear convex constraints
arxiv(2022)
摘要
This paper proposes and analyzes a proximal augmented Lagrangian (NL-IAPIAL) method for solving smooth nonconvex composite optimization problems with nonlinear $\cal K$-convex constraints, i.e., the constraints are convex with respect to the order given by a closed convex cone $\cal K$. Each NL-IAPIAL iteration consists of inexactly solving a proximal augmented Lagrangian subproblem by an accelerated composite gradient (ACG) method followed by a Lagrange multiplier update. Under some mild assumptions, it is shown that NL-IAPIAL generates an approximate stationary solution of the constrained problem in ${\cal O}(\log(1/\rho)/\rho^{3})$ inner iterations, where $\rho>0$ is a given tolerance. Numerical experiments are also given to illustrate the computational efficiency of the proposed method.
更多查看译文
关键词
Primary: 9M05,49M37,90C26,90C30,90C60,65K05,65K10,68Q25,65Y20,inexact proximal augmented Lagrangian method,K-convexity,nonlinear constrained smooth nonconvex composite programming,accelerated first-order methods,iteration complexity
AI 理解论文
溯源树
样例
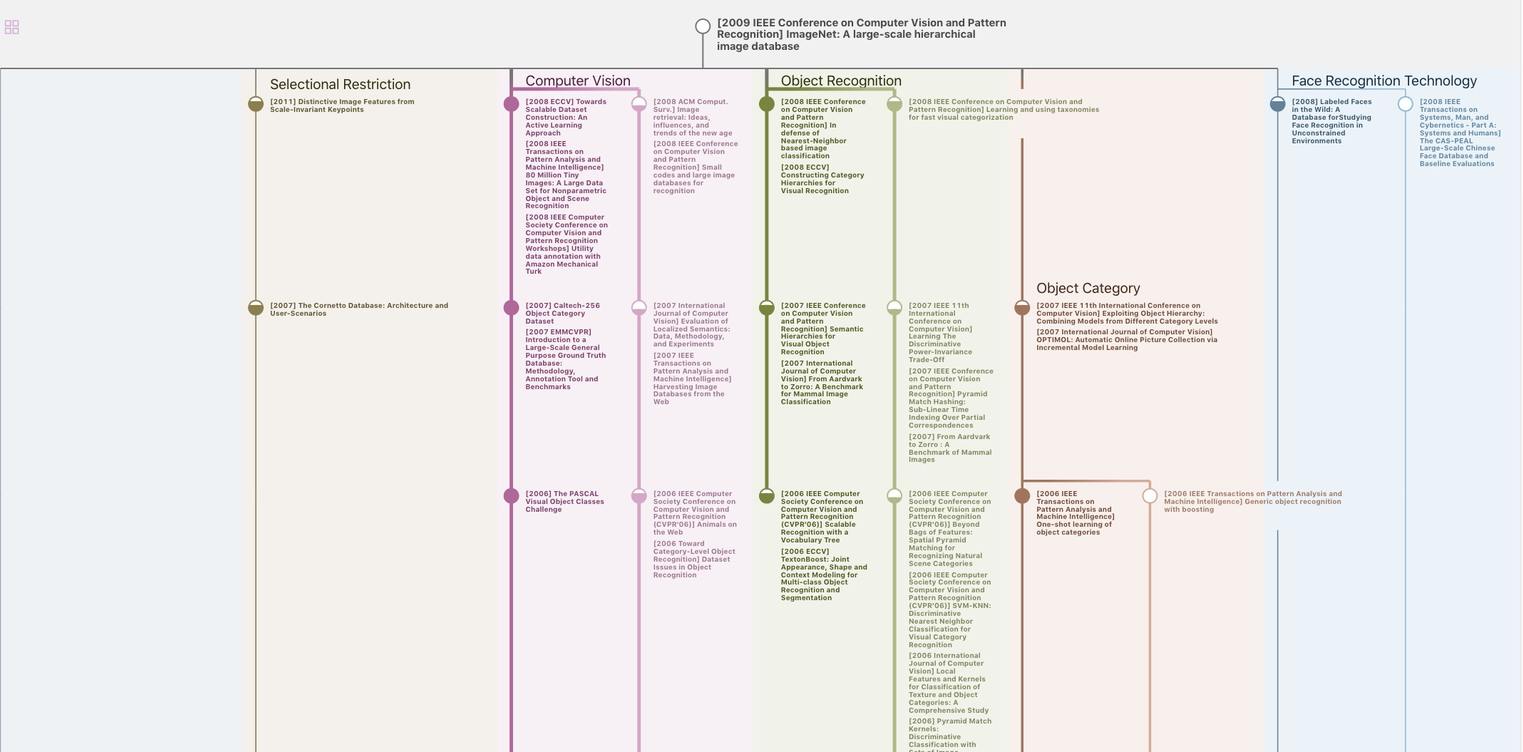
生成溯源树,研究论文发展脉络
Chat Paper
正在生成论文摘要