BREEDS: Benchmarks for Subpopulation Shift
ICLR(2021)
摘要
We develop a methodology for assessing the robustness of models to subpopulation shift---specifically, their ability to generalize to novel data subpopulations that were not observed during training. Our approach leverages the class structure underlying existing datasets to control the data subpopulations that comprise the training and test distributions. This enables us to synthesize realistic distribution shifts whose sources can be precisely controlled and characterized, within existing large-scale datasets. Applying this methodology to the ImageNet dataset, we create a suite of subpopulation shift benchmarks of varying granularity. We then validate that the corresponding shifts are tractable by obtaining human baselines for them. Finally, we utilize these benchmarks to measure the sensitivity of standard model architectures as well as the effectiveness of off-the-shelf train-time robustness interventions.
更多查看译文
关键词
subpopulation shift,benchmarks
AI 理解论文
溯源树
样例
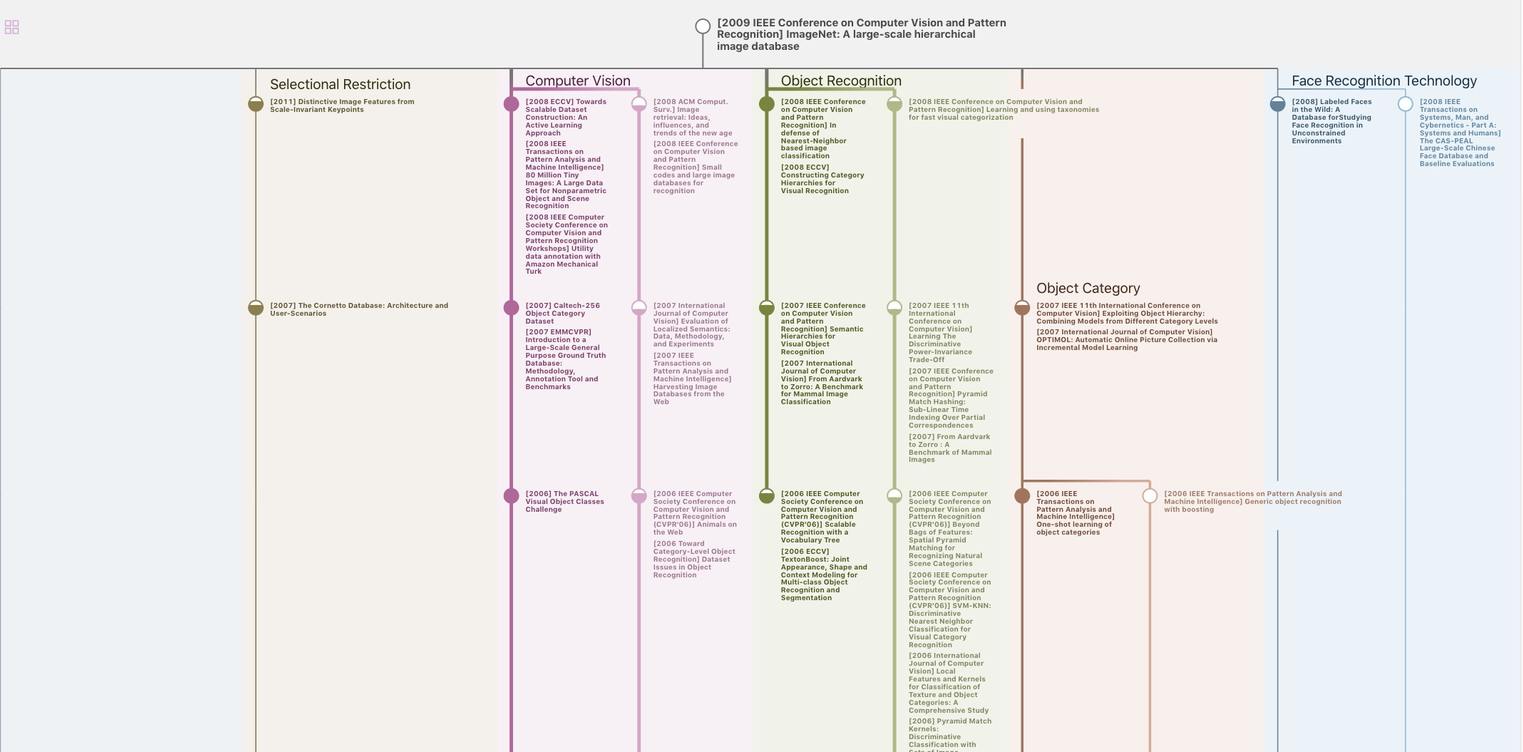
生成溯源树,研究论文发展脉络
Chat Paper
正在生成论文摘要