A parallel evolutionary multiple-try metropolis Markov chain Monte Carlo algorithm for sampling spatial partitions
STATISTICS AND COMPUTING(2021)
摘要
We develop an Evolutionary Markov Chain Monte Carlo (EMCMC) algorithm for sampling spatial partitions that lie within a large, complex, and constrained spatial state space. Our algorithm combines the advantages of evolutionary algorithms (EAs) as optimization heuristics for state space traversal and the theoretical convergence properties of Markov Chain Monte Carlo algorithms for sampling from unknown distributions. Local optimality information that is identified via a directed search by our optimization heuristic is used to adaptively update a Markov chain in a promising direction within the framework of a Multiple-Try Metropolis Markov Chain model that incorporates a generalized Metropolis-Hastings ratio. We further expand the reach of our EMCMC algorithm by harnessing the computational power afforded by massively parallel computing architecture through the integration of a parallel EA framework that guides Markov chains running in parallel.
更多查看译文
关键词
Markov chain Monte Carlo, Evolutionary algorithms, Spatial partitioning
AI 理解论文
溯源树
样例
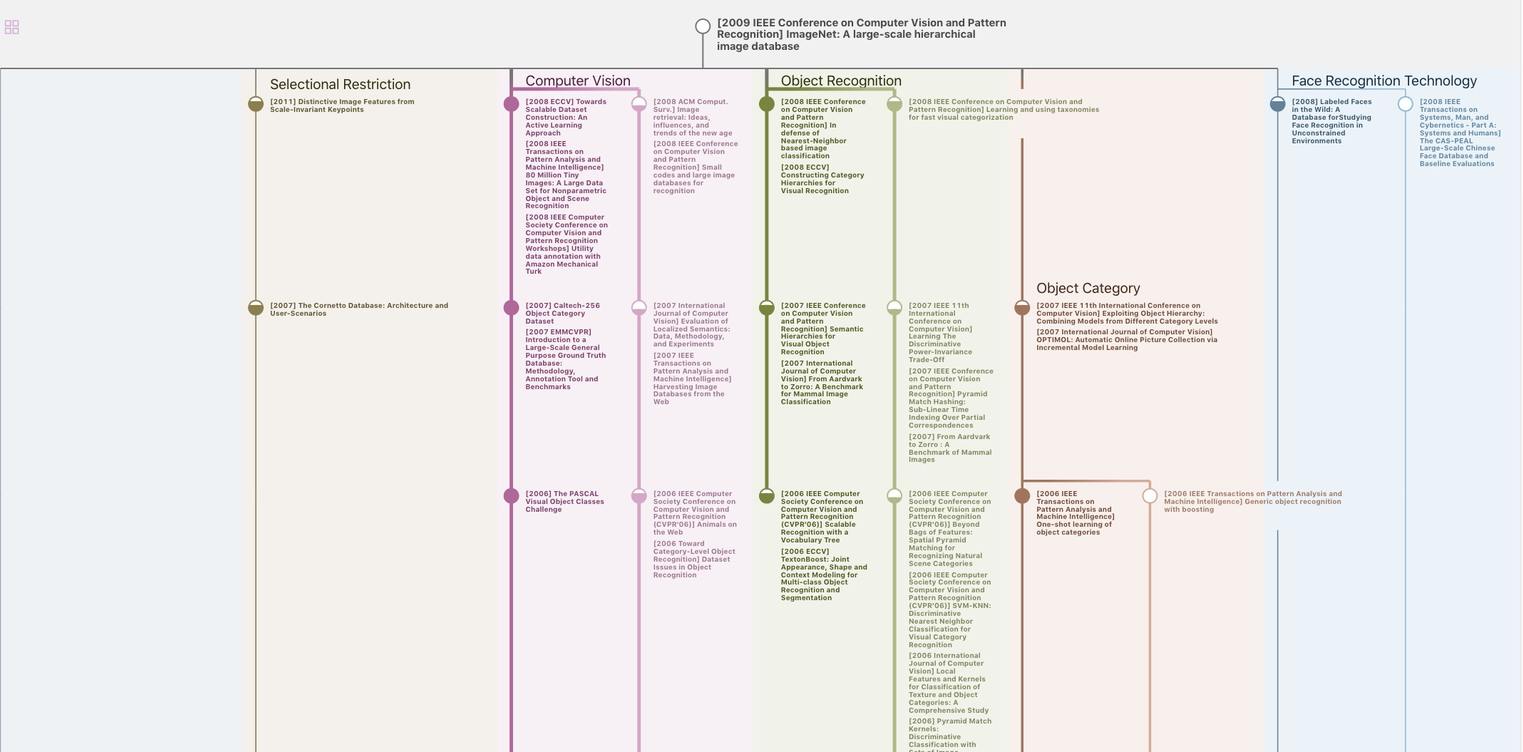
生成溯源树,研究论文发展脉络
Chat Paper
正在生成论文摘要