FedBoosting: Federated learning with gradient protected boosting for text recognition
NEUROCOMPUTING(2024)
摘要
Conventional machine learning methodologies require the centralization of data for model training, which may be infeasible in situations where data sharing limitations are imposed due to concerns such as privacy and gradient protection. The Federated Learning (FL) framework enables the collaborative learning of a shared model without necessitating the centralization or sharing of data among the data proprietors. Nonetheless, in this paper, we demonstrate that the generalization capability of the joint model is suboptimal for Non-Independent and Non-Identically Distributed (Non-IID) data, particularly when employing the Federated Averaging (FedAvg) strategy as a result of the weight divergence phenomenon. Consequently, we present a novel boosting algorithm for FL to address both the generalization and gradient leakage challenges, as well as to facilitate accelerated convergence in gradient-based optimization. Furthermore, we introduce a secure gradient sharing protocol that incorporates Homomorphic Encryption (HE) and Differential Privacy (DP) to safeguard against gradient leakage attacks. Our empirical evaluation demonstrates that the proposed Federated Boosting (FedBoosting) technique yields significant enhancements in both prediction accuracy and computational efficiency in the visual text recognition task on publicly available benchmarks.
更多查看译文
关键词
Deep learning,Federated learning,Privacy preserving
AI 理解论文
溯源树
样例
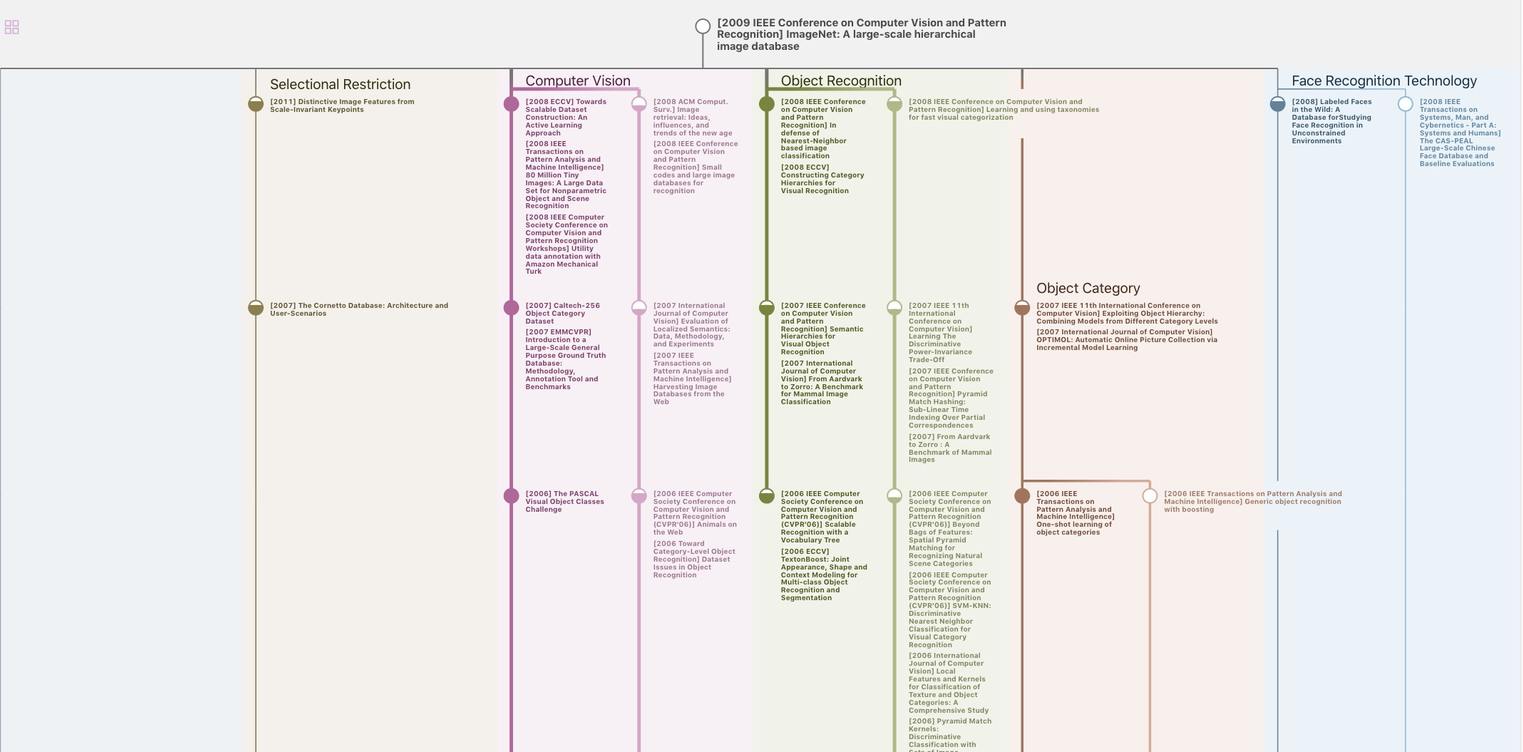
生成溯源树,研究论文发展脉络
Chat Paper
正在生成论文摘要