Simultaneous Tracking , Object Registration , and Mapping ( STORM ) by
semanticscholar(2018)
摘要
An autonomous system needs to be aware of its surroundings and know where it is in its environment in order to operate robustly in unknown environments. This problem is known as Simultaneous Localization and Mapping (SLAM). SLAM techniques have been successfully implemented on systems operating in the real world. However, most SLAM approaches assume that the environment does not change during operation — the static world assumption. When this assumption is violated (e.g. an object moves), the SLAM estimate degrades. Consequently, the static world assumption prevents robots from interacting with their environments (e.g. manipulating objects) and restricts them to navigating in static environments. Additionally, most SLAM systems generate maps composed of low-level features that lack information about objects and their locations in the scene. This representation limits the map’s utility, preventing it from being used for tasks beyond navigation such as object manipulation and task planning. We present Simultaneous Tracking, Object Registration, and Mapping (STORM), a SLAM system that represents an environment as a collection of dynamic objects. STORM enables a robot to build and maintain maps of dynamic environments, use the map estimates to manipulate objects, and localize itself in the map when revisiting the environment. We demonstrate STORM’s capabilities with simulation and real-world experiments and compare its performance against that of a typical SLAM approach. Thesis Supervisor: Gian Luca Mariottini Title: Perception and Localization Group Leader The Charles Stark Draper Laboratory Thesis Supervisor: Sertac Karaman Title: Associate Professor of Aeronautics and Astronautics
更多查看译文
AI 理解论文
溯源树
样例
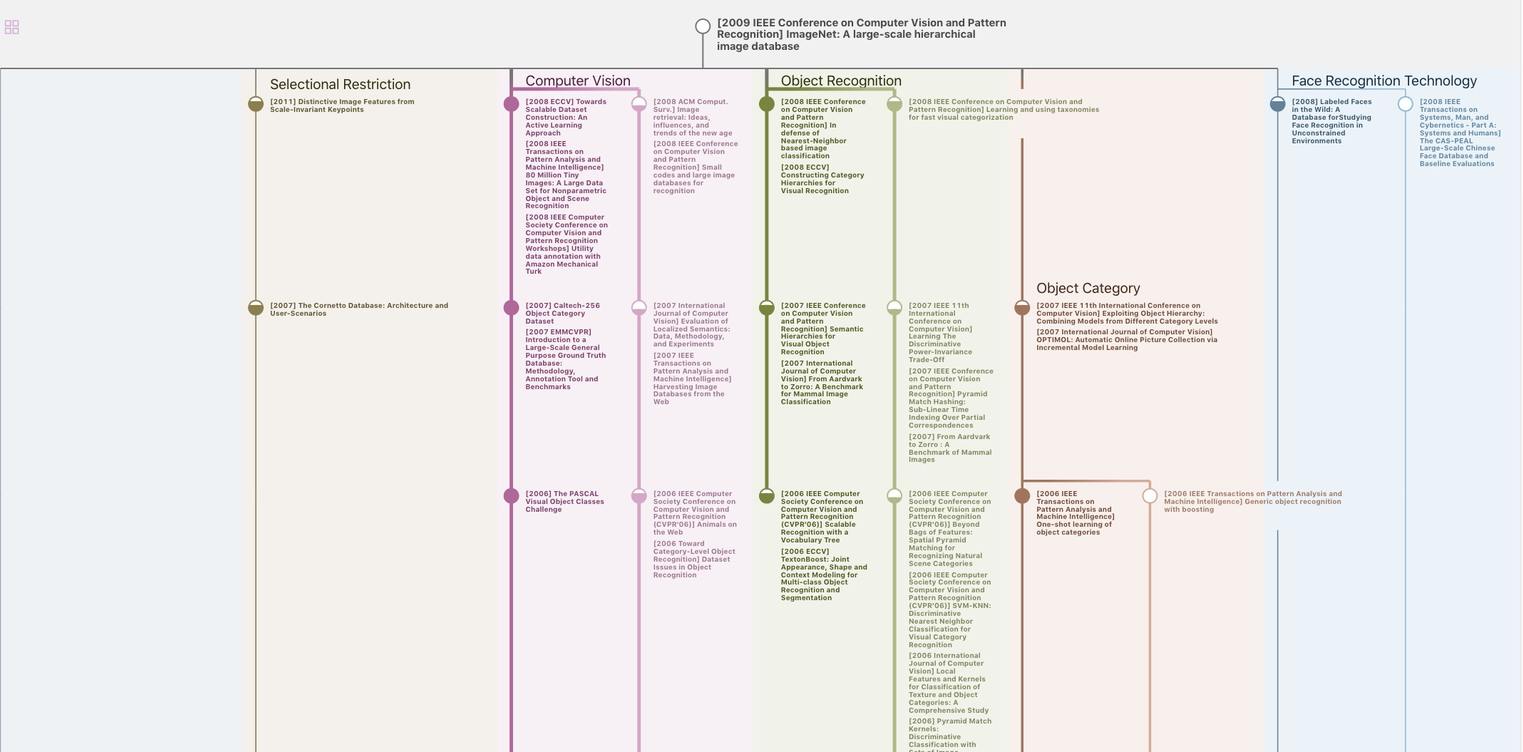
生成溯源树,研究论文发展脉络
Chat Paper
正在生成论文摘要