Predicting Clinical Diagnosis from Patients Electronic Health Records Using BERT-based Neural Networks
AIME(2020)
摘要
In this paper we study the problem of predicting clinical diagnoses from textual Electronic Health Records (EHR) data. We show the importance of this problem in medical community and present comprehensive historical review of the problem and proposed methods. As the main scientific contributions we present a modification of Bidirectional Encoder Representations from Transformers (BERT) model for sequence classification that implements a novel way of Fully-Connected (FC) layer composition and a BERT model pretrained only on domain data. To empirically validate our model, we use a large-scale Russian EHR dataset consisting of about 4 million unique patient visits. This is the largest such study for the Russian language and one of the largest globally. We performed a number of comparative experiments with other text representation models on the task of multiclass classification for 265 disease subset of ICD-10. The experiments demonstrate improved performance of our models compared to other baselines, including a fine-tuned Russian BERT (RuBERT) variant. We also show comparable performance of our model with a panel of experienced medical experts. This allows us to hope that implementation of this system will reduce misdiagnosis.
更多查看译文
关键词
patients electronic health records,clinical diagnosis,neural networks,bert-based
AI 理解论文
溯源树
样例
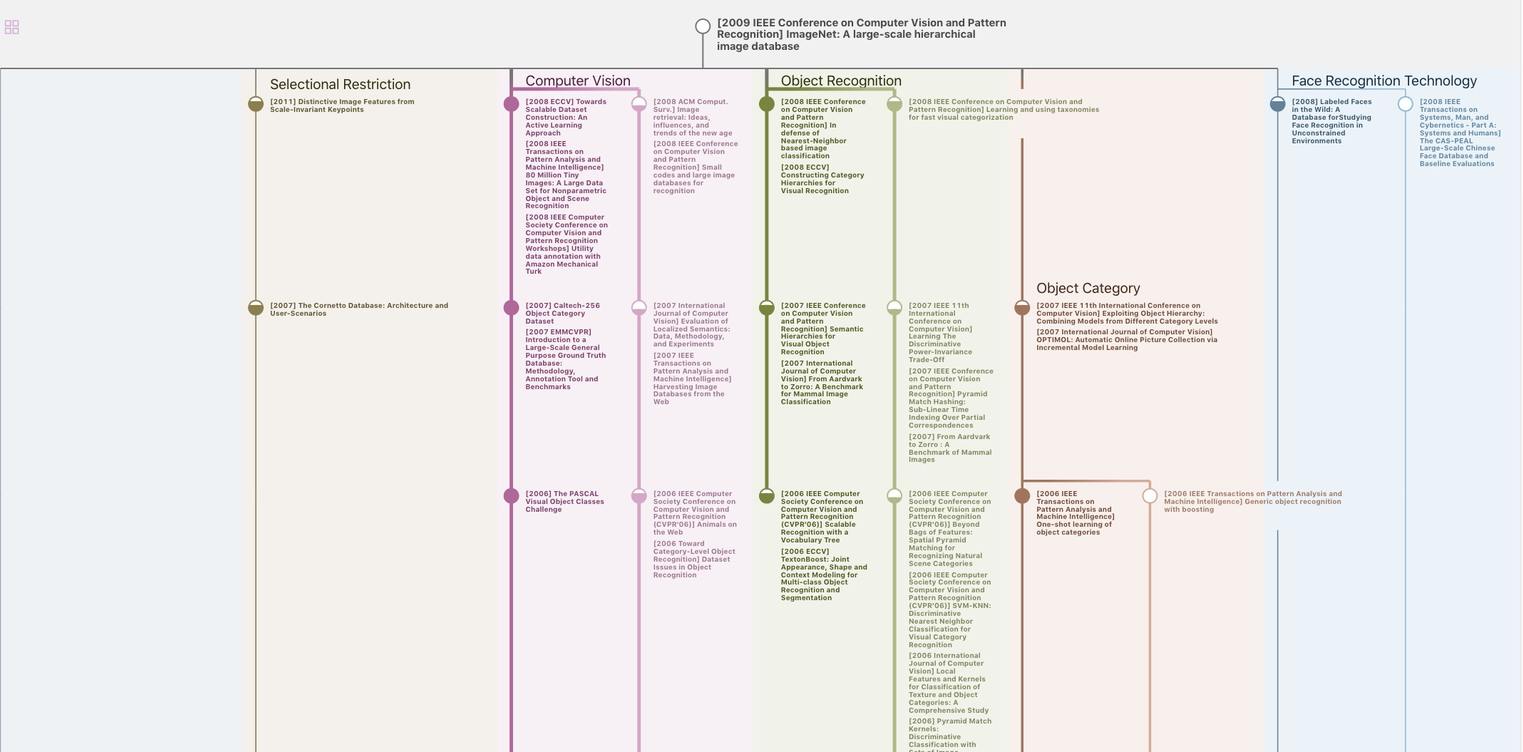
生成溯源树,研究论文发展脉络
Chat Paper
正在生成论文摘要