Support Vector Machines with Sparse Binary High-Dimensional Feature Vectors
semanticscholar(2016)
摘要
We introduce SparseMinOver, a maximum margin Perceptron training algorithm based on the MinOver algorithm that can be used for SVM training when the feature vectors are sparse, high-dimensional, and binary. Such feature vectors arise when the CRO feature map is used to map the input space to the feature space. We show that the training algorithm is efficient with this type of feature vector, while preserving the accuracy of the underlying SVM. We demonstrate the accuracy and efficiency of this technique on a number of datasets, including TIMIT, for which training a standard SVM with RBF kernel is prohibitively expensive. SparseMinOver relies on storing large indices and is particularly suited to large memory machines. External Posting Date: March 18, 2016 [Fulltext] Internal Posting Date: March 18, 2016 [Fulltext] Copyright 2016 Hewlett Packard Enterprise Development LP Support Vector Machines with Sparse Binary High-Dimensional Feature Vectors Kave Eshghi Hewlett Packard Labs 1501 Page Mill Rd. Palo Alto, CA 94304 kave.eshghi@hpe.com Mehran Kafai Hewlett Packard Labs 1501 Page Mill Rd. Palo Alto, CA 94304 mehran.kafai@hpe.com
更多查看译文
AI 理解论文
溯源树
样例
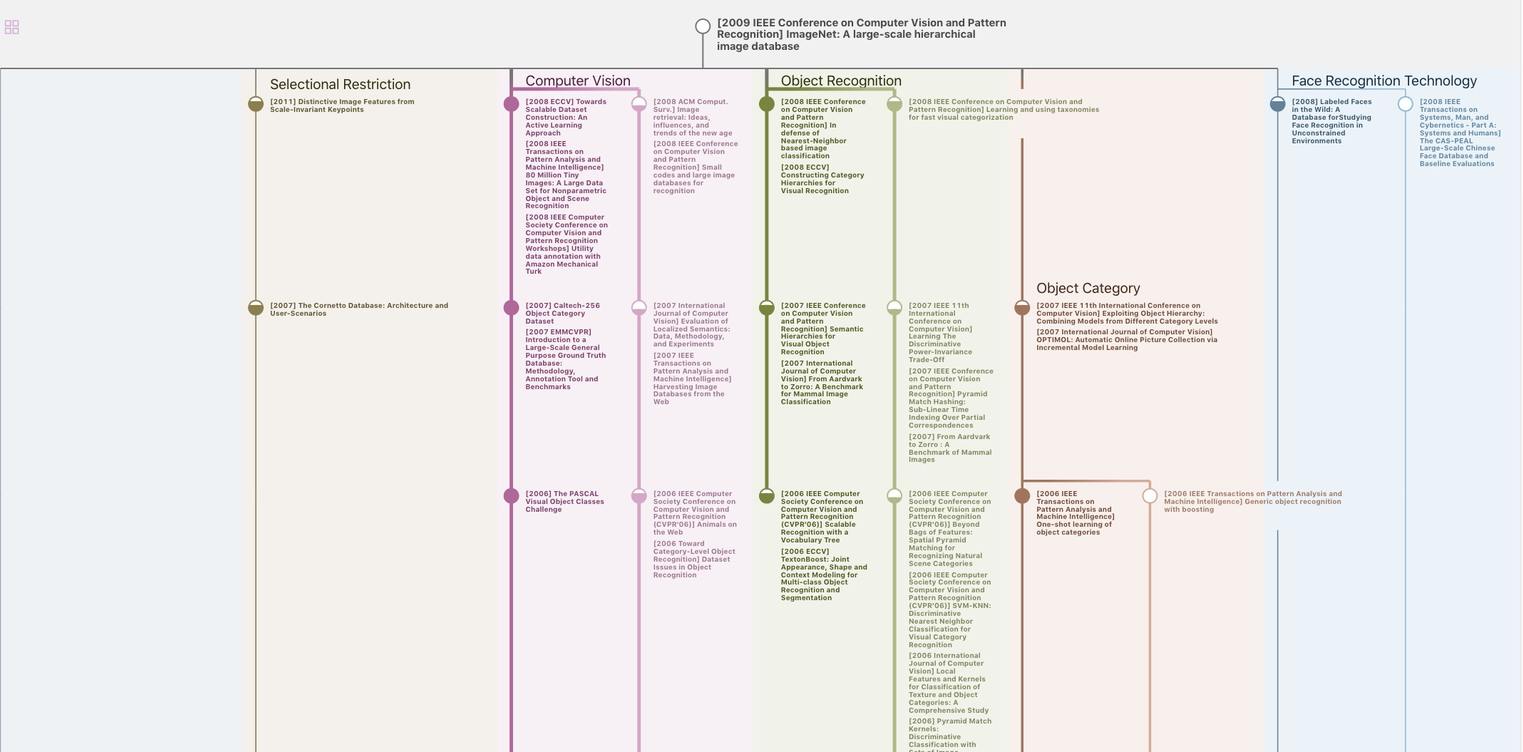
生成溯源树,研究论文发展脉络
Chat Paper
正在生成论文摘要