Parallelizing MCMC sampling via space partitioning
Statistics and Computing(2022)
摘要
Efficient sampling of many-dimensional and multimodal density functions is a task of great interest in many research fields. We describe an algorithm that allows parallelizing inherently serial Markov chain Monte Carlo (MCMC) sampling by partitioning the space of the function parameters into multiple subspaces and sampling each of them independently. The samples of the different subspaces are then reweighted by their integral values and stitched back together. This approach allows reducing sampling wall-clock time by parallel operation. It also improves sampling of multimodal target densities and results in less correlated samples. Finally, the approach yields an estimate of the integral of the target density function.
更多查看译文
关键词
MCMC Parallelization, Probability and statistics, Integral estimation
AI 理解论文
溯源树
样例
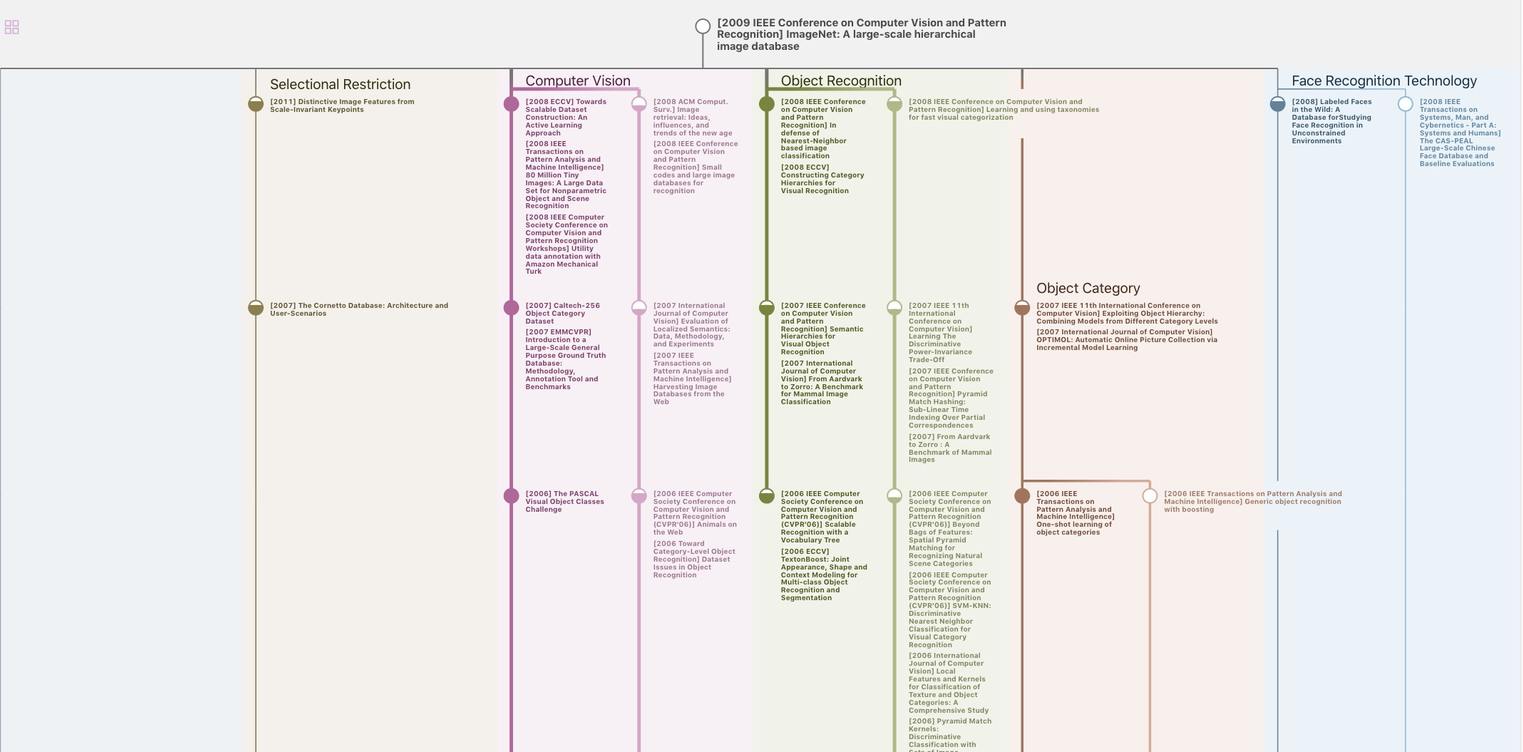
生成溯源树,研究论文发展脉络
Chat Paper
正在生成论文摘要