Evaluation Of Sampling Methods For Scatterplots
IEEE TRANSACTIONS ON VISUALIZATION AND COMPUTER GRAPHICS(2021)
摘要
Given a scatterplot with tens of thousands of points or even more, a natural question is which sampling method should be used to create a small but "good" scatterplot for a better abstraction. We present the results of a user study that investigates the influence of different sampling strategies on multi-class scatterplots. The main goal of this study is to understand the capability of sampling methods in preserving the density, outliers, and overall shape of a scatterplot. To this end, we comprehensively review the literature and select seven typical sampling strategies as well as eight representative datasets. We then design four experiments to understand the performance of different strategies in maintaining: 1) region density; 2) class density; 3) outliers; and 4) overall shape in the sampling results. The results show that: 1) random sampling is preferred for preserving region density; 2) blue noise sampling and random sampling have comparable performance with the three multi-class sampling strategies in preserving class density; 3) outlier biased density based sampling, recursive subdivision based sampling, and blue noise sampling perform the best in keeping outliers; and 4) blue noise sampling outperforms the others in maintaining the overall shape of a scatterplot.
更多查看译文
关键词
Sampling methods, Task analysis, Shape, Data visualization, Visualization, Bibliographies, Scalability, Scatterplot, data sampling, empirical evaluation
AI 理解论文
溯源树
样例
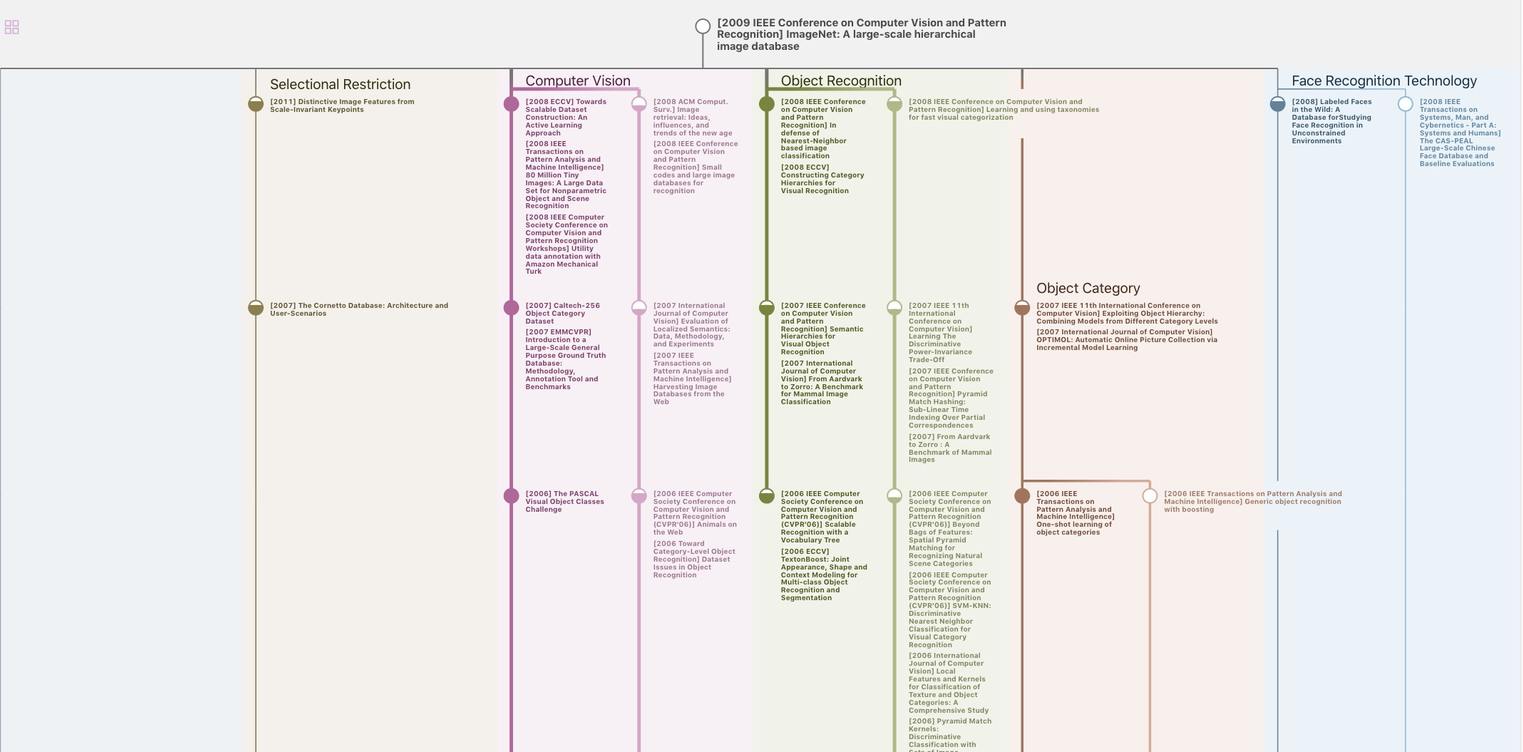
生成溯源树,研究论文发展脉络
Chat Paper
正在生成论文摘要