Machine Learning Based Localization of LoRaWAN Devices via Inter-Technology Knowledge Transfer
2020 IEEE International Conference on Communications Workshops (ICC Workshops)(2020)
摘要
Being able to localize smart devices in Low Power Wide Area Networks (LPWANs) is of primary importance in many Internet of Things applications, including Smart Cities. When GPS positioning is not available, a common strategy is to employ fingerprinting localization, which leverages Received Signal Strength (RSS) radio maps constructed offline during a calibration phase. Often, radio maps can then be interpolated to increase the spatial resolution thus improving localization accuracy. We consider different LPWAN technologies coexisting in the same area, and we explore the possibility of augmenting the localization performance by transferring assistance data for RSS map calibration from one technology to the other. We leverage RSS samples from two real-life LPWANs, namely Wireless M-Bus and LoRaWAN, and we propose several methods for localizing devices through knowledge transfer, comparing them to classical techniques based on simple interpolation within the same technology. Results show that transfer-based approaches are able to improve the localization accuracy up to 12% compared to simple interpolation based on single technology and 16% compared to the case where no interpolation strategy is applied.
更多查看译文
关键词
LoRaWAN,Fingerprinting Localization,Radio Map Interpolation,Transfer Learning
AI 理解论文
溯源树
样例
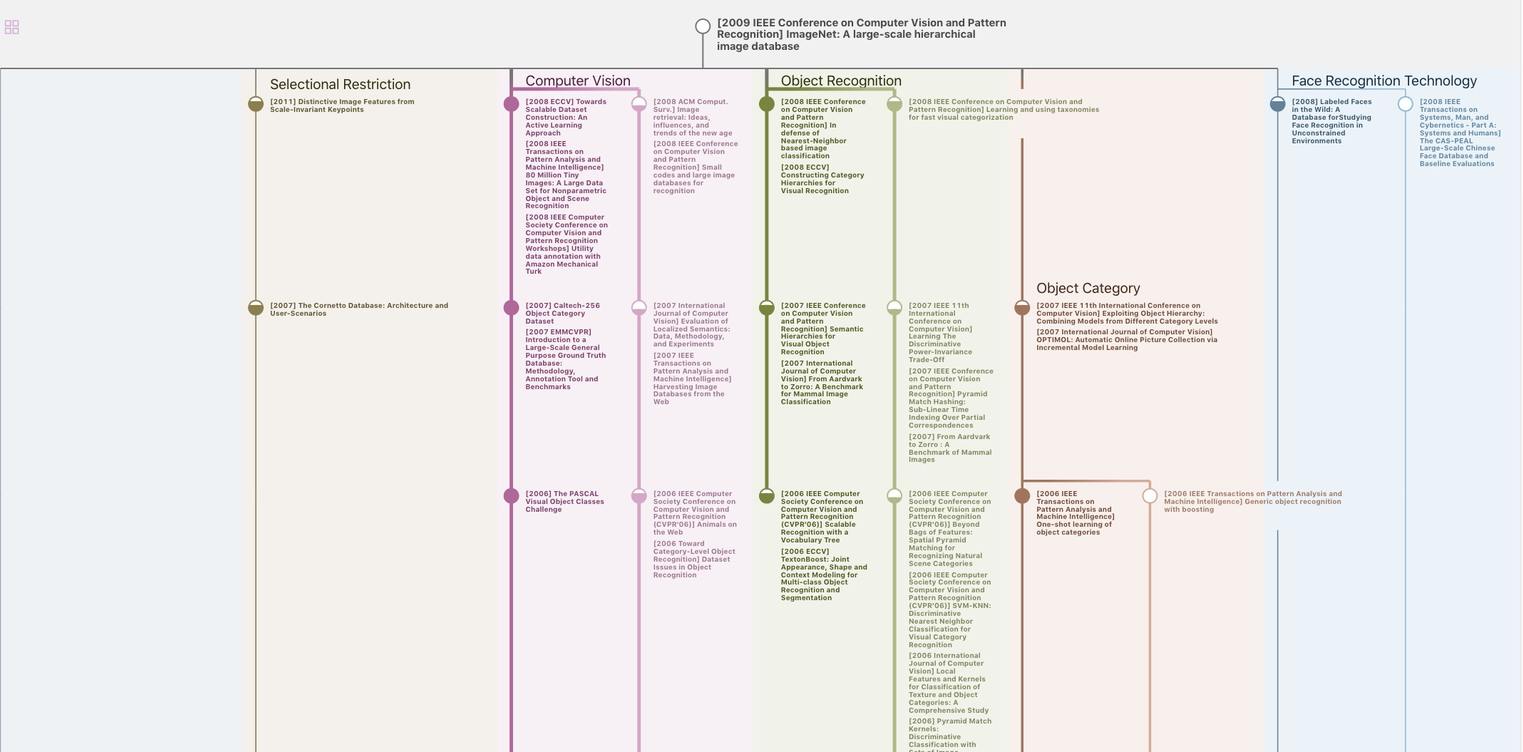
生成溯源树,研究论文发展脉络
Chat Paper
正在生成论文摘要