Efficient Online Estimation of Empowerment for Reinforcement Learning
arxiv(2020)
摘要
Training artificial agents to acquire desired skills through model-free reinforcement learning (RL) depends heavily on domain-specific knowledge, and the ability to reset the system to desirable configurations for better reward signals. The former hinders generalization to new domains; the latter precludes training in real-life conditions because physical resets are not scalable. Recently, intrinsic motivation was proposed as an alternative objective to alleviate the first issue, but there has been no reasonable remedy for the second. In this work, we present an efficient online algorithm for a type of intrinsic motivation, known as empowerment, and address both limitations. Our method is distinguished by its significantly lower sample and computation complexity, along with improved training stability compared to the relevant state of the art. We achieve this superior efficiency by transforming the challenging empowerment computation into a convex optimization problem through neural networks. In simulations, our method manages to train policies with neither domain-specific knowledge nor manual intervention. To address the issue of resetting in RL, we further show that our approach boosts learning when there's no early termination. Our proposed method opens doors for studying intrinsic motivation for policy training and scaling up model-free RL training in real-life conditions.
更多查看译文
关键词
empowerment,reinforcement learning,estimation,efficient
AI 理解论文
溯源树
样例
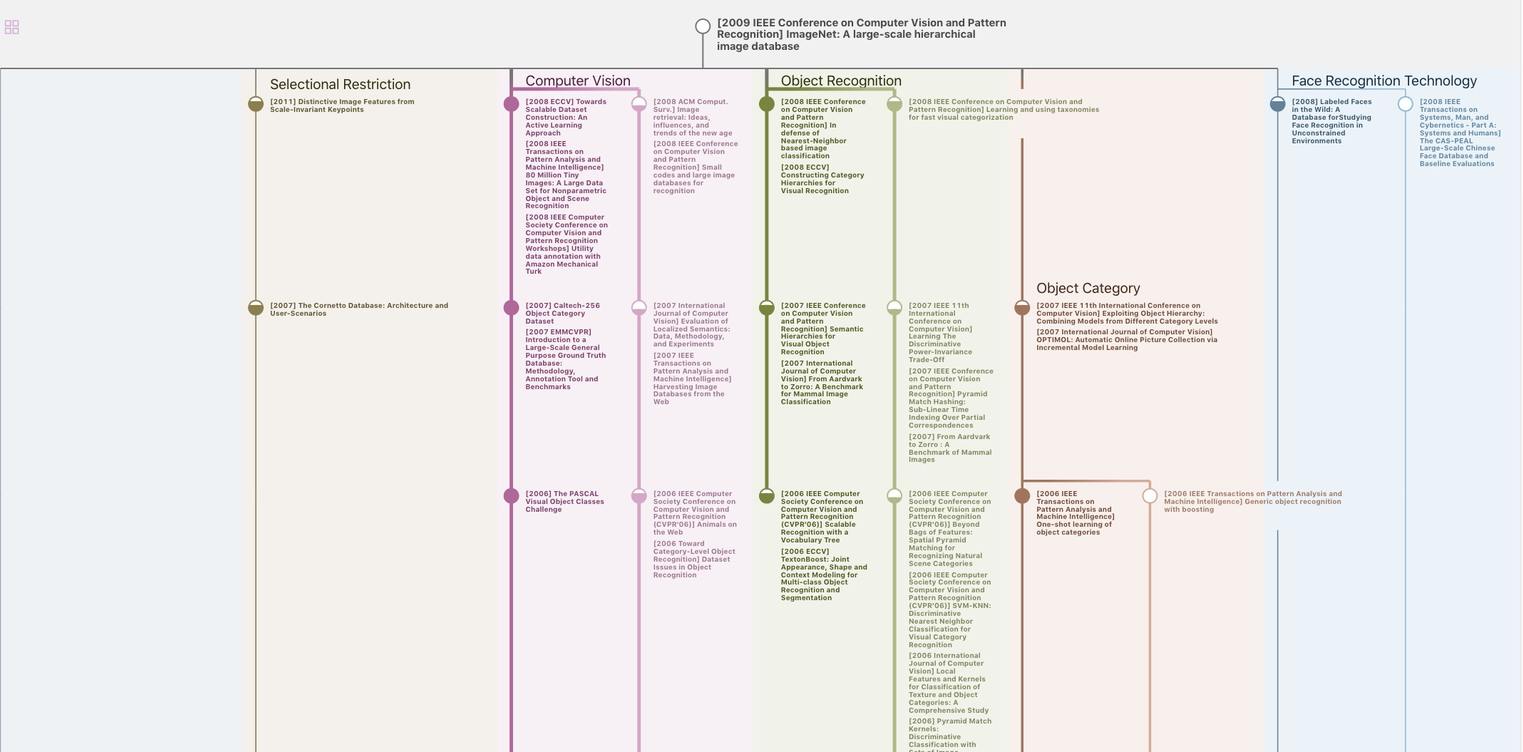
生成溯源树,研究论文发展脉络
Chat Paper
正在生成论文摘要