Plug-And-Pipeline: Efficient Regularization for Single-Step Adversarial Training
2020 IEEE/CVF Conference on Computer Vision and Pattern Recognition Workshops (CVPRW)(2020)
摘要
Adversarial Training (AT) is a straight forward solution to learn robust models by augmenting the training mini-batches with adversarial samples. Adversarial attack methods range from simple non-iterative (single-step) methods to computationally complex iterative (multi-step) methods. Although the single-step methods are efficient, the models trained using these methods merely appear to be robust, due to the masked gradients. In this work, we propose a novel regularizer named Plug-And-Pipeline (PAP) for single-step AT. The proposed regularizer attenuates the gradient masking effect by promoting the model to learn similar representations for both single-step and multi-step adversaries. Further, we present a novel pipelined approach that allows an efficient implementation of the proposed regularizer. Plug-And-Pipeline yields robustness comparable to multi-step AT methods, while requiring a low computational overhead, similar to that of single-step AT methods.
更多查看译文
关键词
straight forward solution,single-step adversarial training,efficient regularization,pipelined approach,multistep adversaries,gradient masking effect,masked gradients,single-step methods,computationally complex iterative,adversarial attack methods,adversarial samples,training mini-batches
AI 理解论文
溯源树
样例
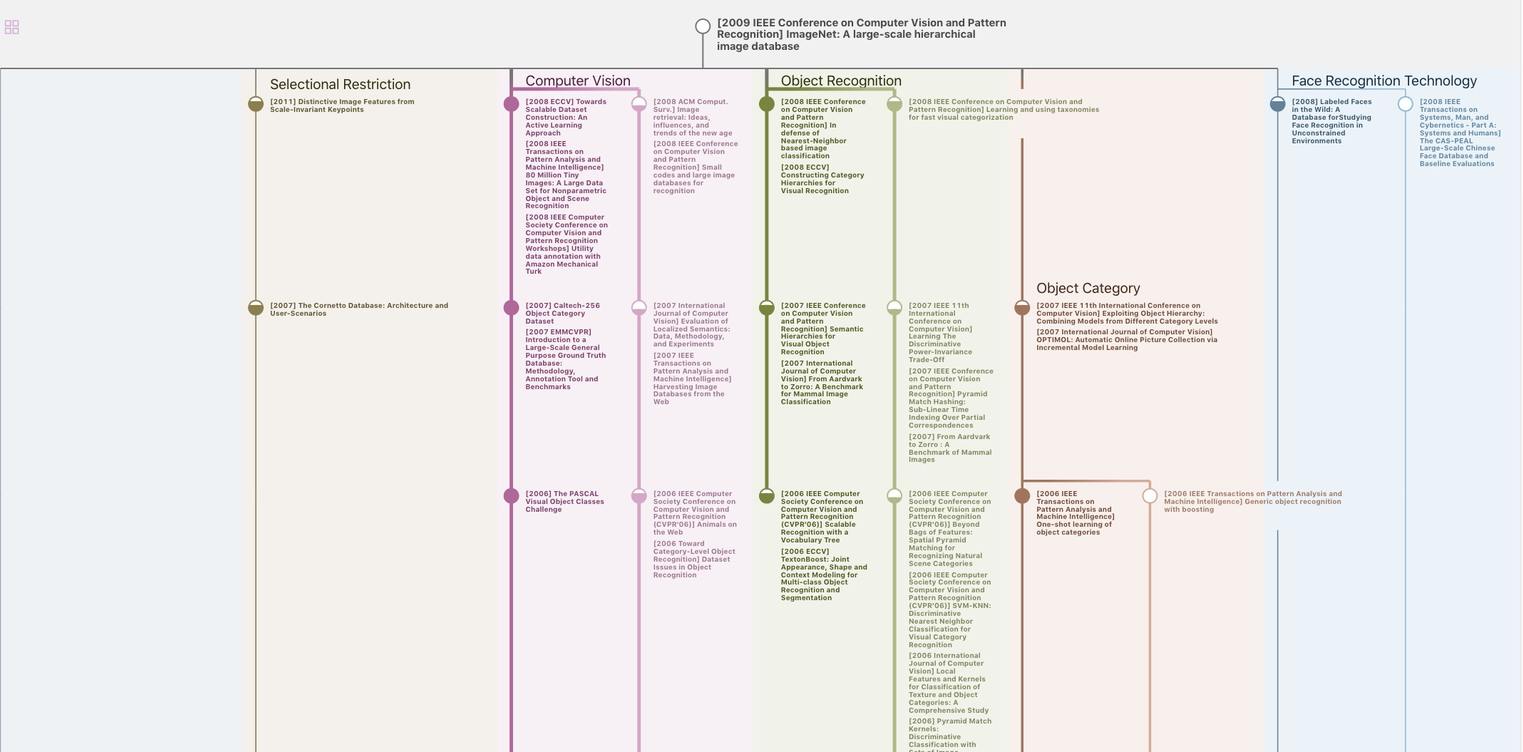
生成溯源树,研究论文发展脉络
Chat Paper
正在生成论文摘要