Uncertainty Quantification Using Generalized Polynomial Chaos for Online Simulations of Automotive Propulsion Systems *
2020 American Control Conference (ACC)(2020)
摘要
Online simulations conducted in vehicles can enable predictive control of automotive systems. This capability can be especially valuable for complex propulsion systems to manage performance, safety, and efficiency under changing drive conditions. Reliable online simulations require accurate models. However, modeling errors are unavoidable, and the inputs from the driver and environment are subject to uncertainty and generally unknown a priori, rendering the system stochastic. Furthermore, limited computing resources in a vehicle can prohibit solving stochastic systems, posing a major challenge. This paper seeks to alleviate these computational bottlenecks by utilizing generalized Polynomial Chaos to efficiently propagate and quantify uncertainty without loss of accuracy for online propulsion system simulations. To demonstrate the effectiveness of this method, uncertainty quantification is performed for simulations of vehicle launch where both model and input uncertainties are considered. A standard Monte Carlo method is used as a baseline for comparison. It is shown that, for the same accuracy, the proposed method is more than two orders of magnitude faster than a Monte Carlo method. A variance-based sensitivity analysis is also used to quantify the statistical contribution from each uncertainty source to the output. The outcome suggests that the proposed method is well-suited to automotive applications where fast and accurate on-board simulation capabilities are required.
更多查看译文
关键词
Torque,Uncertainty,Computational modeling,Propulsion,Engines,Chaos,Automotive engineering
AI 理解论文
溯源树
样例
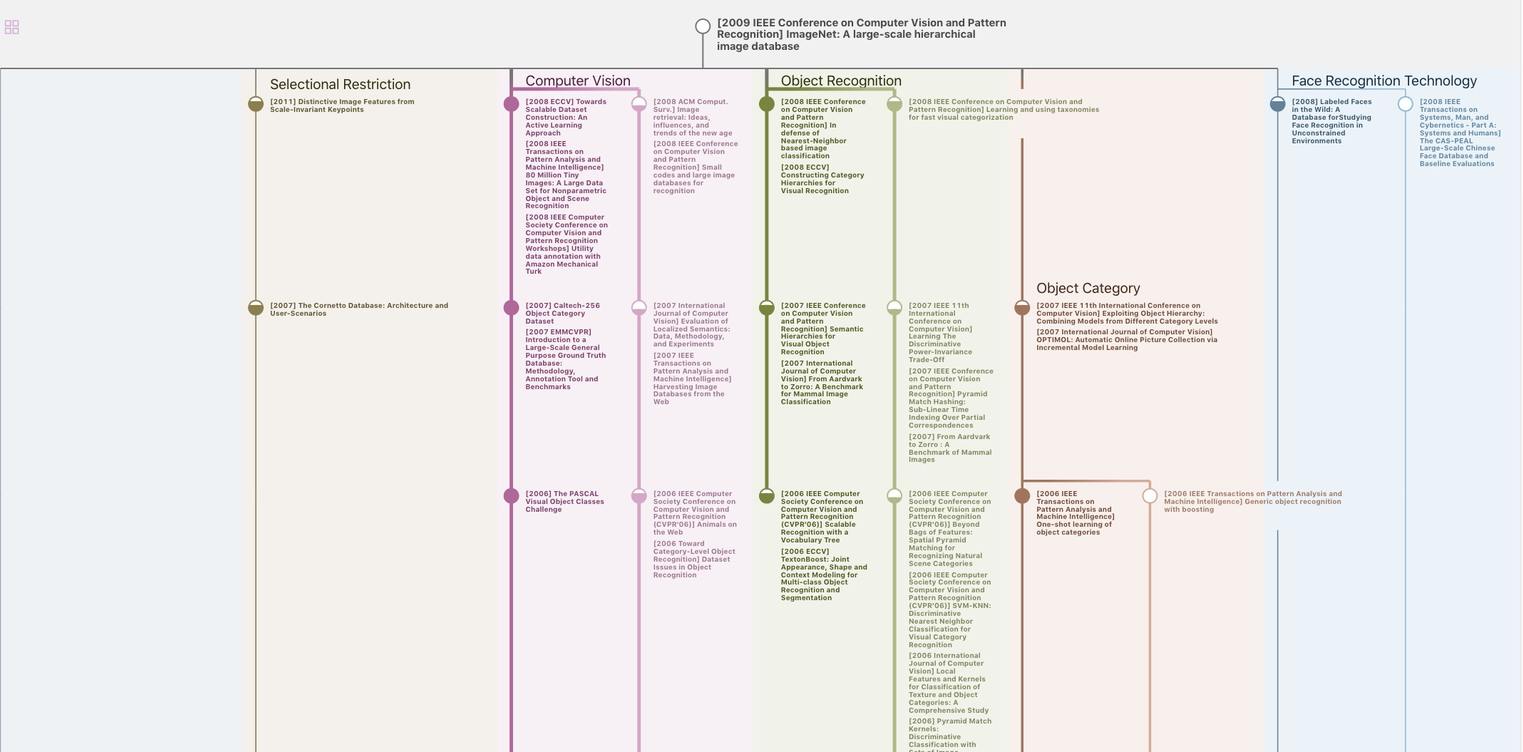
生成溯源树,研究论文发展脉络
Chat Paper
正在生成论文摘要