Distance-Weighted Graph Neural Networks On Fpgas For Real-Time Particle Reconstruction In High Energy Physics
FRONTIERS IN BIG DATA(2021)
摘要
Graph neural networks have been shown to achieve excellent performance for several crucial tasks in particle physics, such as charged particle tracking, jet tagging, and clustering. An important domain for the application of these networks is the FGPA-based first layer of real-time data filtering at the CERN Large Hadron Collider, which has strict latency and resource constraints. We discuss how to design distance-weighted graph networks that can be executed with a latency of less than one mu s on an FPGA. To do so, we consider a representative task associated to particle reconstruction and identification in a next-generation calorimeter operating at a particle collider. We use a graph network architecture developed for such purposes, and apply additional simplifications to match the computing constraints of Level-1 trigger systems, including weight quantization. Using the hls4ml library, we convert the compressed models into firmware to be implemented on an FPGA. Performance of the synthesized models is presented both in terms of inference accuracy and resource usage.
更多查看译文
关键词
deep learning, field-programmable gate arrays, fast inference, graph network, imaging calorimeter
AI 理解论文
溯源树
样例
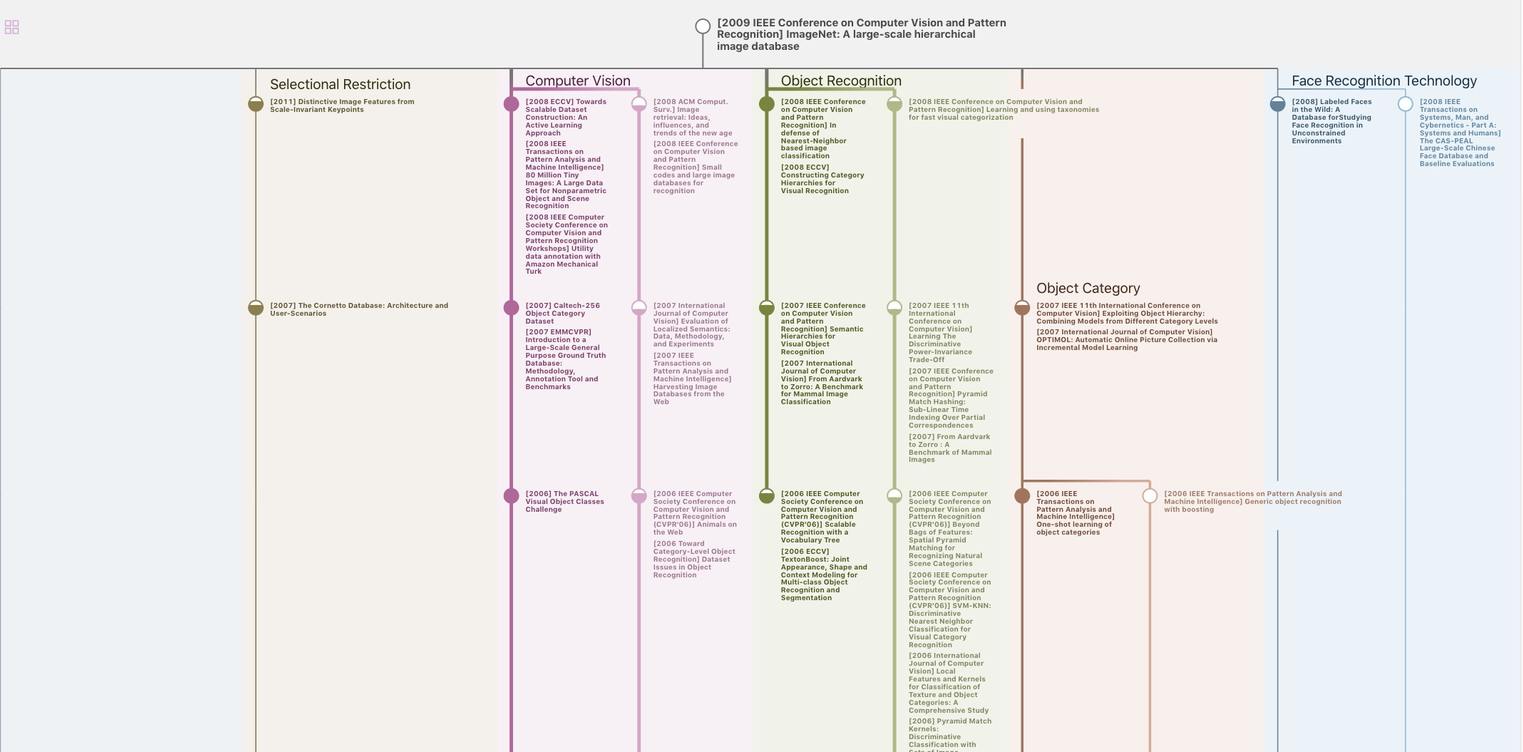
生成溯源树,研究论文发展脉络
Chat Paper
正在生成论文摘要