Topometric Imitation Learning For Route Following Under Appearance Change.
CVPR Workshops(2020)
摘要
Traditional navigation models in autonomous driving rely heavily on metric maps, which severely limits their application in large scale environments. In this paper, we introduce a two-level navigation architecture that contains a topological-metric memory structure and a deep image-based controller. The hybrid memory extracts visual features at each location point with a deep convolutional neural network, and stores information about local driving commands at each location point based on metric information estimated from ego-motion information. The topological-metric memory is seamlessly integrated with a conditional imitation learning controller through the navigational commands that drives the vehicle between different vertices without collision. We test the whole system in teach-and-repeat experiments in an urban driving simulator. Results show that after being trained in a separate environment, the system could quickly adapt to novel environments with a single teach trial and follow route successively under various illumination and weather conditions.
更多查看译文
关键词
urban driving simulator,topometric imitation learning,traditional navigation models,two-level navigation architecture,topological-metric memory structure,deep image-based controller,visual feature extraction,deep convolutional neural network,local driving commands,metric information,egomotion information,conditional imitation learning controller,navigational commands,teach-and-repeat experiments
AI 理解论文
溯源树
样例
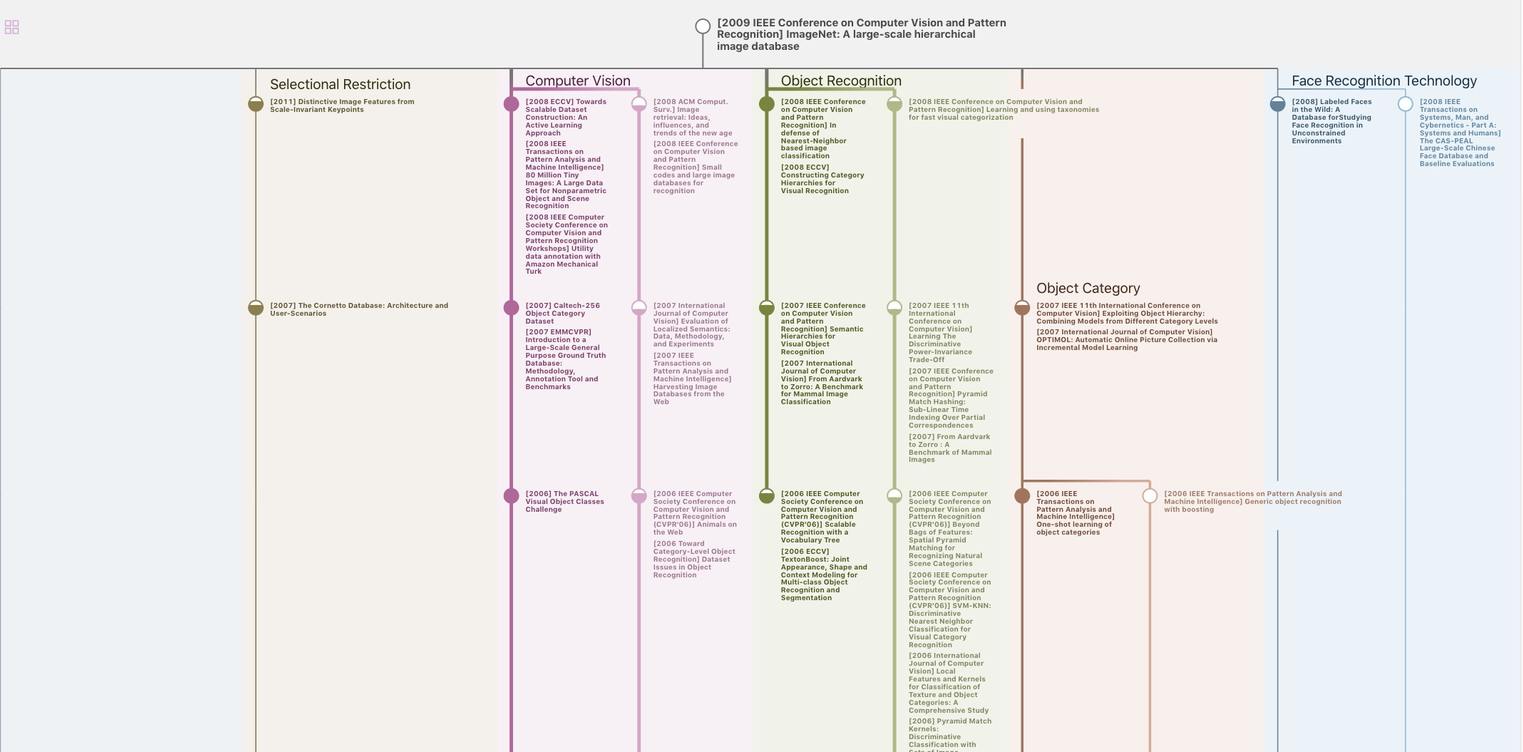
生成溯源树,研究论文发展脉络
Chat Paper
正在生成论文摘要