Exact and arbitrarily accurate non-parametric two-sample tests based on rank spacings
arxiv(2022)
摘要
A common method for deriving non-parametric tests is to reformulate a parametric test in terms of sample ranks. Despite being distribution free (even in finite samples), the resulting tests often display remarkable asymptotic power properties, typically matching the efficiency of their parametric counterpart. Empirically, these favorable power properties have been shown to persist in non-asymptotic regimes as well, prompting the need for finite-sample characterizations of the corresponding rank-based statistics. Here, we provide such characterization for the family of weighted $p$-norms of rank spacings, which includes the classical tests of Mann-Whitney, Dixon, and various generalizations thereof. For $p=1$, we provide exact expressions for the involved distributions, while for $p>1$ we describe the associated moment sequences and derive an algorithm to recover the distributions of interest from these sequences in a fast and stable manner. We use this framework to develop a new family of non-parametric tests mirroring properties of generalized likelihood-ratios, prove new tail bounds for Dixon's and Greenwood's statistics, and prove a previously formulated conjecture regarding the global efficiency of rank-based tests against the $F$-test in the context of scale-families.
更多查看译文
关键词
rank spacings,tests,non-parametric,two-sample
AI 理解论文
溯源树
样例
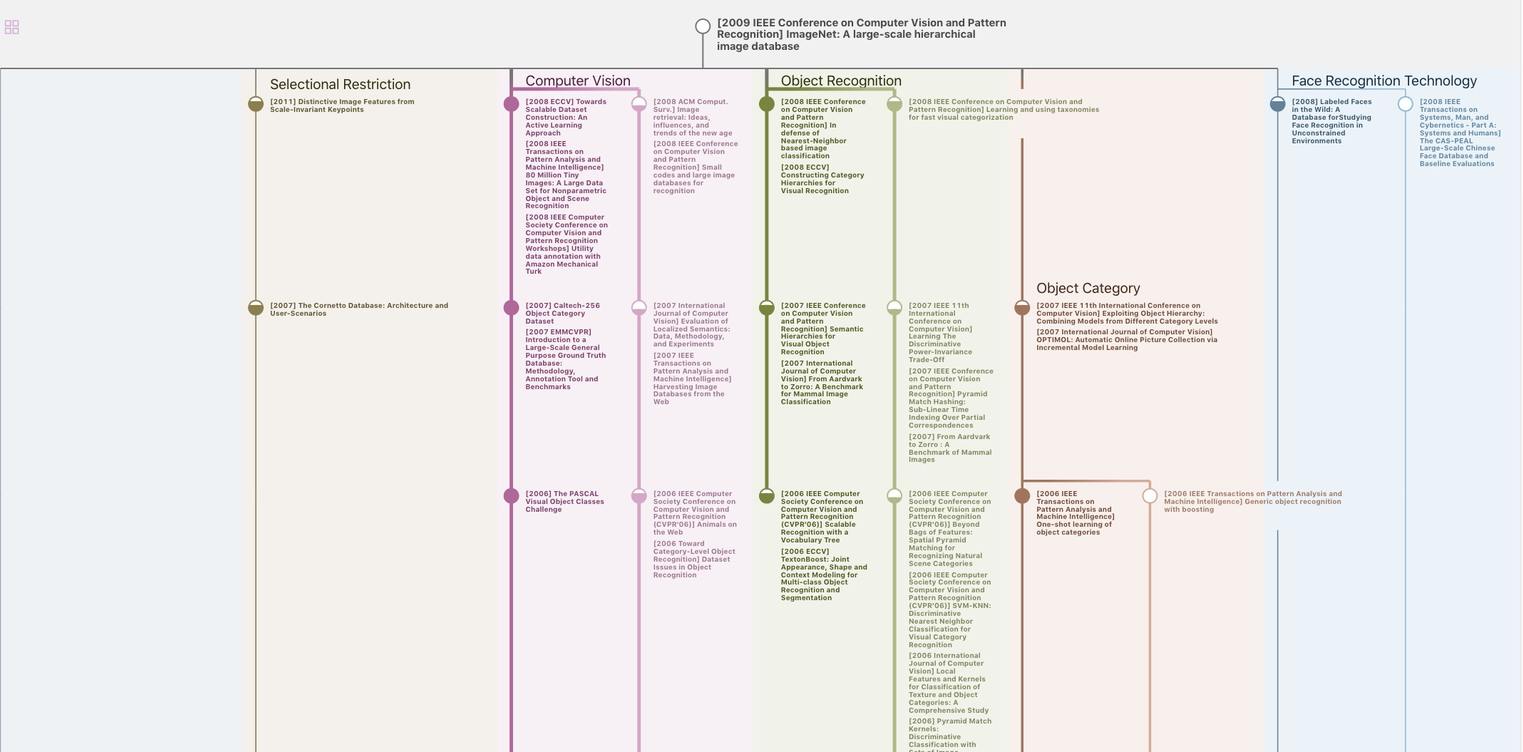
生成溯源树,研究论文发展脉络
Chat Paper
正在生成论文摘要