Monte Carlo Gradient Quantization
CVPR Workshops(2020)
摘要
We propose Monte Carlo methods to leverage both sparsity and quantization to compress gradients of neural networks throughout training. On top of reducing the communication exchanged between multiple workers in a distributed setting, we also improve the computational efficiency of each worker. Our method, called Monte Carlo Gradient Quantization (MCGQ), shows faster convergence and higher performance than existing quantization methods on image classification and language modeling. Using both low-bit-width-quantization and high sparsity levels, our method more than doubles the rates of existing compression methods from 200× to 520× and 462× to more than 1200× on different language modeling tasks.
更多查看译文
关键词
compression methods,high sparsity levels,low-bit-width-quantization,language modeling,image classification,quantization methods,Monte Carlo gradient quantization,computational efficiency,distributed setting,multiple workers,neural networks,Monte Carlo methods
AI 理解论文
溯源树
样例
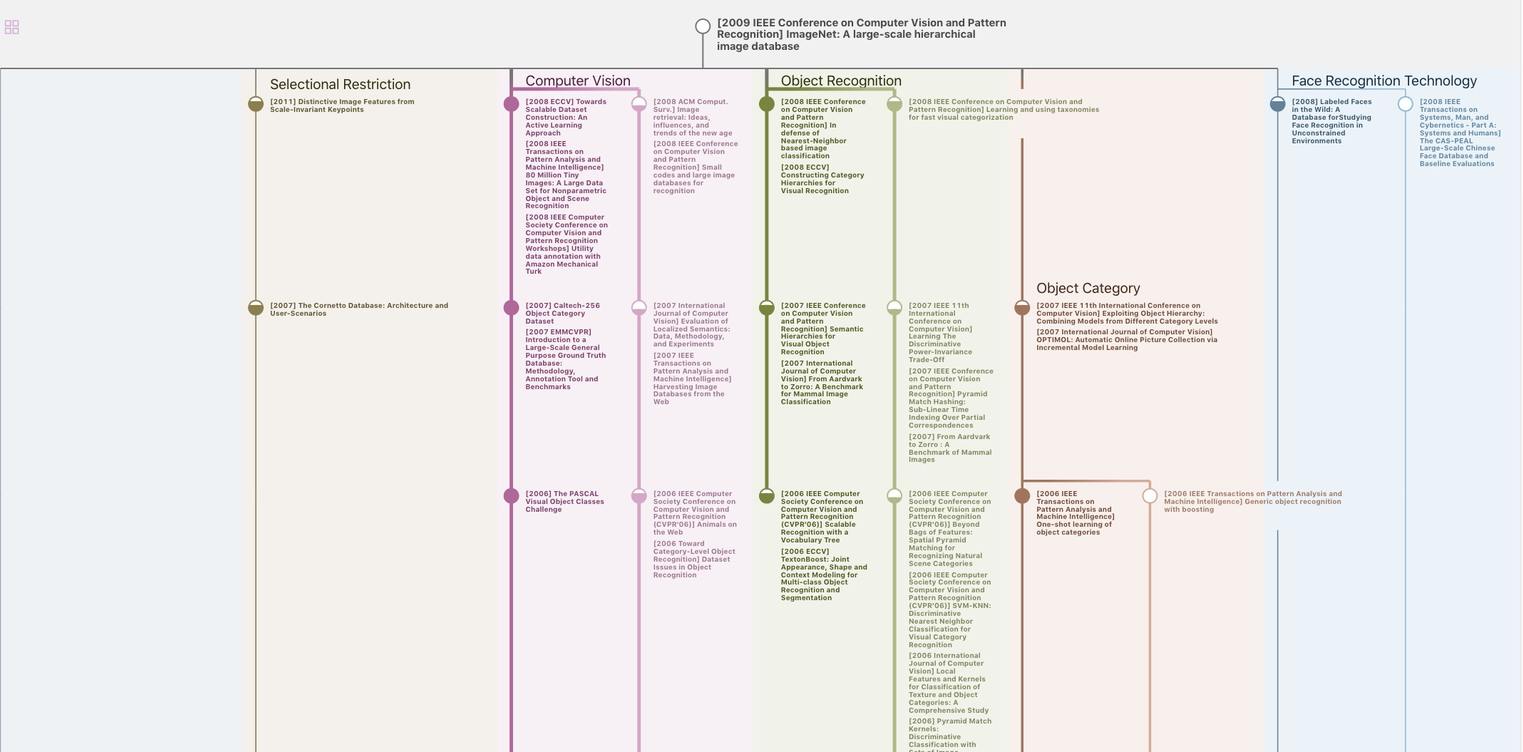
生成溯源树,研究论文发展脉络
Chat Paper
正在生成论文摘要