Learning Compositional Neural Programs for Continuous Control
arxiv(2020)
摘要
We propose a novel solution to challenging sparse-reward, continuous control problems that require hierarchical planning at multiple levels of abstraction. Our solution, dubbed AlphaNPI-X, involves three separate stages of learning. First, we use off-policy reinforcement learning algorithms with experience replay to learn a set of atomic goal-conditioned policies, which can be easily repurposed for many tasks. Second, we learn self-models describing the effect of the atomic policies on the environment. Third, the self-models are harnessed to learn recursive compositional programs with multiple levels of abstraction. The key insight is that the self-models enable planning by imagination, obviating the need for interaction with the world when learning higher-level compositional programs. To accomplish the third stage of learning, we extend the AlphaNPI algorithm, which applies AlphaZero to learn recursive neural programmer-interpreters. We empirically show that AlphaNPI-X can effectively learn to tackle challenging sparse manipulation tasks, such as stacking multiple blocks, where powerful model-free baselines fail.
更多查看译文
关键词
compositional neural programs,control,learning
AI 理解论文
溯源树
样例
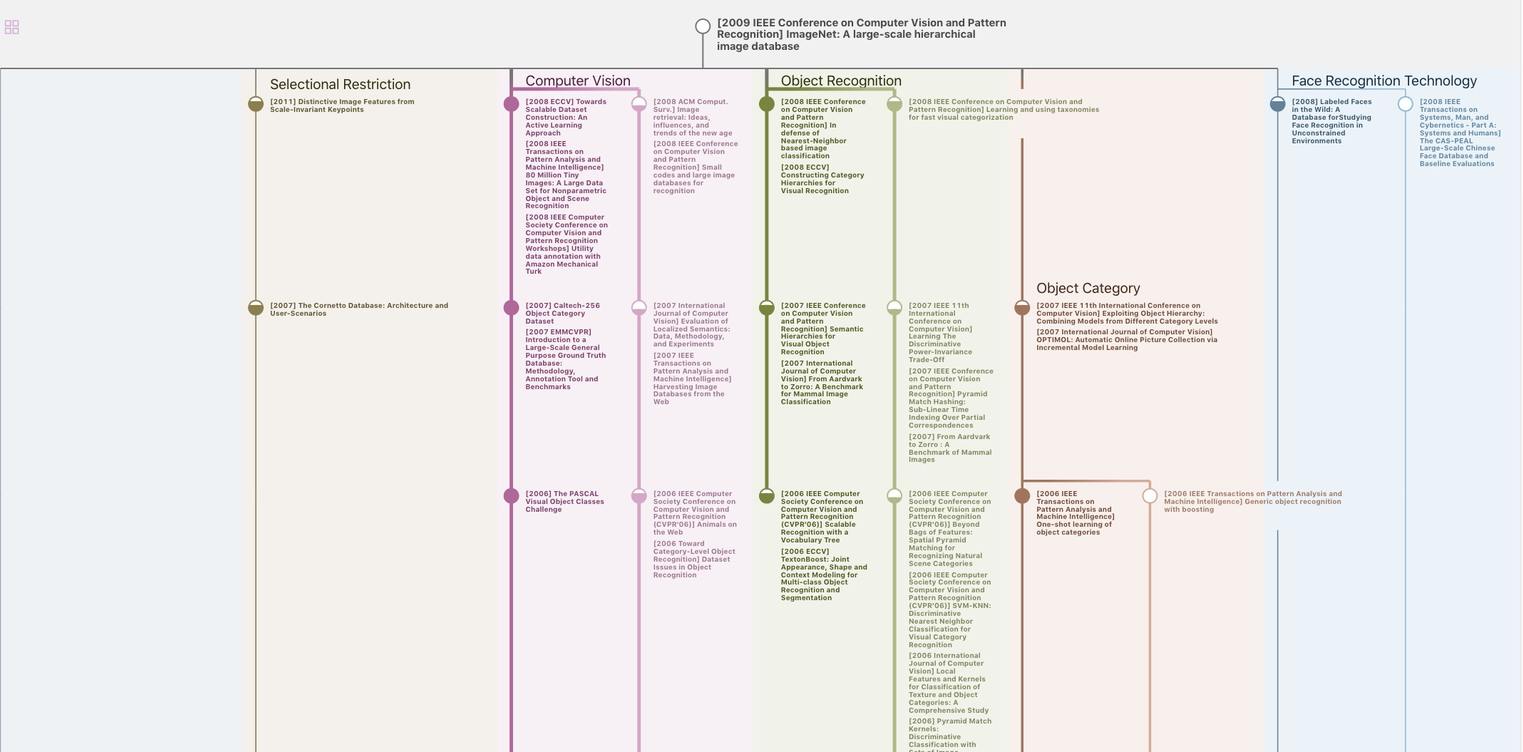
生成溯源树,研究论文发展脉络
Chat Paper
正在生成论文摘要