Multi -Agent Deep Reinforcement Learning based Power Control for Large Energy Harvesting Networks
2019 International Symposium on Modeling and Optimization in Mobile, Ad Hoc, and Wireless Networks (WiOPT)(2019)
摘要
The goal in this work is to design online power control policies for large energy harvesting (EH) networks where, due to large energy overhead involved in the exchange of state information among the nodes, it is infeasible to use a centralized policy. Furthermore, typical applications of EH networks concern the scenario where the statistical information, about both the EH process and the wireless channel, is not available. In order to address these challenges, we propose a mean-field multiagent deep reinforcement learning framework. The proposed approach enables the nodes to learn online power control policies in a fully distributed fashion, i.e., it does not require the nodes to exchange the information about their states. Using the underlying structure of the problem, we analytically establish the convergence of the proposed scheme. In particular, we show that the policies obtained using the proposed approach converge to the ‘stationary’ Nash equilibrium. Our simulation results illustrate the efficacy of the power control policies, learned through the proposed approach. In particular, the mean-field multi-agent reinforcement learning scheme achieves a performance close to the state-of-the-art centralized policies which operate using the information about the state of whole network.
更多查看译文
AI 理解论文
溯源树
样例
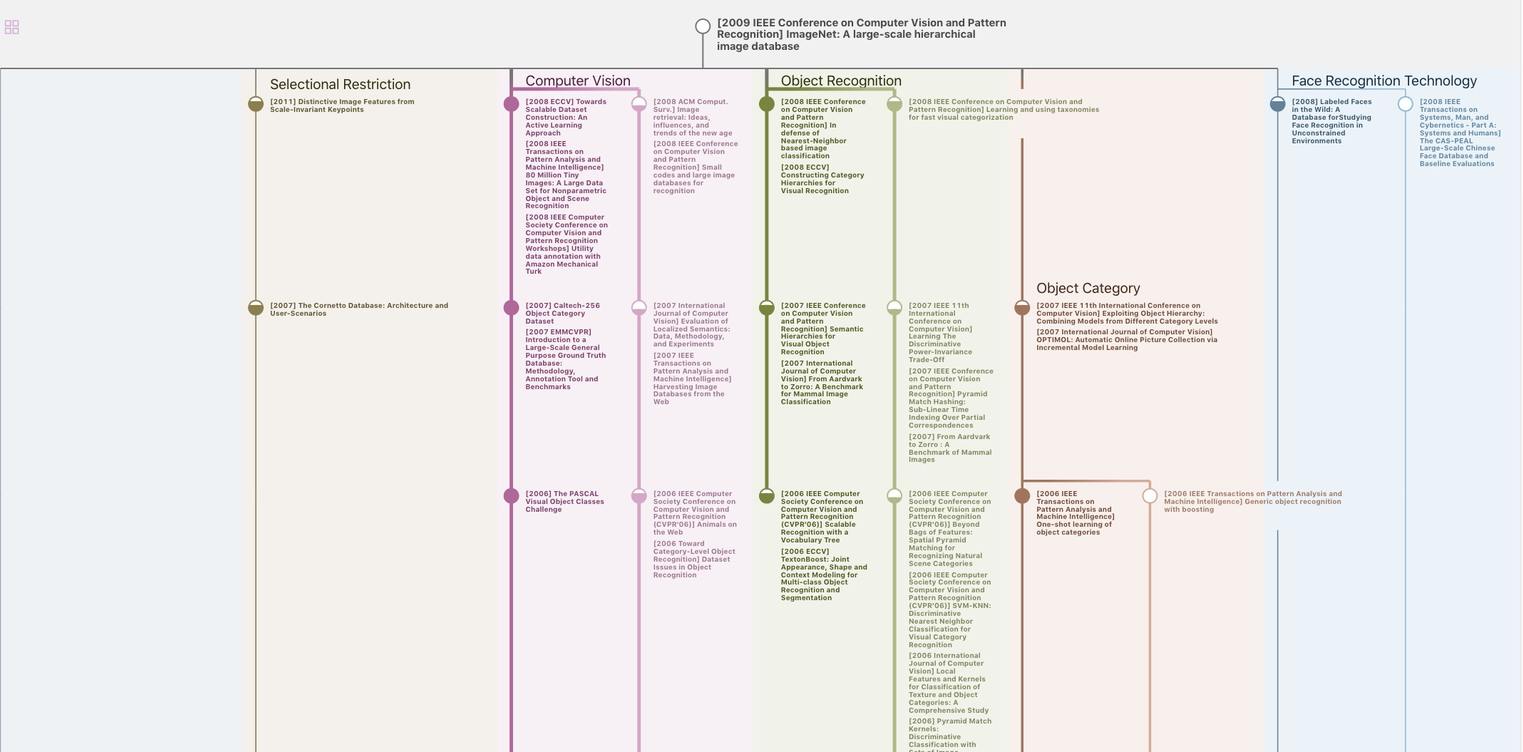
生成溯源树,研究论文发展脉络
Chat Paper
正在生成论文摘要