Segmentation Of Stem Cell Colonies In Fluorescence Microscopy Images With Transfer Learning
MEDICAL IMAGING 2020: IMAGE PROCESSING(2021)
摘要
We evaluate the use of TernausNet V2, a pre-trained VGG-16 U-net for segmentation of Green Fluorescent Protein (GFP) stained stem cells from giga pixel fluorescence microscopy images. Fluorescence microscopy is a difficult modality for automated stem cell segmentation algorithms due to high noise and low contrast. As such segmentation algorithms for cell counting and tracking typically yield more consistent results in other imaging modalities such as Phase Contrast (PC) microscopy due to greater ability to distinguish between foreground and background. Recent methods have shown that U-net based models can achieve state-of-the-art segmentation performance of GFP microscopy, although all available methods continue to overly segment the protein features and have difficulty capturing the entirety the cell. We investigate the use of TernausNet, a VGG-16 based U-Net architecture that was pre-trained from ImageNet and show that it is able to improve the accuracy of GFP stem cell segmentation on giga-scale NIST fluorescence microscopy images in comparison to a baseline U-net model. Quantitative results show that the proposed TernausNet V2 architecture model is able to better distinguish the entire region of the cell and reduce overly segmenting proteins as compared to U-net. TernausNet achieved greater accuracy with ROC AUC of 0.956 and F1-Score of 0.810 as compared to the baseline U-net with AUC 0.936 and F1-Score 0.775. Therefore, we suggest that the TernausNet V2 architecture with transfer learning improves the performance of stem-cell segmentation is able to outperform U-net models for the segmentation of giga pixel GFP stained fluorescence microscopy images.
更多查看译文
关键词
Transfer learning, microscopy, semantic segmentation, supervised learning
AI 理解论文
溯源树
样例
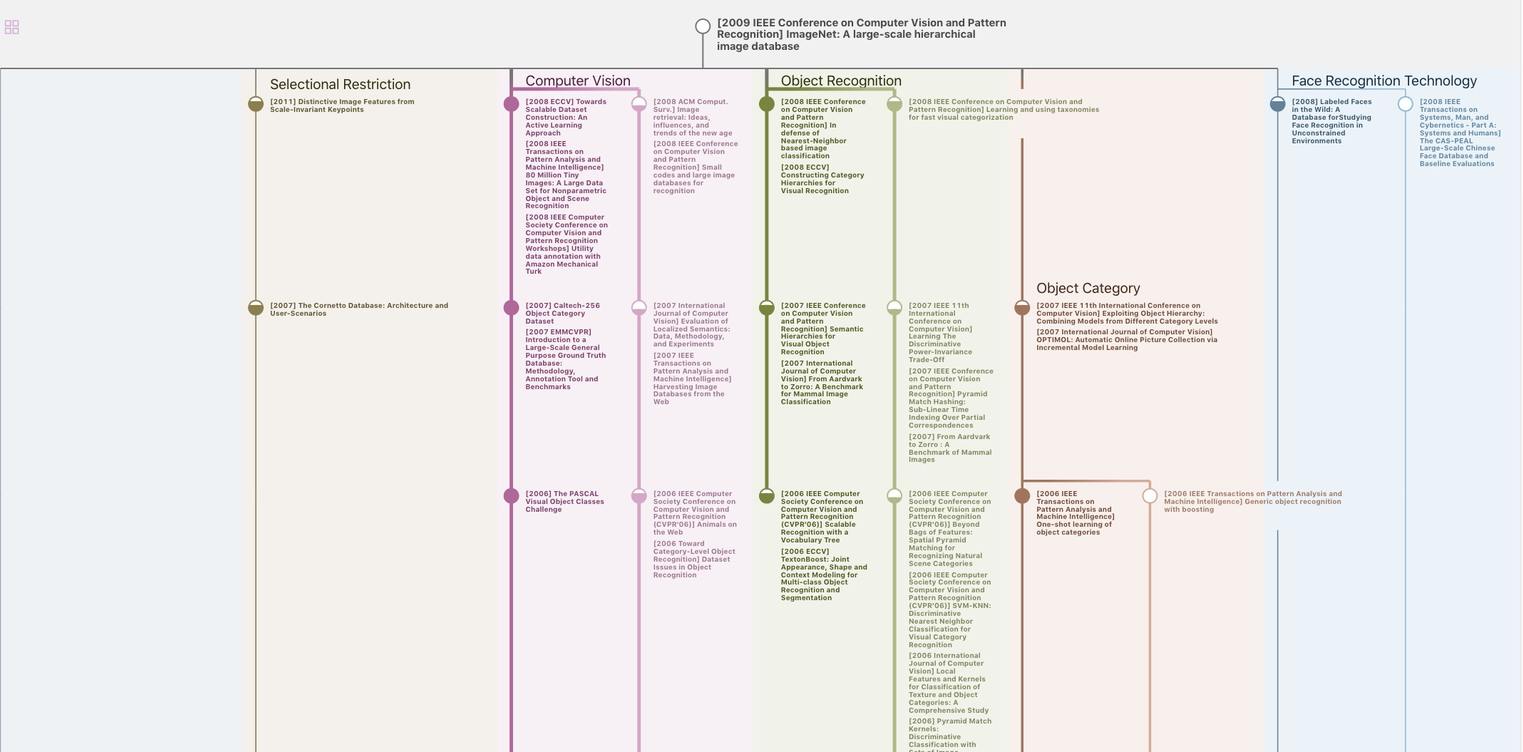
生成溯源树,研究论文发展脉络
Chat Paper
正在生成论文摘要