Self-Loop Uncertainty: A Novel Pseudo-Label for Semi-Supervised Medical Image Segmentation
medical image computing and computer-assisted intervention(2020)
摘要
Witnessing the success of deep learning neural networks in natural image processing, an increasing number of studies have been proposed to develop deep-learning-based frameworks for medical image segmentation. However, since the pixel-wise annotation of medical images is laborious and expensive, the amount of annotated data is usually deficient to well-train a neural network. In this paper, we propose a semi-supervised approach to train neural networks with limited labeled data and a large quantity of unlabeled images for medical image segmentation. A novel pseudo-label (namely self-loop uncertainty), generated by recurrently optimizing the neural network with a self-supervised task, is adopted as the ground-truth for the unlabeled images to augment the training set and boost the segmentation accuracy. The proposed self-loop uncertainty can be seen as an approximation of the uncertainty estimation yielded by ensembling multiple models with a significant reduction of inference time. Experimental results on two publicly available datasets demonstrate the effectiveness of our semi-supervised approach.
更多查看译文
关键词
Semi-supervised learning, Pseudo-label, Jigsaw puzzles
AI 理解论文
溯源树
样例
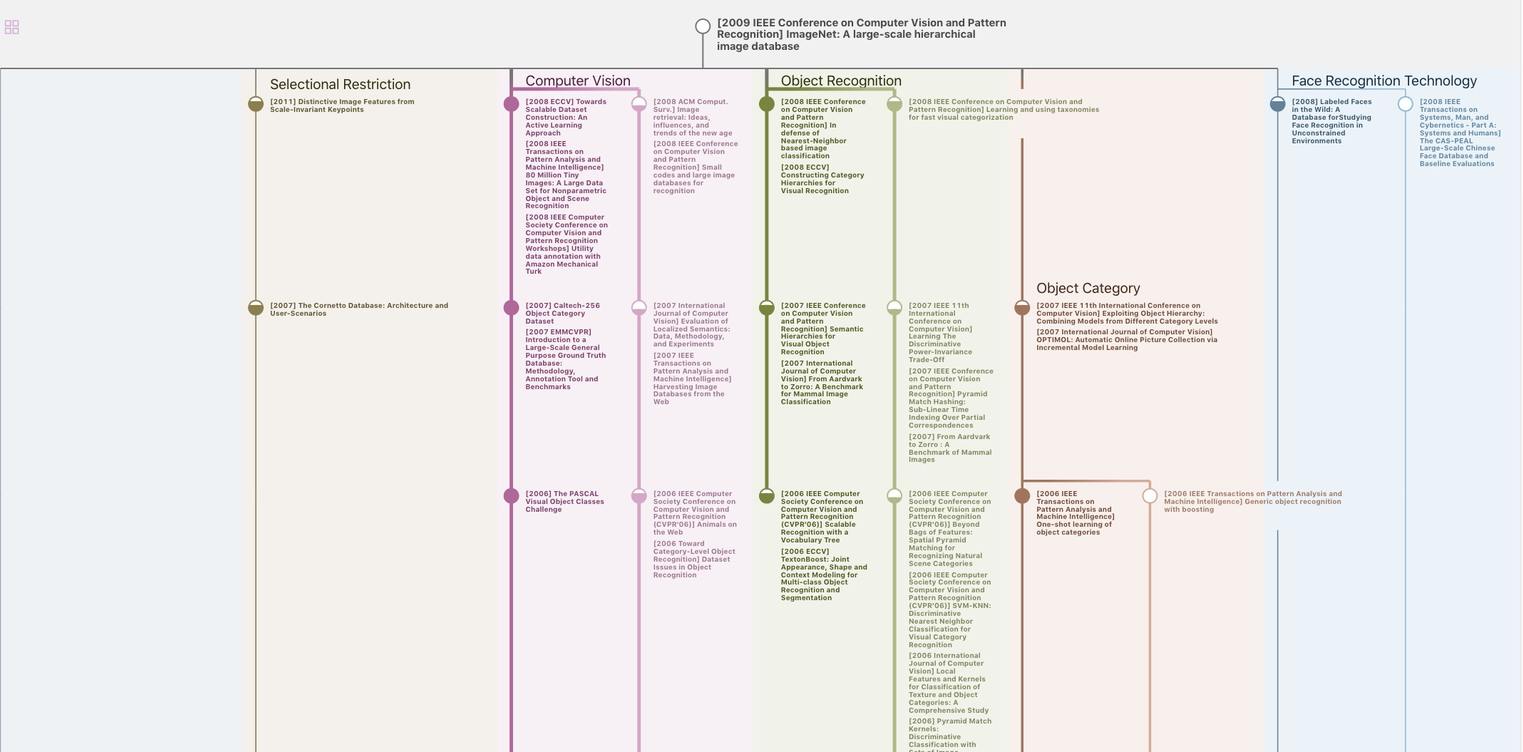
生成溯源树,研究论文发展脉络
Chat Paper
正在生成论文摘要